AUC Score :
Short-term Tactic1 :
Dominant Strategy :
Time series to forecast n:
ML Model Testing : Statistical Inference (ML)
Hypothesis Testing : Polynomial Regression
Surveillance : Major exchange and OTC
1Short-term revised.
2Time series is updated based on short-term trends.
Key Points
SLEI faces a mixed outlook. Increased adoption of its AI-powered platform for content creation and distribution, coupled with strategic partnerships, could drive substantial revenue growth and market share expansion. Positive consumer sentiment and a successful rollout of new products would further enhance financial performance. However, SLEI is exposed to several risks. Intense competition within the content creation and distribution industry could squeeze profit margins and hinder growth. Technological disruptions, particularly advancements in generative AI, pose a threat if SLEI fails to innovate and adapt quickly. Economic downturns could reduce consumer spending on digital content, impacting SLEI's financial results. Regulatory scrutiny and changes in data privacy laws could also affect the company's operations.About Super League Enterprise
Super League Enterprise Inc. (SLGG) is a digital gaming and esports entertainment company focused on building experiences across the metaverse. The company primarily operates in the rapidly expanding gaming industry, facilitating community building and content creation within popular video game titles. SLGG's business model revolves around providing tools, services, and infrastructure to game developers, content creators, and players, fostering engagement and monetization opportunities within digital gaming ecosystems. Its focus is on live streaming, interactive experiences, and virtual events to attract and retain audiences.
SLGG generates revenue through various channels, including advertising, sponsorships, and licensing agreements related to its proprietary platforms and technologies. The company aims to capitalize on the growing popularity of esports and online gaming by expanding its reach and services, creating a bridge between game developers and players. SLGG strives to cultivate a strong community platform where users can engage with games, create content, and interact with each other, creating a multifaceted and dynamic digital entertainment environment.
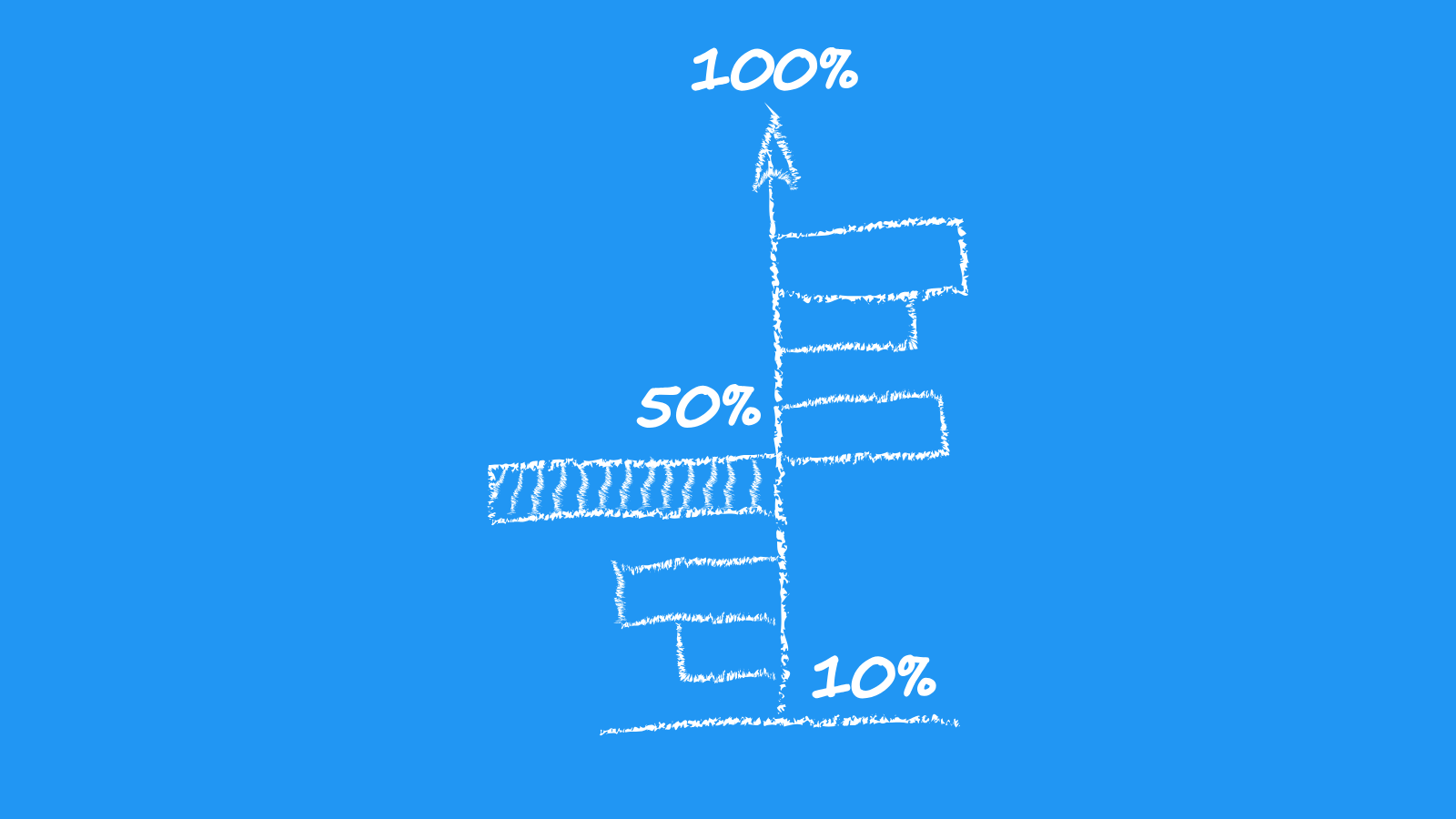
SLE Stock Forecasting Model: A Data Science and Economics Approach
For Super League Enterprise Inc. (SLE), our team of data scientists and economists has developed a comprehensive machine learning model for stock price forecasting. The core of our model leverages a diverse range of data sources, including historical SLE trading data (volume, open, high, low, and close prices), financial statements (revenue, earnings per share, debt levels, cash flow), and market sentiment indicators (news articles, social media