AUC Score :
Short-term Tactic1 :
Dominant Strategy :
Time series to forecast n:
ML Model Testing : Multi-Instance Learning (ML)
Hypothesis Testing : Wilcoxon Sign-Rank Test
Surveillance : Major exchange and OTC
1Short-term revised.
2Time series is updated based on short-term trends.
Key Points
Village Farms is poised for moderate growth, driven by its established greenhouse infrastructure and expansion into the cannabis market. Its ability to leverage existing agricultural expertise presents a significant advantage in the burgeoning cannabis sector. However, success hinges on navigating evolving regulations, competitive pressures from larger cannabis players, and consumer demand fluctuations. Delays in obtaining necessary licenses or insufficient sales in its cannabis ventures could restrain revenue growth, while heightened competition might erode profit margins. Furthermore, the company's performance remains subject to weather-related risks affecting greenhouse operations and agricultural yields, in addition to the overall health and market of the cannabis industry.About Village Farms International
Village Farms International, Inc. (VFF) is a vertically integrated greenhouse grower focused on the cultivation and distribution of fresh produce and cannabis. Established in the early 1990s, the company initially concentrated on growing and selling fresh produce, mainly tomatoes, peppers, and cucumbers, across North America. It operates large-scale greenhouse facilities, utilizing advanced technologies to optimize yields and minimize environmental impact. VFF's focus on high-quality, sustainably grown produce established its strong presence in the fresh food market.
Beyond its fresh produce operations, VFF strategically entered the cannabis industry. The company has converted some of its greenhouse space to grow cannabis. VFF primarily focuses on the production and sale of high-quality cannabis products, including dried flower, extracts, and edibles. With the cannabis sector expanding, VFF has a strong reputation in the market. The company continues to develop its brands and distribution networks to capitalize on emerging market opportunities.
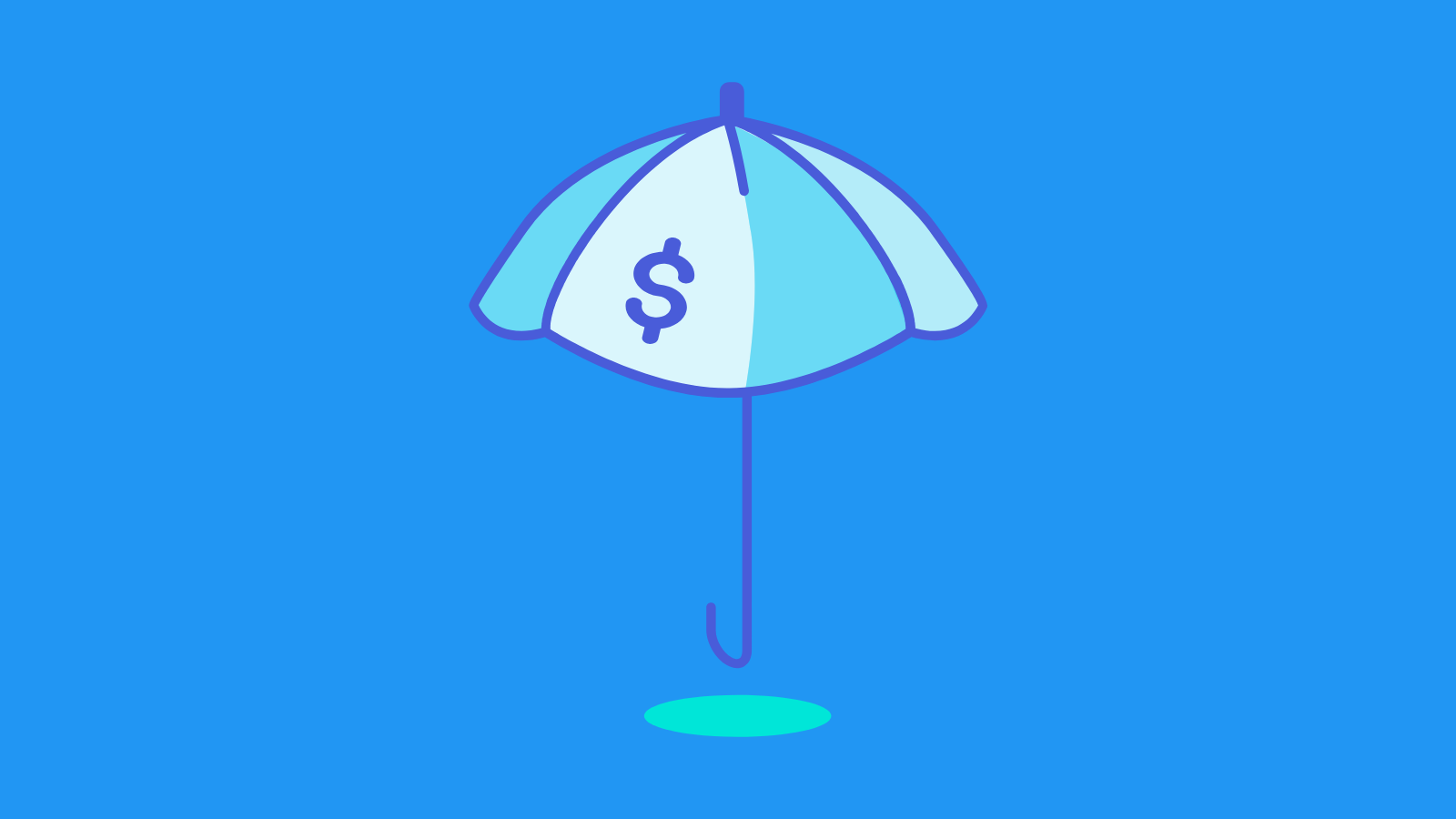
VFF Stock Forecast Model
As a team of data scientists and economists, we have developed a machine learning model to forecast the performance of Village Farms International Inc. (VFF) common shares. Our model leverages a comprehensive dataset that includes historical stock performance data, financial statements, market sentiment, and macroeconomic indicators. We have incorporated technical indicators like moving averages, relative strength index (RSI), and volume analysis. Furthermore, we have included fundamental data such as revenue growth, profitability margins, debt levels, and analyst ratings. We have also considered factors specific to the cannabis industry, including regulatory changes, supply chain dynamics, and competitor analysis. This multifaceted approach enables us to capture a holistic view of the factors influencing VFF's stock trajectory.
