AUC Score :
Short-term Tactic1 :
Dominant Strategy :
Time series to forecast n:
ML Model Testing : Reinforcement Machine Learning (ML)
Hypothesis Testing : Ridge Regression
Surveillance : Major exchange and OTC
1Short-term revised.
2Time series is updated based on short-term trends.
Key Points
SUZ will likely experience moderate growth, fueled by increased demand for pulp in Asia and ongoing efforts to improve operational efficiency. Expansion into new markets, particularly for specialty pulp products, could further bolster revenue. However, SUZ faces risks including fluctuations in pulp prices, which are sensitive to global economic conditions and supply chain disruptions. Adverse weather events, such as droughts or floods, could also negatively impact production and profitability. Currency exchange rate volatility, especially between the Brazilian Real and the US dollar, poses a significant financial risk. The company's substantial debt load and potential regulatory changes in Brazil also warrant close monitoring.About Suzano S.A.
Suzano S.A. (SUZ) is a global leader in the production of eucalyptus pulp, one of the world's largest integrated pulp and paper producers. The company is headquartered in Brazil and operates significant forestry plantations alongside its pulp mills, ensuring a sustainable supply chain. Suzano also produces a diverse range of paper products, including printing and writing paper, packaging paper, and tissue paper, serving markets worldwide. Its vertically integrated model gives it greater control over its production processes, enhancing efficiency and cost management within the industry.
The company's strategic focus emphasizes sustainability and innovation, with significant investments in research and development to improve its pulp yields and develop eco-friendly paper solutions. Suzano is deeply committed to environmental stewardship and the responsible management of its vast forest resources, aiming to minimize its environmental footprint through sustainable forestry practices. It is publicly listed and adheres to international corporate governance standards, reflecting its commitment to transparency and long-term value creation for its stakeholders.
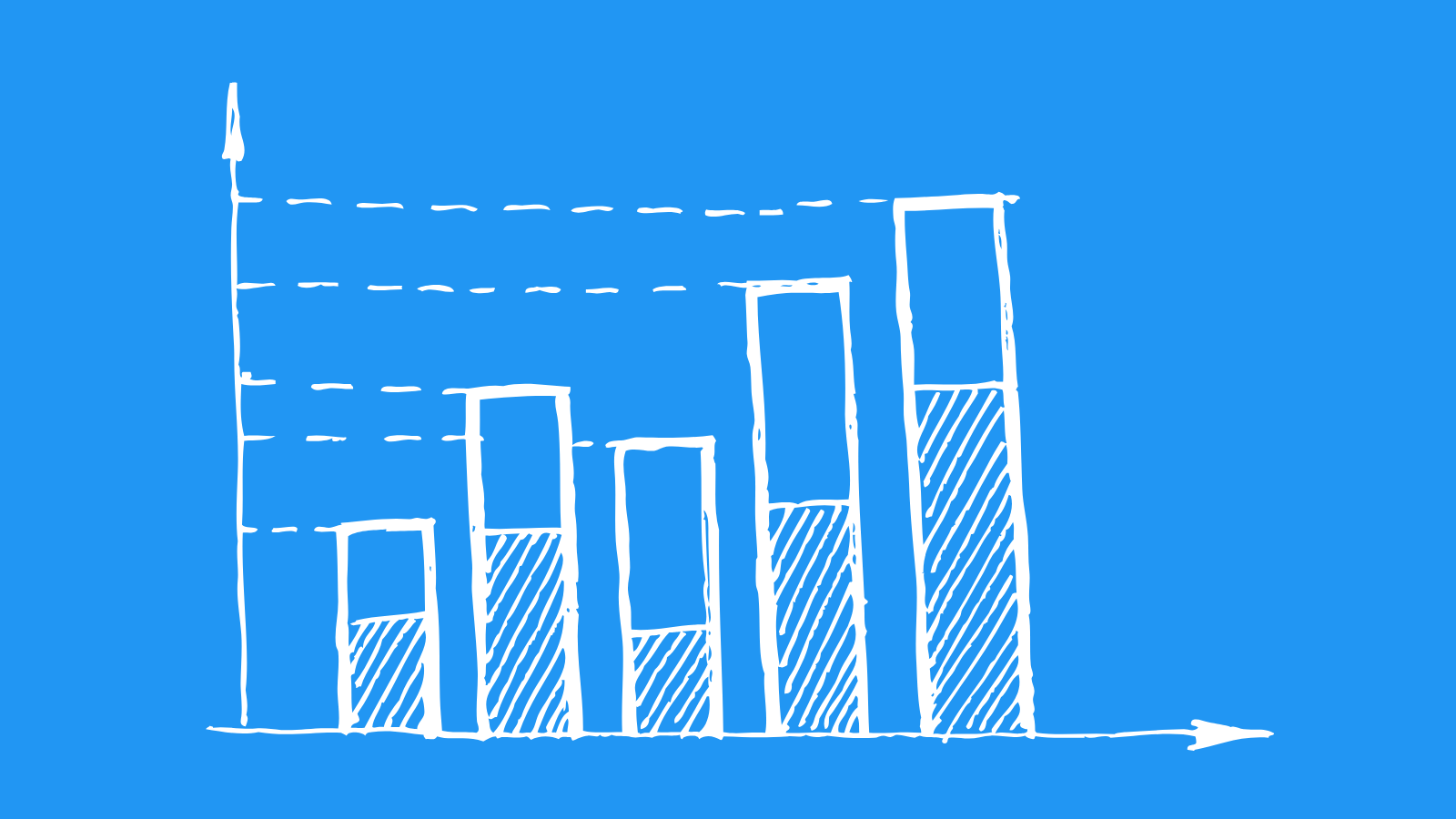
SUZ Stock: A Machine Learning Forecasting Model
Our team proposes a sophisticated machine learning model to forecast the performance of Suzano S.A. American Depositary Shares (SUZ). The model will leverage a diverse range of data inputs. This encompasses historical stock performance data, including opening, closing, high, and low values, trading volume, and technical indicators such as moving averages, Relative Strength Index (RSI), and Moving Average Convergence Divergence (MACD). Crucially, we will incorporate fundamental analysis data, including Suzano's quarterly and annual financial statements. These include