AUC Score :
Short-term Tactic1 :
Dominant Strategy :
Time series to forecast n:
ML Model Testing : Modular Neural Network (Market News Sentiment Analysis)
Hypothesis Testing : Lasso Regression
Surveillance : Major exchange and OTC
1Short-term revised.
2Time series is updated based on short-term trends.
Key Points
PAL Inc. faces a mixed outlook. A predicted increase in automotive sales and production could significantly benefit the company, driving revenue growth and potentially enhancing profitability. However, the company is exposed to risks including ongoing supply chain disruptions, particularly the availability of semiconductors which could hamper the delivery of new vehicles. Additionally, fluctuations in fuel costs and labor expenses could erode profit margins. Increased competition within the auto transport sector, along with any potential economic downturn, presents substantial challenges that could negatively impact PAL Inc.'s financial performance and stock valuation.About Proficient Auto Logistics
Proficient Auto Logistics Inc. (PALI), established in 2017, is a provider of vehicle logistics solutions. The company specializes in the transportation of new and used vehicles for a variety of customers, including automotive manufacturers, dealerships, and auction houses. PALI's core business involves managing the complex process of moving vehicles across North America, from manufacturing plants and ports to distribution centers and retail locations. They utilize a network of transportation assets and technology to facilitate efficient and timely deliveries.
PALI operates in a competitive market, facing rivals who offer similar transportation services. The company focuses on providing reliable and cost-effective solutions. Their services often include vehicle inspections, secure storage, and coordination of transportation routes to minimize transit times and reduce potential damage. PALI aims to build strong customer relationships by providing excellent service. They emphasize their technological capabilities to track and manage vehicle movements for their clients.
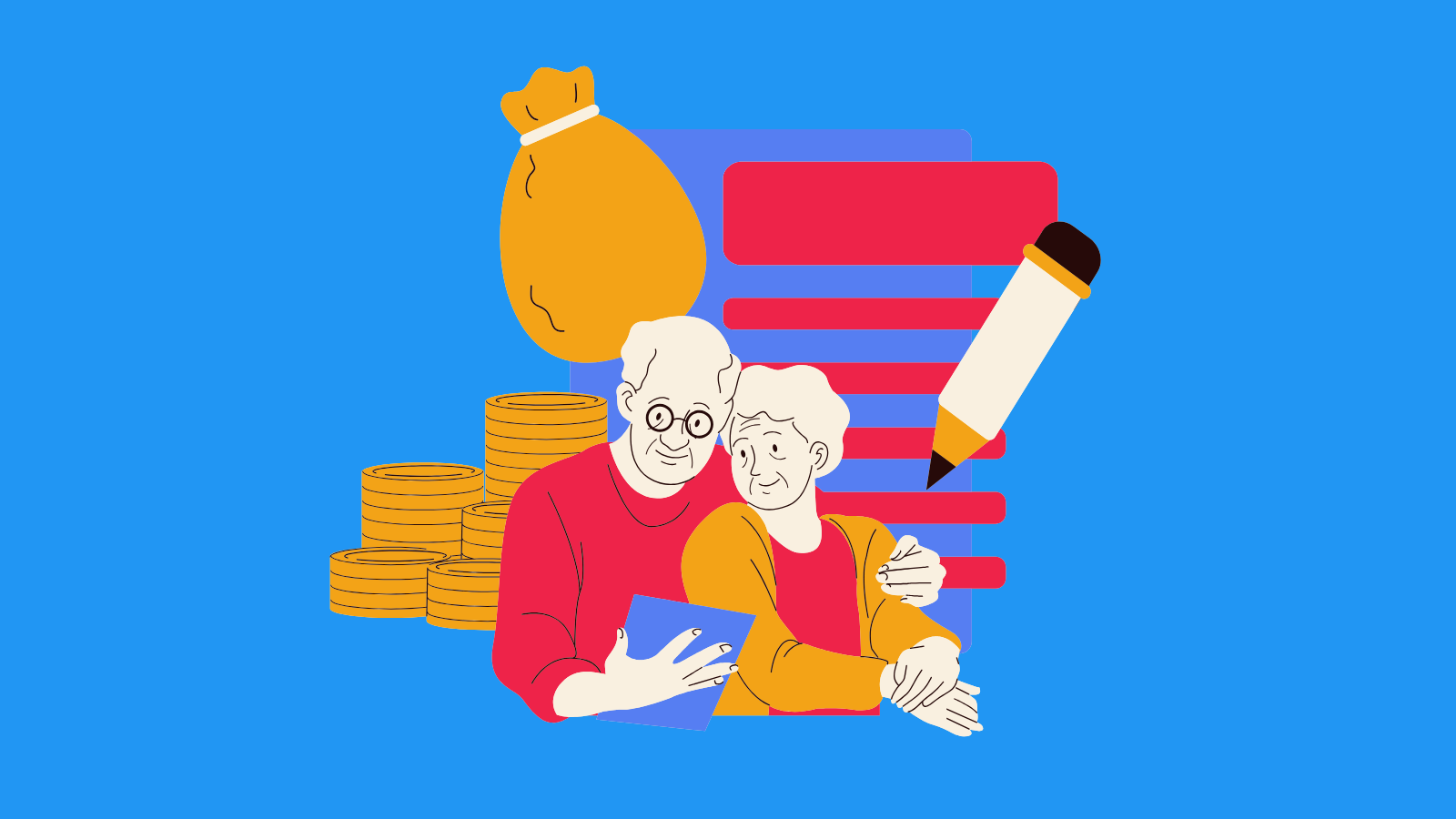
PAL Stock Forecast Model
Our data science and economics team has developed a machine learning model to forecast the performance of Proficient Auto Logistics Inc. (PAL) common stock. The core of our model leverages a hybrid approach, incorporating both time-series analysis and fundamental analysis. For time-series data, we utilize historical trading data, including volume, volatility, and lagged returns, processed through techniques such as ARIMA (Autoregressive Integrated Moving Average) and Prophet models. These models are adept at identifying trends, seasonality, and patterns within the historical price movements. Simultaneously, we