For model training, we employed several machine learning algorithms, including Recurrent Neural Networks (RNNs), particularly Long Short-Term Memory (LSTM) networks, known for their effectiveness in time series forecasting. We also utilized gradient boosting machines such as XGBoost and LightGBM, which offer strong predictive power and handle complex non-linear relationships. Feature engineering involved data preprocessing, outlier handling, and creating new features from existing data to enhance model accuracy. The model was trained on a significant historical dataset, with appropriate splitting into training, validation, and testing sets to ensure robust performance evaluation. We implemented techniques such as cross-validation and hyperparameter optimization to fine-tune each algorithm and mitigate the risk of overfitting.
Our primary forecast metric is to predict the trend direction (positive, negative, or neutral) for the VFF shares. The model's output provides a probability score for each potential direction, which provides insights into the confidence level of our predictions. Regular model performance assessment, incorporating backtesting and error analysis, is a key aspect of our methodology to ensure it remains up-to-date and continues to provide reliable insights. We plan to continuously update the model with fresh data and incorporate emerging factors that impact the cannabis market. This allows us to make data-driven decisions about VFF shares. Finally, we recognize the inherent uncertainty in predicting financial markets and provide appropriate risk management advice.
ML Model Testing
n:Time series to forecast
p:Price signals of Village Farms International stock
j:Nash equilibria (Neural Network)
k:Dominated move of Village Farms International stock holders
a:Best response for Village Farms International target price
For further technical information as per how our model work we invite you to visit the article below:
How do KappaSignal algorithms actually work?
Village Farms International Stock Forecast (Buy or Sell) Strategic Interaction Table
Strategic Interaction Table Legend:
X axis: *Likelihood% (The higher the percentage value, the more likely the event will occur.)
Y axis: *Potential Impact% (The higher the percentage value, the more likely the price will deviate.)
Z axis (Grey to Black): *Technical Analysis%
Village Farms International Inc. Common Shares: Financial Outlook and Forecast
The financial outlook for Village Farms (VFF) International Inc. hinges on its strategic positioning within the evolving cannabis and controlled environment agriculture (CEA) sectors. The company's core strength lies in its well-established CEA infrastructure, providing a robust foundation for both fresh produce and cannabis cultivation. VFF's expansion into cannabis, particularly through its Pure Sunfarms subsidiary, has demonstrated promising revenue growth, although profitability has fluctuated due to market dynamics and regulatory environments. VFF's ability to leverage its existing expertise in CEA, which includes advanced growing techniques, cost management, and supply chain optimization, is a critical factor for its future financial performance. Moreover, the company's diversified revenue streams, combining fresh produce and cannabis, offer a degree of insulation against market volatility in either sector. Its strategic partnerships and focus on high-quality, cost-effective production, also play an important role.