revenue, earnings per share (EPS), debt levels, and cash flow. Furthermore, we will integrate macroeconomic indicators relevant to the pulp and paper industry, such as global GDP growth, interest rates, inflation rates, and currency exchange rates (particularly the Brazilian Real/USD rate). External factors, like industry-specific news, competitor performance, and climate change-related risks, will be incorporated through sentiment analysis of news articles and social media data.
The model will employ an ensemble approach, combining several machine learning algorithms for robust prediction. These algorithms will include Recurrent Neural Networks (RNNs), specifically Long Short-Term Memory (LSTM) networks, suitable for time-series data analysis. Random Forests, which are good at handling non-linear relationships and complex datasets, will also be included. Support Vector Machines (SVMs) will be considered to improve generalization and to capture trends effectively. Each model will be trained on a portion of the historical data, with the remaining data used for validation and testing. The performance of each model will be evaluated using metrics such as mean absolute error (MAE), mean squared error (MSE), and the R-squared. The results of each model will then be combined, using a weighted average approach, to produce the final forecast. Regular re-training and model recalibration will be a critical component to ensure the model's accuracy.
This model will generate forecasts for specific time horizons, from short-term (e.g., daily or weekly) to mid-term (e.g., monthly). The model will provide not only point predictions but also confidence intervals, reflecting the uncertainty associated with the forecasts. Furthermore, the model will facilitate scenario analysis. It will allow us to simulate the impact of different economic conditions, industry events, or policy changes on Suzano's stock performance. The insights gained from this forecasting model will be used to inform investment decisions, risk management strategies, and strategic planning within the company. Continuous monitoring and model improvements, based on new data and evolving market dynamics, will be a key part of model maintenance.
ML Model Testing
n:Time series to forecast
p:Price signals of Suzano S.A. stock
j:Nash equilibria (Neural Network)
k:Dominated move of Suzano S.A. stock holders
a:Best response for Suzano S.A. target price
For further technical information as per how our model work we invite you to visit the article below:
How do KappaSignal algorithms actually work?
Suzano S.A. Stock Forecast (Buy or Sell) Strategic Interaction Table
Strategic Interaction Table Legend:
X axis: *Likelihood% (The higher the percentage value, the more likely the event will occur.)
Y axis: *Potential Impact% (The higher the percentage value, the more likely the price will deviate.)