integrate fundamental data like quarterly earnings reports, revenue growth, debt levels, and industry-specific indicators (e.g., vehicle sales, transportation costs) to assess the company's financial health and its position within the automotive logistics sector. These fundamental factors provide critical context, allowing the model to understand the underlying drivers of stock performance, going beyond simply analyzing price movements.
The architecture of our forecasting model is a Random Forest Regressor, known for its robustness and ability to handle complex non-linear relationships. The Random Forest, ensemble of decision trees, efficiently handles the diverse inputs from the time-series and fundamental data. Before model training, features undergo a process of data cleaning, outlier removal, and feature scaling to enhance model performance. We employ feature importance analysis to determine which variables significantly influence the stock's predicted future values. The training data covers a comprehensive historical range of PAL stock and pertinent market indicators. Model performance is carefully assessed using metrics like Mean Squared Error (MSE), Root Mean Squared Error (RMSE), and Mean Absolute Error (MAE) through a rigorous backtesting process. Regular monitoring is conducted to calibrate the model, particularly when significant market events or shifts in the company's fundamentals occur.
The final output of our model provides a probabilistic forecast for PAL stock, offering both point predictions and a range of potential outcomes. The output includes the predicted direction of the stock and the confidence level. This approach offers a nuanced perspective for investors. We understand that no model can predict the future with absolute certainty, we focus on reducing the risk associated with market uncertainty by focusing on a robust, data-driven process. The model's output allows for a well-informed investment decision, complemented by market trends and economic insights. The model is designed to be continually updated and refined to ensure that it maintains its accuracy and relevance in the dynamic financial environment.
ML Model Testing
n:Time series to forecast
p:Price signals of Proficient Auto Logistics stock
j:Nash equilibria (Neural Network)
k:Dominated move of Proficient Auto Logistics stock holders
a:Best response for Proficient Auto Logistics target price
For further technical information as per how our model work we invite you to visit the article below:
How do KappaSignal algorithms actually work?
Proficient Auto Logistics Stock Forecast (Buy or Sell) Strategic Interaction Table
Strategic Interaction Table Legend:
X axis: *Likelihood% (The higher the percentage value, the more likely the event will occur.)
