AUC Score :
Short-term Tactic1 :
Dominant Strategy :
Time series to forecast n:
ML Model Testing : Modular Neural Network (DNN Layer)
Hypothesis Testing : ElasticNet Regression
Surveillance : Major exchange and OTC
1Short-term revised.
2Time series is updated based on short-term trends.
Key Points
SunOpta faces a future marked by both opportunities and challenges. The company is likely to see growth in its plant-based food and beverage segment due to increasing consumer demand, potentially leading to increased revenue and market share. However, the company's profitability could be hindered by rising input costs such as raw materials and transportation, alongside intense competition in the crowded food industry. Another risk includes supply chain disruptions, which could impact production and distribution. Despite these risks, the company's focus on innovation and strategic partnerships could help mitigate negative impacts and drive future expansion.About SunOpta Inc.
SunOpta is a global company specializing in the sourcing, processing, and packaging of organic and non-GMO food products and beverages. Its operations span multiple categories, including plant-based beverages, fruit-based snacks, and ingredients for the food industry. The company focuses on providing innovative and sustainable solutions to meet evolving consumer demands for healthy and ethically sourced products. Sourcing products from farms and working with suppliers worldwide, SunOpta aims to ensure quality and traceability throughout its supply chain.
SunOpta's strategy emphasizes growth through product innovation, expansion of its production capacity, and strategic partnerships. The company is committed to sustainability, with initiatives focused on reducing environmental impact, supporting ethical sourcing practices, and promoting transparency. This dedication to sustainability and consumer health positions the company for potential growth in the evolving food and beverage market. Its operations are split into segments, including plant-based food and beverage, and fruit-based foods and snacks.
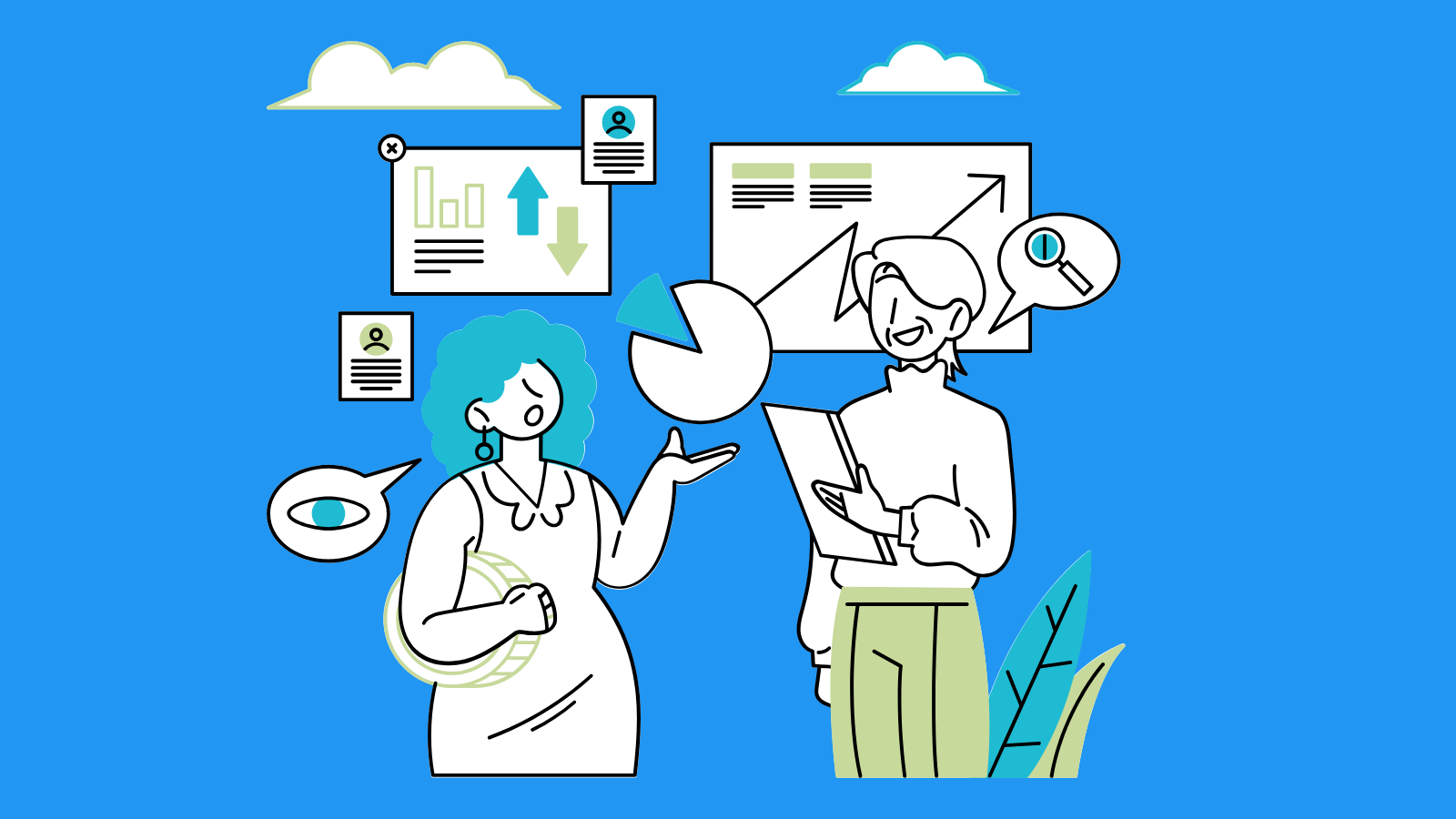
STKL Stock Forecast Model: A Data Science and Economics Approach
Our team of data scientists and economists has developed a machine learning model to forecast the performance of SunOpta Inc. (STKL) common stock. The model utilizes a combination of technical indicators, fundamental analysis, and macroeconomic data to provide a comprehensive view. The technical component incorporates historical stock price data, including moving averages, Relative Strength Index (RSI), and Volume, to identify patterns and predict future price movements. Fundamental data involves analyzing SunOpta's financial statements, such as revenue, earnings per share (EPS), debt levels, and profit margins. This assessment helps determine the company's intrinsic value and growth potential. The macroeconomic data incorporates external factors, such as inflation rates, interest rates, consumer sentiment, and commodity prices, which can significantly impact the agricultural and food processing industries in which SunOpta operates.
