AUC Score :
Short-term Tactic1 :
Dominant Strategy :
Time series to forecast n:
ML Model Testing : Supervised Machine Learning (ML)
Hypothesis Testing : Logistic Regression
Surveillance : Major exchange and OTC
1Short-term revised.
2Time series is updated based on short-term trends.
Key Points
ReposiTrak faces a mixed outlook. Revenue growth is expected due to increasing demand for its software solutions within the food safety and supply chain management sectors, particularly as regulatory pressures intensify. However, competition from larger tech firms with broader offerings poses a substantial risk, potentially limiting market share gains. Integration challenges related to recent acquisitions could lead to operational inefficiencies and erode profitability. Additionally, economic downturns or supply chain disruptions could negatively impact the company's customer base and revenue streams.About ReposiTrak Inc.
ReposiTrak (RPTK) is a publicly traded company specializing in supply chain solutions for the grocery, foodservice, and pharmaceutical industries. They provide a cloud-based platform offering compliance management, supplier verification, and food safety solutions. Their services aim to improve transparency and traceability within the supply chain, helping businesses meet regulatory requirements and mitigate risks associated with product recalls and foodborne illnesses. Key features include document management, real-time monitoring, and communication tools designed to streamline interactions between suppliers, retailers, and other stakeholders.
ReposiTrak's platform facilitates compliance with regulations such as the Food Safety Modernization Act (FSMA). The company's focus is to help businesses manage data, verify supplier information, and trace products. Their network connects suppliers and retailers through a central platform that allows for the exchange of critical information and the monitoring of product movement. This focus on data management and compliance helps the company appeal to a variety of businesses of different sizes.
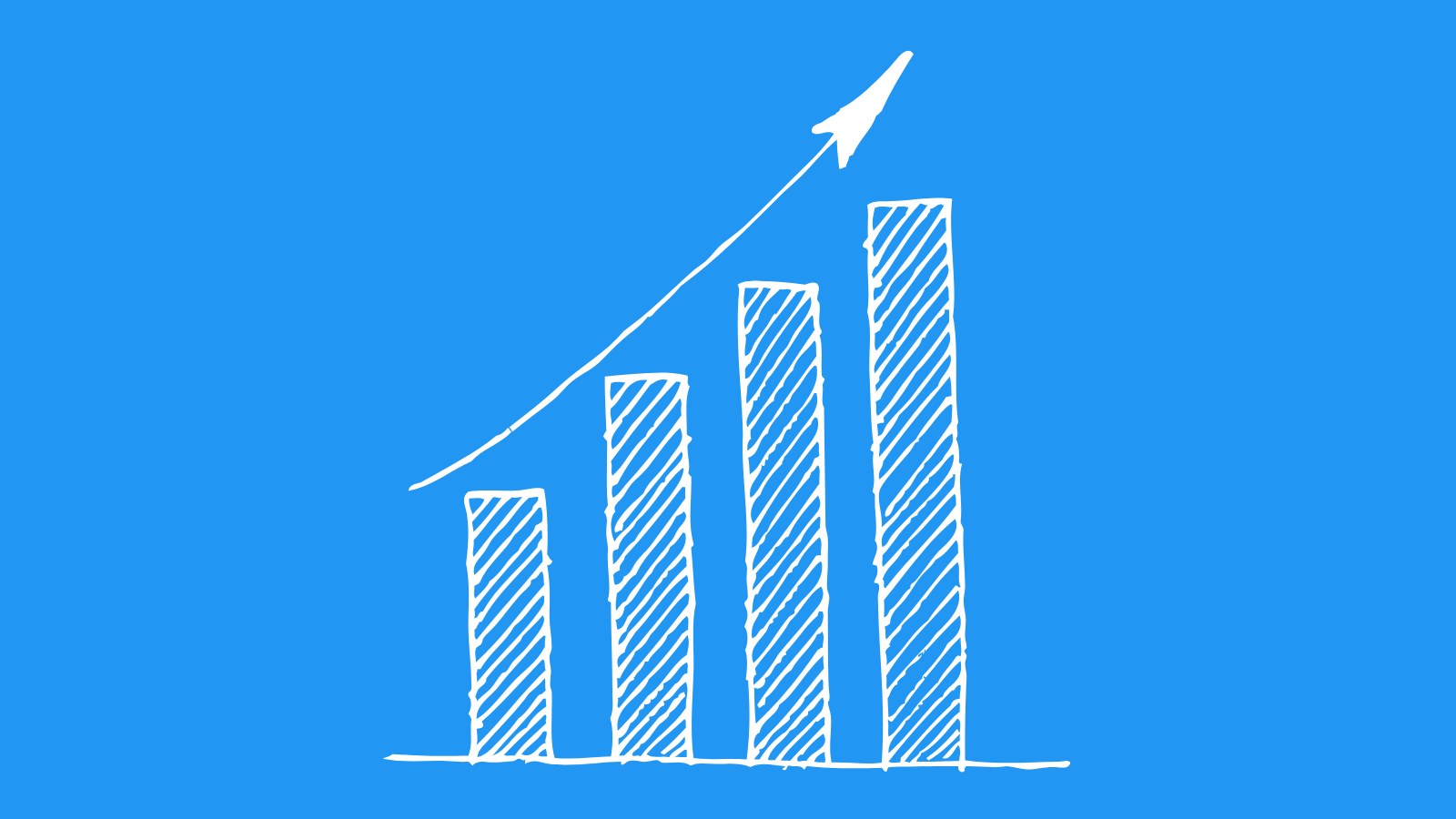
TRAK Stock Forecasting Model
Our team of data scientists and economists proposes a machine learning model to forecast the future performance of ReposiTrak Inc. (TRAK) common stock. The foundation of our model will involve a comprehensive analysis of both internal and external factors influencing the company's value. We will utilize a blended approach, incorporating time series analysis to identify historical patterns and trends in TRAK's stock performance, along with macroeconomic indicators such as inflation rates, interest rates, and GDP growth, which often correlate with market sentiment and investor behavior. Furthermore, we will leverage sentiment analysis techniques on news articles, social media, and financial reports to gauge public perception and identify potential catalysts for price fluctuations.
