AUC Score :
Short-term Tactic1 :
Dominant Strategy :
Time series to forecast n:
ML Model Testing : Multi-Task Learning (ML)
Hypothesis Testing : ElasticNet Regression
Surveillance : Major exchange and OTC
1Short-term revised.
2Time series is updated based on short-term trends.
Key Points
The TR/CC CRB Wheat index is projected to experience moderate volatility. An anticipated increase in global wheat production, especially from key exporting nations, will likely exert downward pressure on prices. This may be partially offset by uncertainties surrounding weather patterns in major growing regions, potentially impacting yields and creating price support. Geopolitical tensions or unforeseen trade disruptions could lead to unexpected price spikes. Overall, the index faces a moderate risk profile, with the potential for both gains and losses depending on the interplay of supply-side factors like harvest outcomes, demand drivers linked to global consumption and trade flows, and external events capable of disrupting market equilibrium.About TR/CC CRB Wheat Index
The Thomson Reuters/CoreCommodity CRB (TR/CC CRB) Wheat Index is a benchmark designed to track the price movements of wheat futures contracts. It serves as a key indicator of the overall performance of the wheat market, reflecting the collective sentiment and expectations of traders regarding supply, demand, and other influencing factors. The index is weighted based on the trading volume and open interest of the underlying wheat futures contracts, typically traded on major exchanges such as the Chicago Board of Trade (CBOT). This methodology ensures that the index accurately represents the most liquid and actively traded segments of the wheat market.
The TR/CC CRB Wheat Index is widely used by investors, analysts, and agricultural professionals for various purposes, including market analysis, risk management, and portfolio diversification. Its performance provides insights into the agricultural sector, global food prices, and broader economic trends. The index is often utilized as a reference point for tracking the impact of geopolitical events, weather conditions, and government policies on the wheat market. It's an important tool for making informed decisions related to wheat-based commodities.
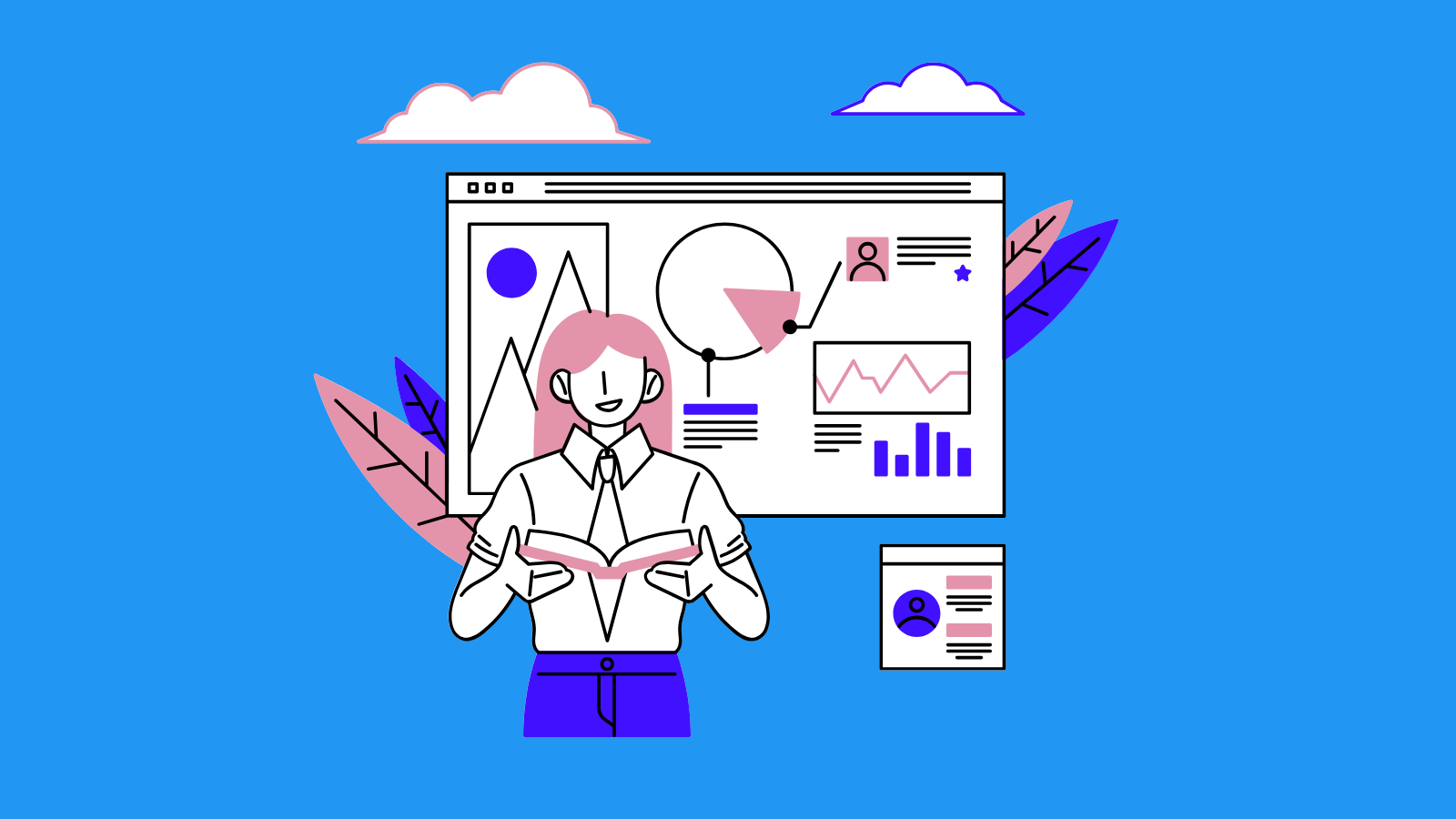
TR/CC CRB Wheat Index Forecasting Model
Our multidisciplinary team of data scientists and economists has developed a machine learning model for forecasting the TR/CC CRB Wheat index. This model leverages a comprehensive dataset encompassing various macroeconomic and agricultural factors. We have incorporated historical time series data for the Wheat index itself, alongside key economic indicators such as inflation rates (e.g., Consumer Price Index), interest rates (e.g., Federal Funds Rate), and Gross Domestic Product (GDP) growth. Furthermore, our model integrates agricultural-specific variables, including weather patterns (temperature, rainfall), crop yields, global wheat production, and inventory levels. We also account for geopolitical events that can significantly influence commodity markets, considering trade agreements, political instability, and major policy decisions by key wheat-producing and consuming nations. The model's architecture will incorporate a blend of techniques.
