AUC Score :
Short-term Tactic1 :
Dominant Strategy :
Time series to forecast n:
ML Model Testing : Statistical Inference (ML)
Hypothesis Testing : Stepwise Regression
Surveillance : Major exchange and OTC
1Short-term revised.
2Time series is updated based on short-term trends.
Key Points
TFI's future performance is anticipated to be positive, driven by continued expansion through strategic acquisitions and the integration of acquired businesses, likely leading to increased revenue and market share. However, risks remain, including challenges in effectively integrating new acquisitions, which could lead to operational inefficiencies and financial strain. Additionally, the company faces exposure to fluctuations in fuel prices and potential economic downturns, impacting profitability and demand for its services. Furthermore, intense competition in the transportation industry could squeeze margins and limit growth potential.About TFI International
TFI International Inc. (TFI) is a Canadian-based transportation and logistics company providing a wide range of services across North America. It operates through several business segments, including package and courier, less-than-truckload (LTL), truckload, and logistics. These segments collectively offer solutions for the movement of freight, ranging from small packages to large shipments, and encompassing various transportation modes such as ground, air, and sea. TFI's extensive network and diverse service offerings cater to a broad customer base, including businesses in retail, manufacturing, and e-commerce.
TFI has grown significantly through strategic acquisitions, expanding its geographic reach and service capabilities. The company focuses on operational efficiency and customer satisfaction. Its strategy emphasizes a disciplined approach to capital allocation and integrating acquired businesses effectively. With a strong presence in the transportation and logistics sector, TFI International continues to adapt to market demands and technological advancements, aiming to maintain its position as a leading provider of integrated supply chain solutions.
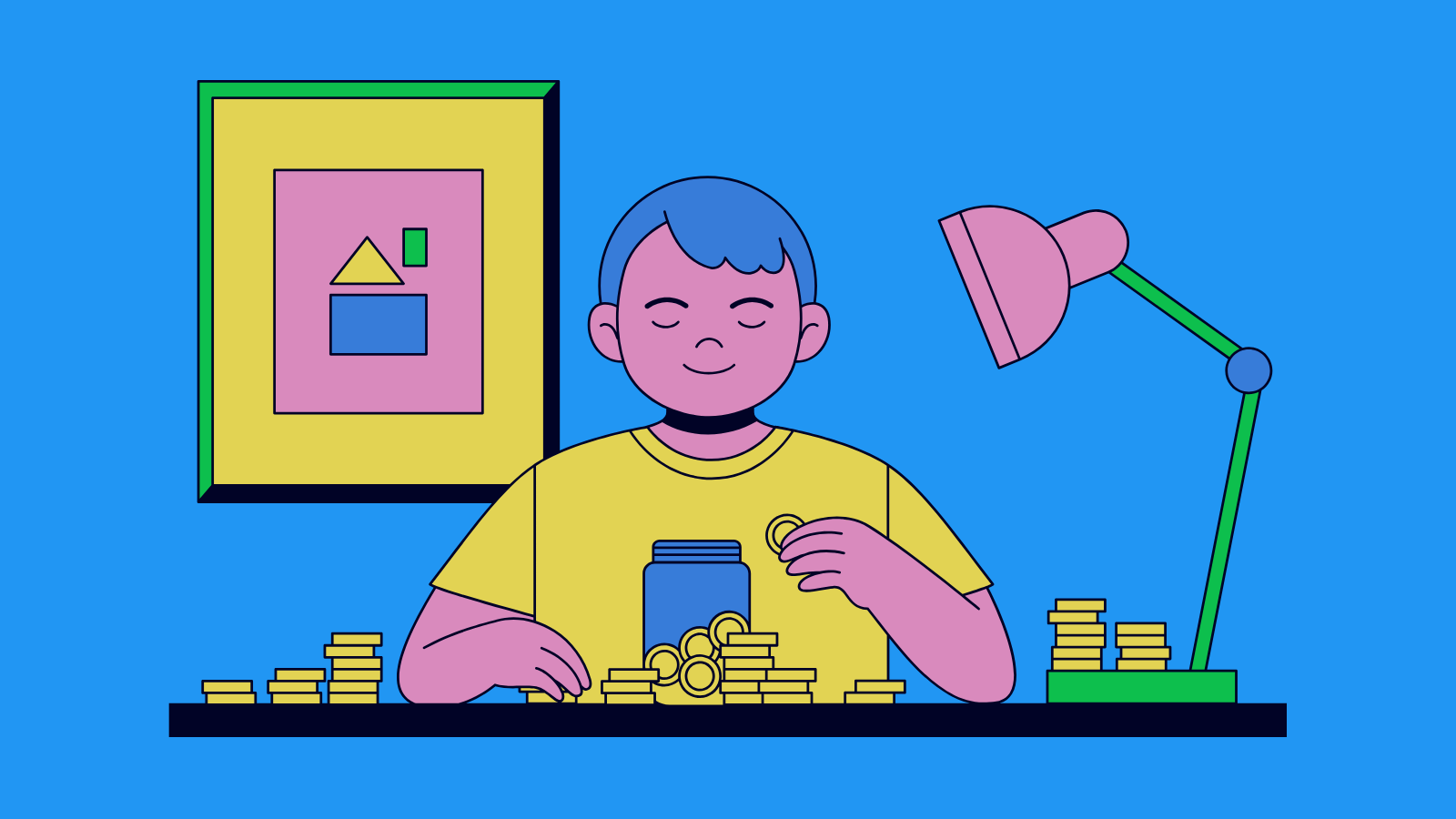
TFI International Inc. (TFII) Stock Forecast Model
Our data science and economics team has developed a machine learning model to forecast the performance of TFI International Inc. (TFII) common shares. The model leverages a diverse dataset encompassing both internal and external factors. Internal data includes TFII's financial statements (revenue, earnings, cash flow), operational metrics (tonnage, shipping volumes, logistics efficiency), and management commentary from earnings calls. We also analyze historical stock price data to identify trends, patterns, and volatility. External data integrated into the model involves macroeconomic indicators such as GDP growth, inflation rates, interest rates, and consumer spending, as these factors significantly influence the transportation and logistics industry. Furthermore, we incorporate industry-specific variables like fuel prices, transportation capacity utilization rates, and competitor performance. Finally, we consider global trade flows and geopolitical risks that could impact TFII's operations.