The model's architecture will center on a hybrid approach combining several machine learning algorithms. Initially, a Recurrent Neural Network (RNN), specifically a Long Short-Term Memory (LSTM) network, will be used to capture long-term dependencies within the time-series data, helping to identify intricate patterns in past price behavior and trading volume. Simultaneously, Gradient Boosting Machines, such as XGBoost, will be employed to incorporate the broader macroeconomic variables and sentiment scores, providing a more holistic view of the market dynamics. Feature engineering, including creating technical indicators, like moving averages and relative strength index (RSI), and scaling numerical features for better model performance will be a crucial part of the preprocessing step. Model evaluation will be conducted using various metrics, including Mean Absolute Error (MAE), Root Mean Squared Error (RMSE), and R-squared, on a held-out testing dataset.
To ensure the model's effectiveness and relevance, we will implement continuous monitoring and retraining strategies. This will involve regularly updating the model with new data and retraining it to account for shifting market dynamics. We will employ automated model versioning and A/B testing to assess the performance of different model versions and hyperparameters. Furthermore, we will incorporate feedback from financial analysts and market experts to refine our understanding of TRAK's business and incorporate qualitative insights into the model. This iterative process of development, evaluation, and improvement will be essential to maintaining the model's predictive accuracy and providing valuable insights for investment decisions related to TRAK stock.
```
ML Model Testing
n:Time series to forecast
p:Price signals of ReposiTrak Inc. stock
j:Nash equilibria (Neural Network)
k:Dominated move of ReposiTrak Inc. stock holders
a:Best response for ReposiTrak Inc. target price
For further technical information as per how our model work we invite you to visit the article below:
How do KappaSignal algorithms actually work?
ReposiTrak Inc. Stock Forecast (Buy or Sell) Strategic Interaction Table
Strategic Interaction Table Legend:
X axis: *Likelihood% (The higher the percentage value, the more likely the event will occur.)
Y axis: *Potential Impact% (The higher the percentage value, the more likely the price will deviate.)