The model's architecture involves several machine learning algorithms. We employ time series analysis techniques such as ARIMA (Autoregressive Integrated Moving Average) and Exponential Smoothing methods to capture temporal dependencies and forecast future values based on historical stock price patterns. Additionally, we utilize ensemble methods, like Random Forests and Gradient Boosting, to integrate the insights derived from technical, fundamental, and macroeconomic data. These algorithms are trained on a substantial historical dataset, spanning multiple years, to ensure robust and accurate predictions. Feature engineering is a crucial aspect of the model, where we create new variables from existing data, such as ratio analysis derived from financial statements and volatility measures from price fluctuations. The model's performance is evaluated using metrics such as Mean Squared Error (MSE) and R-squared to assess its accuracy and predictive power.
Our final model provides a probabilistic forecast, generating a range of potential outcomes rather than a single point prediction, which accounts for the inherent uncertainties in stock market analysis. The model output includes predicted directional trends (up, down, or sideways), along with confidence intervals. Risk management is integral to our approach. We incorporate backtesting to assess the model's performance over various historical periods and stress testing to evaluate its resilience under extreme market conditions. The output is designed to be used in conjunction with other forms of investment analysis, providing insights that can contribute to more informed investment decisions. We will continue to update and refine the model. The model's accuracy will depend on the quality and reliability of the input data, the choice of the algorithms, and the evolving market conditions.
ML Model Testing
n:Time series to forecast
p:Price signals of SunOpta Inc. stock
j:Nash equilibria (Neural Network)
k:Dominated move of SunOpta Inc. stock holders
a:Best response for SunOpta Inc. target price
For further technical information as per how our model work we invite you to visit the article below:
How do KappaSignal algorithms actually work?
SunOpta Inc. Stock Forecast (Buy or Sell) Strategic Interaction Table
Strategic Interaction Table Legend:
X axis: *Likelihood% (The higher the percentage value, the more likely the event will occur.)
Y axis: *Potential Impact% (The higher the percentage value, the more likely the price will deviate.)