The model utilizes a hybrid approach combining the strengths of time series analysis and machine learning algorithms. We plan to use a combination of Autoregressive Integrated Moving Average (ARIMA) models and recurrent neural networks (RNNs), such as Long Short-Term Memory (LSTM) networks. ARIMA models will capture the autocorrelation and trend components within the historical wheat index data. LSTM networks will be employed to learn non-linear relationships and patterns among the diverse set of macroeconomic, agricultural, and geopolitical features. We'll integrate these models with a custom built ensemble, which is made by stacking the result from ARIMA model and LSTM models, and weighted according to their previous predictive performance. We will evaluate the model's performance using standard metrics, including Mean Absolute Error (MAE), Root Mean Squared Error (RMSE), and R-squared, and use these metrics to select the best model parameters through cross-validation.
The ultimate goal of this model is to provide accurate and timely forecasts of the TR/CC CRB Wheat index. This will enable market participants, including farmers, traders, and policymakers, to make informed decisions. The model will be trained and validated on historical data, and then continuously monitored and updated with new data to maintain its predictive accuracy. We will conduct rigorous sensitivity analyses to understand how each input variable affects the model's output, allowing us to identify the most significant drivers of wheat price fluctuations. Furthermore, we will develop a visualization dashboard for displaying forecast results, uncertainty bounds, and key influencing factors to facilitate understanding and decision-making. Our final deliverable will include model documentation, user manuals, and a detailed explanation of the forecasting methodology and data sources.
ML Model Testing
n:Time series to forecast
p:Price signals of TR/CC CRB Wheat index
j:Nash equilibria (Neural Network)
k:Dominated move of TR/CC CRB Wheat index holders
a:Best response for TR/CC CRB Wheat target price
For further technical information as per how our model work we invite you to visit the article below:
How do KappaSignal algorithms actually work?
TR/CC CRB Wheat Index Forecast Strategic Interaction Table
Strategic Interaction Table Legend:
X axis: *Likelihood% (The higher the percentage value, the more likely the event will occur.)
Y axis: *Potential Impact% (The higher the percentage value, the more likely the price will deviate.)
Z axis (Grey to Black): *Technical Analysis%
TR/CC CRB Wheat Index: Financial Outlook and Forecast
The TR/CC CRB Wheat Index, a benchmark reflecting the price movements of wheat futures contracts, is currently navigating a complex landscape shaped by a confluence of factors. Global wheat production levels are a primary driver. Harvest yields in key exporting countries like Russia, Ukraine, the United States, and the European Union will significantly influence the index. Weather patterns, including droughts, excessive rainfall, or extreme temperatures, can dramatically impact crop yields and subsequently, the supply available in the market. Geopolitical instability, particularly in the Black Sea region, presents a considerable risk. Any disruptions to Ukrainian wheat exports, a major global supplier, can put upward pressure on the index. Furthermore, government policies, such as export restrictions or trade agreements, can create volatility by altering the flow of wheat across international borders. The demand side is also an important factor. Global population growth, dietary preferences, and the use of wheat for animal feed all play a role in shaping demand. These factors collectively create a dynamic environment for the wheat index, making its movement highly sensitive to supply and demand imbalances.
