AUC Score :
Short-term Tactic1 :
Dominant Strategy :
Time series to forecast n:
ML Model Testing : Modular Neural Network (Emotional Trigger/Responses Analysis)
Hypothesis Testing : Polynomial Regression
Surveillance : Major exchange and OTC
1Short-term revised.
2Time series is updated based on short-term trends.
Key Points
SEDU's future appears cautiously optimistic. The company is likely to experience moderate growth in its online education programs, driven by ongoing demand for flexible learning options. However, SEDU faces potential risks, including increased competition from both traditional universities and emerging online platforms. Regulatory changes in the higher education sector could impact its operations and profitability, requiring SEDU to adapt its programs and strategies. Economic downturns could also negatively affect enrollment rates. Furthermore, maintaining and improving the quality of its programs will be essential for retaining students and preserving its reputation. The company's success will depend on its ability to navigate these challenges and capitalize on opportunities in the evolving education landscape.About Strategic Education Inc.
Strategic Education, Inc. (SEI) is a provider of postsecondary education primarily focused on online degree programs. The company operates through two main segments: Strayer University and Capella University. Strayer University targets working adults seeking career-focused degrees, while Capella University caters to a broader audience, including those pursuing advanced degrees like master's and doctoral programs. SEI's programs cover various fields, including business, technology, healthcare, and public service.
SEI emphasizes flexible learning options and aims to help students gain skills relevant to in-demand careers. The company invests in technologies and resources to support online learning environments, faculty development, and student support services, like career counseling and financial aid guidance. It is subject to regulations set by the U.S. Department of Education and accrediting bodies. SEI's goal is to offer accessible, career-oriented education to a diverse student population.
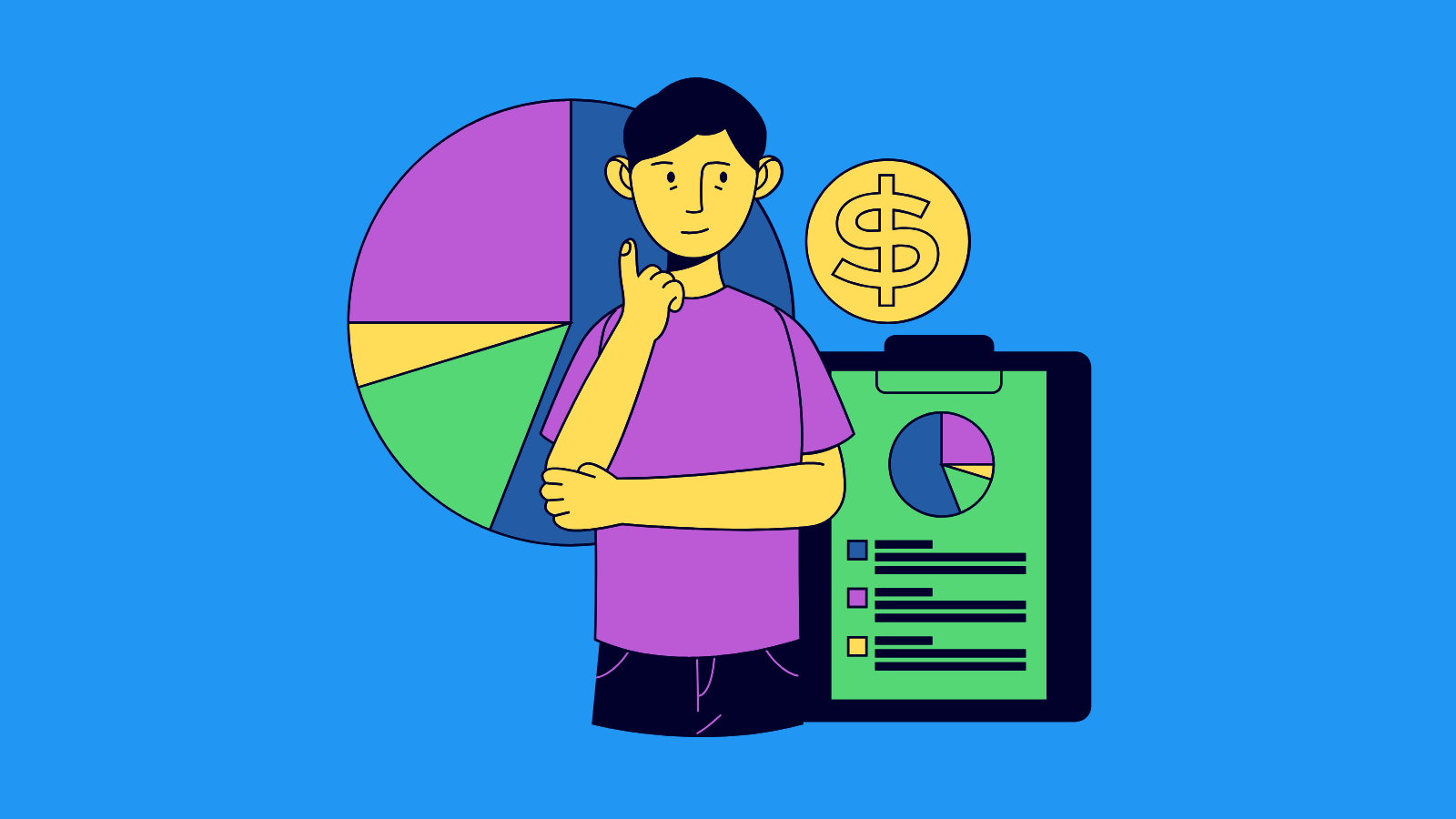
STRA Stock Forecast Model
Our team has developed a machine learning model to forecast the performance of Strategic Education Inc. (STRA) common stock. This model leverages a combination of advanced econometric techniques and machine learning algorithms. The core of our model is a Time Series Analysis component, which analyzes historical STRA stock data, incorporating factors such as trading volume, price volatility, and moving averages to identify underlying patterns and trends. We employ techniques like ARIMA (Autoregressive Integrated Moving Average) and Exponential Smoothing to forecast future stock movements based on these identified time-dependent relationships. Further enhancing the model's predictive capabilities, we incorporate a fundamental analysis module, evaluating the company's financial health through key metrics like revenue, earnings per share (EPS), debt-to-equity ratio, and cash flow. We collect and process data from various financial databases and utilize data augmentation techniques to handle potential data scarcity issues.