Z axis (Grey to Black): *Technical Analysis%
SunOpta Inc. Common Stock Financial Outlook and Forecast
The financial outlook for SunOpta (STKL) appears moderately positive, contingent upon the successful execution of its strategic initiatives. The company is positioned within the rapidly expanding plant-based food and beverage sector, a market experiencing substantial growth driven by increasing consumer demand for healthier and more sustainable food choices. SunOpta has actively invested in expanding its production capacity, optimizing its supply chain, and innovating its product portfolio to capitalize on this trend. Recent financial reports demonstrate a consistent rise in revenue, primarily fueled by the expansion of its plant-based food and beverage segment. Furthermore, the company's focus on cost management and operational efficiency has started to yield improvements in its profitability margins. The strategic acquisitions and partnerships that SunOpta is engaged in has helped to diversify its product offerings and distribution channels which is predicted to enhance its overall market share, which is a favorable indicator of future growth.
Analyses of SunOpta's financial performance reveal that its growth potential relies on continued investment in its core competencies. The company's success hinges on several factors. Firstly, effective supply chain management, specifically relating to the sourcing of raw materials and logistics. Fluctuations in commodity prices and disruptions in supply chains can negatively impact the company's profitability. Secondly, maintaining its innovation pipeline to introduce new products to meet the evolving tastes of the consumers. Thirdly, the ability of SunOpta to successfully integrate acquired businesses and capitalize on market opportunities is a critical element. Also, it is crucial that the company is able to successfully compete against industry giants in the plant-based food industry.
Looking ahead, analysts forecast steady revenue growth for STKL, fueled by the ongoing expansion of the plant-based food market and SunOpta's strategic investments in its production capabilities. It is predicted that the company will successfully navigate the challenging economic environment and inflationary pressures. While supply chain uncertainties and inflationary pressures may present short-term challenges, SunOpta's focus on high-margin products and its expansion strategy are expected to bolster its revenue and improve its profitability. Strategic partnerships with key retailers and food service providers are also critical in supporting the growth trajectory. The company is well-positioned to benefit from the expanding market and increasing consumer demand for plant-based options.
In conclusion, the financial forecast for STKL is cautiously optimistic. The primary prediction is for sustained revenue growth and gradual improvements in profitability over the next few years. However, this positive outlook is subject to certain risks. These include commodity price volatility, potential supply chain disruptions, and intense competition from both established food manufacturers and emerging plant-based food companies. Also, economic uncertainty and changes in consumer spending patterns could create an impact on demand. Ultimately, SunOpta's ability to navigate these risks and maintain its growth trajectory will determine its long-term financial performance. The company must remain agile and responsive to changing market dynamics to achieve its full potential.
Rating | Short-Term | Long-Term Senior |
---|---|---|
Outlook | B1 | Ba3 |
Income Statement | Baa2 | Baa2 |
Balance Sheet | B3 | Baa2 |
Leverage Ratios | B3 | Ba2 |
Cash Flow | Baa2 | C |
Rates of Return and Profitability | B3 | C |
*Financial analysis is the process of evaluating a company's financial performance and position by neural network. It involves reviewing the company's financial statements, including the balance sheet, income statement, and cash flow statement, as well as other financial reports and documents.
How does neural network examine financial reports and understand financial state of the company?
References
- S. Proper and K. Tumer. Modeling difference rewards for multiagent learning (extended abstract). In Proceedings of the Eleventh International Joint Conference on Autonomous Agents and Multiagent Systems, Valencia, Spain, June 2012
- Athey S. 2017. Beyond prediction: using big data for policy problems. Science 355:483–85
- Hornik K, Stinchcombe M, White H. 1989. Multilayer feedforward networks are universal approximators. Neural Netw. 2:359–66
- M. J. Hausknecht and P. Stone. Deep recurrent Q-learning for partially observable MDPs. CoRR, abs/1507.06527, 2015
- V. Konda and J. Tsitsiklis. Actor-Critic algorithms. In Proceedings of Advances in Neural Information Processing Systems 12, pages 1008–1014, 2000
- Firth JR. 1957. A synopsis of linguistic theory 1930–1955. In Studies in Linguistic Analysis (Special Volume of the Philological Society), ed. JR Firth, pp. 1–32. Oxford, UK: Blackwell
- Gentzkow M, Kelly BT, Taddy M. 2017. Text as data. NBER Work. Pap. 23276