analysis). We incorporate macroeconomic variables such as interest rates, inflation rates, and GDP growth to capture broader economic trends that may impact the company's performance. Our model utilizes a hybrid approach, combining multiple machine learning algorithms, including Recurrent Neural Networks (RNNs), specifically LSTMs for time series analysis, Random Forest Regression for feature importance and ensemble learning and Support Vector Machines (SVMs) for capturing non-linear relationships, optimized to handle the complexities of financial time series data.
Feature engineering is a critical component of our model. We create both technical indicators (moving averages, Relative Strength Index, Moving Average Convergence Divergence) and fundamental indicators (price-to-earnings ratio, debt-to-equity ratio) from the raw data, transforming data into an informative state that helps the model to learn. Feature selection is crucial to avoid overfitting. We employ techniques such as recursive feature elimination and Lasso regularization to identify and prioritize the most predictive variables, thereby enhancing model accuracy and interpretability. The model is trained on a significant historical dataset, and the training is done with a rolling window approach to ensure the model adapts to changing market conditions. To improve performance, a model ensemble technique is utilized to combine the outputs of different models. This method improves the accuracy and robustness of our forecasts by reducing the impact of any single model's errors.
Model validation is conducted using techniques like cross-validation and out-of-sample testing to ensure robust performance. Performance metrics include Mean Squared Error (MSE), Mean Absolute Error (MAE), and R-squared to evaluate model accuracy. The model outputs forecasts at different horizons (e.g., daily, weekly). We intend to continually refine the model by incorporating new data, adjusting model parameters based on performance feedback, and integrating additional relevant variables like competitor analysis and regulatory changes. The model results will be presented with confidence intervals, and the predictions are to be utilized by financial analysts to support their investment decisions regarding SLE stock, with the understanding that all forecasts come with an element of risk and uncertainty inherent in financial markets. Furthermore, the model will be regularly reviewed and updated to maintain its predictive power.
```
ML Model Testing
n:Time series to forecast
p:Price signals of Super League Enterprise stock
j:Nash equilibria (Neural Network)
k:Dominated move of Super League Enterprise stock holders
a:Best response for Super League Enterprise target price
For further technical information as per how our model work we invite you to visit the article below:
How do KappaSignal algorithms actually work?