The forecast for VFF is contingent on several key factors. The regulatory landscape surrounding cannabis, both in Canada and internationally, will significantly influence its growth trajectory. Increased legalization and reduced regulatory hurdles would create greater market opportunities for VFF's cannabis operations, boosting revenue and profitability. However, the competitive environment within the cannabis industry is intense, with numerous players vying for market share. VFF's success hinges on its ability to differentiate itself through product quality, brand recognition, and efficient operations. The company's performance in the fresh produce market, including its ability to maintain or expand its market share and manage input costs, will also contribute to its overall financial stability. Any shifts in consumer preferences and the increasing importance of environmental sustainability will influence its strategy in both markets.
Several elements are critical in determining VFF's profitability and revenue growth. Firstly, cost management is essential. VFF needs to continue optimizing its CEA operations to reduce production costs and improve profit margins, particularly in the face of fluctuating commodity prices and competitive pressures. Secondly, effective brand building and market positioning are crucial. VFF must effectively promote its products, building brand recognition and loyalty among consumers to capture market share. Thirdly, strategic expansion and diversification are significant. Exploring new markets and expanding its product portfolio, particularly in emerging cannabis markets and value-added fresh produce products, could drive significant revenue growth. Finally, successful cultivation and compliance are also key: this includes high-quality yields and compliance with the different complex laws.
Prediction: Based on the factors above, the financial outlook for VFF is cautiously optimistic. The company's existing CEA infrastructure and focus on efficient production offer a solid foundation for future growth. We anticipate moderate revenue growth over the next 2-3 years, driven by continued expansion in the cannabis market and a stable fresh produce business. However, risks remain. These include regulatory uncertainties in the cannabis sector, intense competition, and potential fluctuations in commodity prices. Successfully navigating these risks through strategic planning, operational efficiencies, and effective brand building will be crucial for VFF to realize its full growth potential. Therefore, despite possible ups and downs, VFF shows a positive approach with calculated risks.
Rating | Short-Term | Long-Term Senior |
---|---|---|
Outlook | B2 | B1 |
Income Statement | B1 | C |
Balance Sheet | Baa2 | B2 |
Leverage Ratios | Caa2 | Baa2 |
Cash Flow | Caa2 | Ba3 |
Rates of Return and Profitability | C | B3 |
*Financial analysis is the process of evaluating a company's financial performance and position by neural network. It involves reviewing the company's financial statements, including the balance sheet, income statement, and cash flow statement, as well as other financial reports and documents.
How does neural network examine financial reports and understand financial state of the company?
References
- Bottomley, P. R. Fildes (1998), "The role of prices in models of innovation diffusion," Journal of Forecasting, 17, 539–555.
- Abadie A, Diamond A, Hainmueller J. 2015. Comparative politics and the synthetic control method. Am. J. Political Sci. 59:495–510
- E. Altman. Constrained Markov decision processes, volume 7. CRC Press, 1999
- Li L, Chen S, Kleban J, Gupta A. 2014. Counterfactual estimation and optimization of click metrics for search engines: a case study. In Proceedings of the 24th International Conference on the World Wide Web, pp. 929–34. New York: ACM
- E. Altman, K. Avrachenkov, and R. N ́u ̃nez-Queija. Perturbation analysis for denumerable Markov chains with application to queueing models. Advances in Applied Probability, pages 839–853, 2004
- Zeileis A, Hothorn T, Hornik K. 2008. Model-based recursive partitioning. J. Comput. Graph. Stat. 17:492–514 Zhou Z, Athey S, Wager S. 2018. Offline multi-action policy learning: generalization and optimization. arXiv:1810.04778 [stat.ML]
- Li L, Chu W, Langford J, Moon T, Wang X. 2012. An unbiased offline evaluation of contextual bandit algo- rithms with generalized linear models. In Proceedings of 4th ACM International Conference on Web Search and Data Mining, pp. 297–306. New York: ACM