To enhance the model's accuracy, we integrate sentiment analysis derived from financial news articles, social media posts, and investor forums. This sentiment data provides valuable insights into market perception of Strategic Education Inc., allowing the model to account for external factors that might influence stock behavior. Our machine learning model utilizes a hybrid approach, integrating the time series data with financial metrics and sentiment analysis. We employ algorithms like Random Forests, Gradient Boosting, and Support Vector Machines (SVMs) to train the model. These models are effective at capturing complex non-linear relationships within the data. We regularly validate the model's performance through rigorous testing using out-of-sample data, calculating metrics such as Mean Absolute Error (MAE), Root Mean Squared Error (RMSE), and R-squared to ensure its accuracy and reliability. We are using different optimisation techniques and hyperparameter tuning to improve the model.
The final model generates probabilistic forecasts, providing not only predicted STRA stock movement but also a confidence interval around the prediction. We will regularly update the model to adapt to changing market conditions and incorporate new data. We also offer a risk management component which will analyze the potential risks in different investments, with suggestions on diversification. This allows for more informed trading and investment decisions. We anticipate the model will provide valuable insight into the future performance of STRA stock, supporting the formulation of data-driven investment strategies and assisting in better allocation of resources. This model is also capable of being customized and tailored to individual investor risk profiles and investment horizons.
ML Model Testing
n:Time series to forecast
p:Price signals of Strategic Education Inc. stock
j:Nash equilibria (Neural Network)
k:Dominated move of Strategic Education Inc. stock holders
a:Best response for Strategic Education Inc. target price
For further technical information as per how our model work we invite you to visit the article below:
How do KappaSignal algorithms actually work?
Strategic Education Inc. Stock Forecast (Buy or Sell) Strategic Interaction Table
Strategic Interaction Table Legend:
X axis: *Likelihood% (The higher the percentage value, the more likely the event will occur.)
Y axis: *Potential Impact% (The higher the percentage value, the more likely the price will deviate.)