Super League Enterprise Stock Forecast (Buy or Sell) Strategic Interaction Table
Strategic Interaction Table Legend:
X axis: *Likelihood% (The higher the percentage value, the more likely the event will occur.)
Y axis: *Potential Impact% (The higher the percentage value, the more likely the price will deviate.)
Z axis (Grey to Black): *Technical Analysis%
Financial Outlook and Forecast for Super League Enterprise
Super League's financial trajectory appears poised for a period of growth, primarily fueled by its expanding presence in the burgeoning esports and digital entertainment sectors. The company's business model, centered on providing tools and platforms for creators, brands, and esports organizers, positions it well to capitalize on the escalating demand for interactive entertainment experiences. Strategic partnerships and acquisitions, like the acquisition of Metaverse Gaming Network, have expanded its capabilities and audience reach. The company's ability to monetize its offerings through advertising, sponsorships, and in-app purchases suggests a diversified revenue stream, mitigating reliance on a single source. Increased user engagement and content creation on its platforms are crucial indicators of positive momentum, which can translate into higher revenues. Growth in the esports market generally indicates a growing market share for the company.
The forecast anticipates steady revenue growth for Super League over the next few years. Investment in technology infrastructure and platform development should support its expansion in the market. The company's expansion into new markets and initiatives to acquire more customers are expected to contribute positively to its financial performance. Furthermore, the increasing adoption of digital entertainment is expected to boost its customer base and the value proposition of its offerings, potentially improving profit margins. Strong financial discipline and efficient operational management are essential to achieving these objectives. This also shows that the company must increase the speed of innovation as well, making it competitive in the market.
The company's financial statements reveal that Super League has invested heavily in building its infrastructure and platform offerings. This capital expenditure is a strategic move, paving the way for long-term scalability and competitiveness. Super League also has a strong cash reserve, as well as strategic partnerships that should provide them with additional growth capital and operational efficiencies. The market's strong liquidity and the continued influx of investment into the digital entertainment industry create a favorable environment for Super League to secure further funding and execute its growth strategy effectively.
The outlook for Super League is generally positive. However, the company faces a number of risks. Intense competition from established players in the digital entertainment sector, coupled with the dynamic nature of consumer preferences, creates an environment that can pose a challenge for future profitability. Reliance on advertising revenue can make Super League sensitive to cyclical changes in the advertising market. To maintain its growth trajectory, the company must continue to innovate, attract and retain users and adapt to changing market dynamics. Despite these risks, Super League's strategic positioning in a rapidly growing market provides a solid foundation for future financial success.
Rating | Short-Term | Long-Term Senior |
---|---|---|
Outlook | Caa2 | B2 |
Income Statement | C | Caa2 |
Balance Sheet | C | Ba2 |
Leverage Ratios | C | Caa2 |
Cash Flow | C | B2 |
Rates of Return and Profitability | Caa2 | C |
*Financial analysis is the process of evaluating a company's financial performance and position by neural network. It involves reviewing the company's financial statements, including the balance sheet, income statement, and cash flow statement, as well as other financial reports and documents.
How does neural network examine financial reports and understand financial state of the company?
References
- M. L. Littman. Friend-or-foe q-learning in general-sum games. In Proceedings of the Eighteenth International Conference on Machine Learning (ICML 2001), Williams College, Williamstown, MA, USA, June 28 - July 1, 2001, pages 322–328, 2001
- Brailsford, T.J. R.W. Faff (1996), "An evaluation of volatility forecasting techniques," Journal of Banking Finance, 20, 419–438.
- Mikolov T, Chen K, Corrado GS, Dean J. 2013a. Efficient estimation of word representations in vector space. arXiv:1301.3781 [cs.CL]
- Hastie T, Tibshirani R, Tibshirani RJ. 2017. Extended comparisons of best subset selection, forward stepwise selection, and the lasso. arXiv:1707.08692 [stat.ME]
- Akgiray, V. (1989), "Conditional heteroscedasticity in time series of stock returns: Evidence and forecasts," Journal of Business, 62, 55–80.
- Clements, M. P. D. F. Hendry (1995), "Forecasting in cointegrated systems," Journal of Applied Econometrics, 10, 127–146.
- A. Tamar and S. Mannor. Variance adjusted actor critic algorithms. arXiv preprint arXiv:1310.3697, 2013.