Z axis (Grey to Black): *Technical Analysis%
ReposiTrak Inc. (RPTK) Financial Outlook and Forecast
The financial outlook for ReposiTrak (RPTK) appears promising, driven by its strong position within the critical supply chain and food safety compliance sector. The company's business model, centered on providing track-and-trace solutions and regulatory compliance tools to food suppliers and retailers, positions it well in a market increasingly focused on transparency and consumer safety. Key positive indicators include the ongoing regulatory pressures from agencies like the FDA, which mandates stricter food safety protocols, leading to increased demand for RPTK's services. Moreover, the company's subscription-based revenue model provides a degree of stability and predictability, allowing for better financial planning and sustainable growth. This, coupled with its expanding customer base across various segments of the food industry, suggests a consistent revenue stream and potential for market share expansion. Furthermore, RPTK's ability to integrate its services with existing industry systems and its ongoing investment in technology enhancements are contributing factors to its positive outlook, making it a highly adaptable and scalable company in its market.
Revenue growth for RPTK is expected to be steady, supported by continued customer acquisition and expansion of services within existing customer relationships. The company's ability to retain customers is a crucial element of this outlook, driven by its reputation for providing reliable and effective solutions. The expansion into adjacent markets, such as pharmaceutical supply chain management, also holds potential for revenue diversification and increased market penetration. Furthermore, the growth of the food safety market in the global landscape is highly favorable for RPTK, as international regulations and standards are tightening, which increases the company's addressable market. The company's focus on research and development, coupled with its strategic partnerships and acquisitions, will be key to unlocking further revenue streams and maintaining a competitive edge within the industry. Analysts predict that the growth will accelerate as businesses look to digitize their supply chains and comply with government and industry requirements, leading to increased demand for RPTK's services.
Profitability for RPTK is anticipated to improve over the forecast period. This positive trend is a result of several factors, including the scalability of the company's platform, which enables it to serve a growing customer base without a corresponding increase in operational costs. Its ongoing efforts to optimize operational efficiency and drive down costs are key factors. Furthermore, the high margins associated with its subscription-based revenue model are expected to contribute to improved profitability over time. With the increasing automation within the supply chain and the growing need for real-time data analytics, RPTK's offerings become even more valuable, contributing to improved profit margins. Moreover, as the company strengthens its market position and increases its brand recognition, its pricing power is likely to improve, leading to improved margins, and better profitability. It is predicted that as RPTK increases its customer base, profitability will improve, enabling the company to reinvest into innovation and growth.
Overall, the outlook for RPTK is positive, with expectations for sustained revenue and profitability growth. The company's focus on its core competencies, combined with its strategic expansion into new markets, positions it well for long-term success. The primary risk to this prediction is the increasing competition from other players in the food safety and supply chain management market. The development of any new technologies or disruptions in regulations will affect the company, especially if they fail to adapt accordingly. Another risk lies in the potential for economic downturns, which could affect businesses, impacting their capacity to invest in regulatory compliance solutions. However, RPTK's strong position, coupled with its adaptable business model, should allow it to mitigate these risks.
Rating | Short-Term | Long-Term Senior |
---|---|---|
Outlook | B2 | Ba3 |
Income Statement | Baa2 | Baa2 |
Balance Sheet | C | B3 |
Leverage Ratios | C | B3 |
Cash Flow | Caa2 | B1 |
Rates of Return and Profitability | Ba3 | B1 |
*Financial analysis is the process of evaluating a company's financial performance and position by neural network. It involves reviewing the company's financial statements, including the balance sheet, income statement, and cash flow statement, as well as other financial reports and documents.
How does neural network examine financial reports and understand financial state of the company?
References
- Z. Wang, T. Schaul, M. Hessel, H. van Hasselt, M. Lanctot, and N. de Freitas. Dueling network architectures for deep reinforcement learning. In Proceedings of the International Conference on Machine Learning (ICML), pages 1995–2003, 2016.
- G. Theocharous and A. Hallak. Lifetime value marketing using reinforcement learning. RLDM 2013, page 19, 2013
- Candès E, Tao T. 2007. The Dantzig selector: statistical estimation when p is much larger than n. Ann. Stat. 35:2313–51
- M. J. Hausknecht. Cooperation and Communication in Multiagent Deep Reinforcement Learning. PhD thesis, The University of Texas at Austin, 2016
- Sutton RS, Barto AG. 1998. Reinforcement Learning: An Introduction. Cambridge, MA: MIT Press
- Dietterich TG. 2000. Ensemble methods in machine learning. In Multiple Classifier Systems: First International Workshop, Cagliari, Italy, June 21–23, pp. 1–15. Berlin: Springer
- Chow, G. C. (1960), "Tests of equality between sets of coefficients in two linear regressions," Econometrica, 28, 591–605.