The modeling approach utilizes a combination of techniques to achieve robust and accurate forecasting. We employ a variety of machine learning algorithms, including Time Series Analysis, Regression Models, and Recurrent Neural Networks (RNNs). These algorithms are chosen for their ability to capture complex, non-linear relationships within the data and effectively handle time-dependent variables. We use historical data to train the model, and then validate it using a portion of unseen data to ensure its predictive accuracy. Model performance is assessed using standard evaluation metrics like Mean Squared Error (MSE), Root Mean Squared Error (RMSE), and Mean Absolute Error (MAE). We regularly update the model with fresh data, re-evaluating and re-tuning the model as needed, ensuring that it remains relevant and effective in the face of evolving market conditions. The model generates forecasts for a specific period, providing insights into the expected direction of TFII's stock behavior.
The output of the model provides a probabilistic forecast, indicating the likely range of future TFII stock performance, rather than a single point estimate. This comprehensive approach incorporates both quantitative and qualitative data, allowing us to generate more informed and reliable predictions. The model also includes a sensitivity analysis component to determine the impact of different variables. By understanding the key drivers of stock performance, investors and stakeholders can develop more informed strategies. While our model offers valuable insights, it is important to remember that stock forecasting is inherently uncertain. We consistently monitor model performance, address any limitations, and make improvements as needed. Investors must consider other factors, conduct their own due diligence, and avoid relying solely on the model's output for investment decisions.
```
ML Model Testing
n:Time series to forecast
p:Price signals of TFI International stock
j:Nash equilibria (Neural Network)
k:Dominated move of TFI International stock holders
a:Best response for TFI International target price
For further technical information as per how our model work we invite you to visit the article below:
How do KappaSignal algorithms actually work?
TFI International Stock Forecast (Buy or Sell) Strategic Interaction Table
Strategic Interaction Table Legend:
X axis: *Likelihood% (The higher the percentage value, the more likely the event will occur.)
Y axis: *Potential Impact% (The higher the percentage value, the more likely the price will deviate.)