Z axis (Grey to Black): *Technical Analysis%
Suzano's Financial Outlook and Forecast
Suzano, a prominent player in the global pulp and paper industry, is currently navigating a complex and dynamic market landscape. The company's financial outlook is intricately tied to several key factors, including global pulp demand, currency fluctuations, and the overall economic health of its primary markets. The company's strategic investments in sustainable forestry and its focus on expanding its product portfolio, including dissolving pulp and paper products, are expected to be positive contributors to long-term value creation. Furthermore, Suzano's ongoing efforts to enhance operational efficiency and manage production costs will play a crucial role in maintaining profitability. However, the company's financial performance will continue to be influenced by external variables, such as global trade policies and the impact of environmental regulations.
The demand for pulp, a key raw material for paper and other products, is expected to remain robust, driven by the increasing consumption of tissue paper and packaging materials globally. Suzano, with its substantial production capacity and strategic geographic positioning, is well-positioned to capitalize on this trend. The company's significant investments in expanding its eucalyptus plantations and optimizing its supply chain will further strengthen its competitive advantage. Currency exchange rates, particularly the Brazilian real against the U.S. dollar, are a major factor influencing Suzano's financial performance, as a large portion of its revenue is generated in U.S. dollars while a significant portion of its operating expenses are denominated in Brazilian reais. Management's ability to strategically hedge against currency risk will be essential to preserving profitability and maximizing shareholder value.
The company's strategic moves towards sustainability are becoming increasingly important. Suzano's commitment to responsible forestry practices, including carbon footprint reduction and biodiversity preservation, aligns with the growing demand for environmentally friendly products and should enhance its brand reputation and access to capital. The company is also focused on expanding its product offerings beyond traditional pulp and paper. Investments in the development of bio-based products and dissolving pulp for textile applications could provide significant growth opportunities. While these diversification efforts may involve higher initial investment costs, they are expected to generate higher margins and enhance the company's long-term growth prospects. This strategic approach positions the company for resilience and growth in a world where sustainability is a critical consideration.
Overall, the forecast for Suzano is positive, underpinned by strong demand for pulp and paper products, strategic investments, and the company's commitment to sustainability. The successful execution of its long-term strategies will be crucial to delivering these positive outcomes. However, the company faces certain risks. Volatility in pulp prices, currency exchange rates, and economic downturns could negatively affect financial results. Furthermore, changes in environmental regulations or trade policies could also pose challenges. Despite these risks, Suzano is strategically positioned to navigate these challenges and take advantage of opportunities. Therefore, the company is likely to see a sustained positive performance over the next few years, with successful management of its risks being a key factor.
Rating | Short-Term | Long-Term Senior |
---|---|---|
Outlook | B2 | Ba2 |
Income Statement | Ba1 | Baa2 |
Balance Sheet | C | Baa2 |
Leverage Ratios | Baa2 | Baa2 |
Cash Flow | Caa2 | B2 |
Rates of Return and Profitability | B2 | Caa2 |
*Financial analysis is the process of evaluating a company's financial performance and position by neural network. It involves reviewing the company's financial statements, including the balance sheet, income statement, and cash flow statement, as well as other financial reports and documents.
How does neural network examine financial reports and understand financial state of the company?
References
- R. Williams. Simple statistical gradient-following algorithms for connectionist reinforcement learning. Ma- chine learning, 8(3-4):229–256, 1992
- Mnih A, Kavukcuoglu K. 2013. Learning word embeddings efficiently with noise-contrastive estimation. In Advances in Neural Information Processing Systems, Vol. 26, ed. Z Ghahramani, M Welling, C Cortes, ND Lawrence, KQ Weinberger, pp. 2265–73. San Diego, CA: Neural Inf. Process. Syst. Found.
- Bennett J, Lanning S. 2007. The Netflix prize. In Proceedings of KDD Cup and Workshop 2007, p. 35. New York: ACM
- Athey S, Blei D, Donnelly R, Ruiz F. 2017b. Counterfactual inference for consumer choice across many prod- uct categories. AEA Pap. Proc. 108:64–67
- Chipman HA, George EI, McCulloch RE. 2010. Bart: Bayesian additive regression trees. Ann. Appl. Stat. 4:266–98
- Wager S, Athey S. 2017. Estimation and inference of heterogeneous treatment effects using random forests. J. Am. Stat. Assoc. 113:1228–42
- V. Borkar. Q-learning for risk-sensitive control. Mathematics of Operations Research, 27:294–311, 2002.