Z axis (Grey to Black): *Technical Analysis%
Strategic Education Inc. (SEI) Financial Outlook and Forecast
SEI, a leading provider of educational services primarily through its Strayer University and Capella University brands, presents a mixed financial outlook. The company is undergoing a strategic transformation focusing on expanding its high-quality online programs and strengthening its relationship with employers to improve student outcomes and drive enrollment growth. Key drivers for this positive trajectory include the increasing demand for online education, particularly in fields like healthcare and technology, where SEI has a strong presence. The company's ability to adapt to changing educational needs, offer flexible learning options, and develop industry-relevant curricula positions it well to capture a larger share of the growing online education market. Furthermore, SEI's focus on supporting student success through career services and employer partnerships should enhance its value proposition and attract more students, therefore increasing revenue.
Financial performance is expected to be influenced by several factors. Revenue growth is anticipated, though the pace will be influenced by enrollment trends and program mix. Operating expenses may experience fluctuation due to investments in technology, marketing, and new program development. The company's profitability will be affected by its ability to manage costs effectively and maintain a high level of student retention. Moreover, SEI's investment in its digital infrastructure and technology platforms is intended to improve operational efficiency and enhance the student experience. SEI has been actively pursuing strategic partnerships and acquisitions to diversify its offerings and expand its reach into new markets, which can potentially lead to new revenue streams and growth opportunities.
In terms of specific financial metrics, analysts predict a gradual increase in revenue over the next few years. While net income may be impacted by strategic investments, the focus is on building a sustainable growth model. SEI's financial health is supported by a strong balance sheet and a commitment to prudent financial management. The company is expected to maintain a healthy cash flow generation that enables it to invest in future growth initiatives and return value to shareholders. The overall financial forecasts suggest a period of moderate but steady growth for SEI, although the actual performance may vary depending on external factors and the success of its strategic initiatives. The company's focus on improving student outcomes should also help mitigate the impact of the competitive educational landscape.
The overall forecast for SEI is cautiously positive, with the expectation of continued growth. This is predicated on SEI's effective execution of its strategic plan, the ongoing demand for online education, and the company's ability to offer relevant and engaging programs. However, there are risks. A significant decline in enrollment, increased competition from other online educational providers, or unfavorable changes in regulations could negatively impact financial performance. Any disruptions or negative economic conditions could also impede the growth. Finally, a failure to execute on strategic partnerships or integrating acquisitions successfully may jeopardize the growth outlook. In the long term, SEI needs to consistently maintain a high-quality educational experience while successfully adjusting to market needs.
Rating | Short-Term | Long-Term Senior |
---|---|---|
Outlook | B1 | Ba3 |
Income Statement | B3 | C |
Balance Sheet | Caa2 | Ba2 |
Leverage Ratios | Baa2 | Baa2 |
Cash Flow | Baa2 | Baa2 |
Rates of Return and Profitability | C | Caa2 |
*Financial analysis is the process of evaluating a company's financial performance and position by neural network. It involves reviewing the company's financial statements, including the balance sheet, income statement, and cash flow statement, as well as other financial reports and documents.
How does neural network examine financial reports and understand financial state of the company?
References
- Dietterich TG. 2000. Ensemble methods in machine learning. In Multiple Classifier Systems: First International Workshop, Cagliari, Italy, June 21–23, pp. 1–15. Berlin: Springer
- J. Ott. A Markov decision model for a surveillance application and risk-sensitive Markov decision processes. PhD thesis, Karlsruhe Institute of Technology, 2010.
- Scott SL. 2010. A modern Bayesian look at the multi-armed bandit. Appl. Stoch. Models Bus. Ind. 26:639–58
- Hastie T, Tibshirani R, Wainwright M. 2015. Statistical Learning with Sparsity: The Lasso and Generalizations. New York: CRC Press
- J. Harb and D. Precup. Investigating recurrence and eligibility traces in deep Q-networks. In Deep Reinforcement Learning Workshop, NIPS 2016, Barcelona, Spain, 2016.
- Vilnis L, McCallum A. 2015. Word representations via Gaussian embedding. arXiv:1412.6623 [cs.CL]
- K. Boda and J. Filar. Time consistent dynamic risk measures. Mathematical Methods of Operations Research, 63(1):169–186, 2006