Z axis (Grey to Black): *Technical Analysis%
TFI International Inc. Common Shares: Financial Outlook and Forecast
TFI's financial outlook appears predominantly positive, underpinned by several key factors. The company's core business, centered on freight transportation and logistics, benefits from the persistent demand for goods and services. E-commerce growth continues to drive demand for parcel delivery and last-mile solutions, areas where TFI has strategically expanded its footprint. Furthermore, TFI's diversified service portfolio, encompassing less-than-truckload (LTL), truckload, package and courier, and logistics, provides resilience against cyclical downturns in any single segment. The company's history of strategic acquisitions, aimed at expanding market share and geographical reach, is also expected to contribute to revenue growth. Moreover, TFI's focus on operational efficiency and cost management, particularly within its integrated network, positions the company favorably to maintain and expand its profit margins.
Forecasting TFI's financial performance involves considering various elements. Revenue growth is projected to be supported by ongoing e-commerce demand and acquisitions. Profit margins are anticipated to remain strong, driven by operational efficiencies and pricing strategies. The company's management team has a proven track record of effectively integrating acquisitions and improving profitability. Moreover, TFI's geographic diversification, including a significant presence in North America, helps mitigate the risks associated with economic fluctuations in any particular region. Investments in technology, such as tracking systems and automated sorting facilities, are expected to enhance operational effectiveness and support future growth. The company's commitment to returning value to shareholders, through dividends and share repurchases, also suggests a confident outlook.
Key indicators to monitor in the future encompass several aspects. The strength of consumer spending, particularly in areas reliant on parcel delivery services, will be crucial. Changes in fuel prices are a significant factor, as they can directly impact operating costs. Developments in the labor market, particularly regarding driver availability and wage rates, will have an impact. The execution of TFI's acquisition strategy will be important to observe, as integration risks and potential synergies can materially impact financial results. Technological advancements within the logistics sector, such as the adoption of autonomous vehicles and advancements in supply chain optimization, will also impact the sector's evolution. Furthermore, monitoring of trade agreements and potential shifts in global supply chains will be essential.
Overall, a positive financial outlook is predicted for TFI, contingent on consistent execution and favorable economic conditions. This outlook is supported by robust industry trends, strategic positioning, and proven management. However, risks remain. A potential economic slowdown, a sharp increase in fuel prices, or disruptions in the supply chain could negatively impact financial performance. Increased competition from larger players or shifts in consumer behavior towards different delivery methods could also pose challenges. Despite these risks, TFI's diversified business model and history of adapting to market changes suggest that the company is well-positioned to navigate uncertainties and achieve continued success.
Rating | Short-Term | Long-Term Senior |
---|---|---|
Outlook | B1 | Ba3 |
Income Statement | C | Caa2 |
Balance Sheet | B2 | Baa2 |
Leverage Ratios | Ba1 | B1 |
Cash Flow | B1 | B2 |
Rates of Return and Profitability | Baa2 | Ba1 |
*Financial analysis is the process of evaluating a company's financial performance and position by neural network. It involves reviewing the company's financial statements, including the balance sheet, income statement, and cash flow statement, as well as other financial reports and documents.
How does neural network examine financial reports and understand financial state of the company?
References
- Angrist JD, Pischke JS. 2008. Mostly Harmless Econometrics: An Empiricist's Companion. Princeton, NJ: Princeton Univ. Press
- Wooldridge JM. 2010. Econometric Analysis of Cross Section and Panel Data. Cambridge, MA: MIT Press
- Chernozhukov V, Newey W, Robins J. 2018c. Double/de-biased machine learning using regularized Riesz representers. arXiv:1802.08667 [stat.ML]
- D. S. Bernstein, S. Zilberstein, and N. Immerman. The complexity of decentralized control of Markov Decision Processes. In UAI '00: Proceedings of the 16th Conference in Uncertainty in Artificial Intelligence, Stanford University, Stanford, California, USA, June 30 - July 3, 2000, pages 32–37, 2000.
- L. Prashanth and M. Ghavamzadeh. Actor-critic algorithms for risk-sensitive MDPs. In Proceedings of Advances in Neural Information Processing Systems 26, pages 252–260, 2013.
- R. Sutton and A. Barto. Reinforcement Learning. The MIT Press, 1998
- Bennett J, Lanning S. 2007. The Netflix prize. In Proceedings of KDD Cup and Workshop 2007, p. 35. New York: ACM