The factors mentioned above need to be seen in the context of the current supply-demand situation. The level of global wheat stocks, both in exporting and importing countries, is crucial. If stocks are high, they provide a buffer against potential supply shocks and can limit the upside potential of the index. Conversely, low stock levels heighten the risk of price increases. Demand-side considerations also matter. The evolving global economy and its health influence overall food consumption patterns, which can impact wheat prices. The value of the US dollar, in which wheat futures contracts are often priced, influences the cost of wheat for international buyers. A strong dollar can make wheat more expensive for other nations and vice-versa. Technological advancements, such as the development of higher-yielding wheat varieties, can also affect long-term price trends. The balance of these forces, influenced by weather conditions and geopolitical events, largely determine the direction of the TR/CC CRB Wheat Index.
Looking ahead, several aspects should be carefully considered. The ongoing conflict in Ukraine is especially significant. Any further disruptions to the country's wheat exports could have a substantial impact on global prices. Weather forecasts for key wheat-producing regions will be closely monitored. Drought conditions in parts of North America or Europe, for example, could significantly raise the index. Demand-side factors, such as the pace of global economic growth and consumer spending habits, will also be crucial. Moreover, the actions of major wheat-exporting nations and their trade policies can create additional volatility. Monitoring the actions of major institutional investors and their positions in the wheat futures market can provide further insights into market sentiment and potential price movements. These interconnected elements create a complex market dynamic that necessitates a comprehensive understanding of current and prospective influences.
The financial outlook for the TR/CC CRB Wheat Index is cautiously optimistic. While the potential for volatility remains, the supply constraints due to geopolitical instability and the risk of adverse weather conditions could lead to sustained price support. The global population is also a major driver for increase in demand. However, several risks could undermine this positive outlook. A sudden resolution to the conflict in Ukraine resulting in normalized exports could cause a sharp decline in prices. A significant increase in wheat production from major exporters, fueled by favorable weather conditions, could similarly trigger a price downturn. Furthermore, a global economic recession could negatively impact demand, thereby reducing prices. Investors in this index should monitor international political conditions, weather reports in critical wheat-growing areas, and global economic indicators with vigilance. The Index has the potential to offer gains, but it's essential to be aware of the various risks.
Rating | Short-Term | Long-Term Senior |
---|---|---|
Outlook | Ba3 | Ba3 |
Income Statement | Baa2 | Baa2 |
Balance Sheet | B2 | Ba3 |
Leverage Ratios | Baa2 | Caa2 |
Cash Flow | Caa2 | Ba1 |
Rates of Return and Profitability | Ba2 | B2 |
*An aggregate rating for an index summarizes the overall sentiment towards the companies it includes. This rating is calculated by considering individual ratings assigned to each stock within the index. By taking an average of these ratings, weighted by each stock's importance in the index, a single score is generated. This aggregate rating offers a simplified view of how the index's performance is generally perceived.
How does neural network examine financial reports and understand financial state of the company?
References
- L. Prashanth and M. Ghavamzadeh. Actor-critic algorithms for risk-sensitive MDPs. In Proceedings of Advances in Neural Information Processing Systems 26, pages 252–260, 2013.
- M. Colby, T. Duchow-Pressley, J. J. Chung, and K. Tumer. Local approximation of difference evaluation functions. In Proceedings of the Fifteenth International Joint Conference on Autonomous Agents and Multiagent Systems, Singapore, May 2016
- Bottou L. 1998. Online learning and stochastic approximations. In On-Line Learning in Neural Networks, ed. D Saad, pp. 9–42. New York: ACM
- H. Kushner and G. Yin. Stochastic approximation algorithms and applications. Springer, 1997.
- Tibshirani R. 1996. Regression shrinkage and selection via the lasso. J. R. Stat. Soc. B 58:267–88
- A. Shapiro, W. Tekaya, J. da Costa, and M. Soares. Risk neutral and risk averse stochastic dual dynamic programming method. European journal of operational research, 224(2):375–391, 2013
- Bottou L. 1998. Online learning and stochastic approximations. In On-Line Learning in Neural Networks, ed. D Saad, pp. 9–42. New York: ACM