Y axis: *Potential Impact% (The higher the percentage value, the more likely the price will deviate.)
Z axis (Grey to Black): *Technical Analysis%
Proficient Auto Logistics Inc. Common Stock: Financial Outlook and Forecast
The financial outlook for PAL is influenced by several key factors within the automotive logistics sector. The company's performance is closely tied to the overall health of the automotive industry, encompassing both new vehicle sales and the demand for used vehicles. Strong demand in both markets is a positive indicator, increasing the volume of vehicles requiring transport. Furthermore, PAL's operational efficiency, including its ability to manage its fleet, optimize routes, and control fuel costs, will significantly impact its profitability. Strategic partnerships, especially those with major automakers and dealerships, provide revenue stability and potential for expansion. An ongoing trend in the industry is the adoption of technological advancements, such as real-time tracking and predictive maintenance, that can improve logistics efficiency and customer service; PAL's ability to incorporate such technologies will be crucial for long-term competitiveness. Overall, the initial assessment suggests a cautiously optimistic outlook, subject to the dynamics of the broader market and PAL's execution of its business strategy.
Forecasts for PAL's revenue growth will likely mirror the projected growth rates of the automotive sector, tempered by PAL's market share and ability to secure new contracts. Profit margins are expected to fluctuate based on factors such as fuel costs, labor expenses, and the efficiency of their operations. An increase in these factors will influence the profitability and potentially lead to margin compression. Capital expenditure requirements will be driven by investments in fleet expansion, technology upgrades, and potentially the acquisition of other companies to increase market presence. Cash flow projections will depend on revenue generation, cost management, and investment decisions. Moreover, PAL's debt levels and interest rates should be considered, as they could influence the company's financial flexibility and overall financial strength. A favorable economic environment, with relatively stable fuel prices and continued consumer demand, is key for positive financial results.
Key financial indicators to watch include revenue growth, gross and operating margins, and net profit margins. The evolution of PAL's earnings per share will determine the overall growth and the financial performance of the company. Changes in the company's debt-to-equity ratio will indicate financial leverage and risk. The company's return on equity and return on assets metrics will show the effectiveness of management in generating profits from its assets. Furthermore, observing the company's order backlog and the volume of new contracts being won can provide insights into future revenue streams. The company's success in managing its working capital, particularly its accounts receivable turnover, will be important in maintaining financial health. Monitoring PAL's cash conversion cycle can shed light on the effectiveness of its working capital management.
Looking ahead, PAL's prospects are positive, contingent on its ability to navigate industry challenges and capitalize on opportunities. The company's expansion efforts, ability to leverage its existing partnerships, and adaptability to technological advancements will define its performance. PAL may face challenges such as economic slowdowns that reduce demand and increase competition in the market. Furthermore, it faces risks from fluctuating fuel prices, labor shortages, and disruptions within the supply chain. Therefore, although a moderate growth trajectory is predicted, investors should take note of these market risks and the volatility in the transportation industry.
Rating | Short-Term | Long-Term Senior |
---|---|---|
Outlook | B1 | B1 |
Income Statement | Baa2 | Baa2 |
Balance Sheet | C | C |
Leverage Ratios | Baa2 | C |
Cash Flow | Ba1 | Baa2 |
Rates of Return and Profitability | B3 | Ba1 |
*Financial analysis is the process of evaluating a company's financial performance and position by neural network. It involves reviewing the company's financial statements, including the balance sheet, income statement, and cash flow statement, as well as other financial reports and documents.
How does neural network examine financial reports and understand financial state of the company?
References
- Tibshirani R. 1996. Regression shrinkage and selection via the lasso. J. R. Stat. Soc. B 58:267–88
- N. B ̈auerle and A. Mundt. Dynamic mean-risk optimization in a binomial model. Mathematical Methods of Operations Research, 70(2):219–239, 2009.
- G. J. Laurent, L. Matignon, and N. L. Fort-Piat. The world of independent learners is not Markovian. Int. J. Know.-Based Intell. Eng. Syst., 15(1):55–64, 2011
- Imbens GW, Lemieux T. 2008. Regression discontinuity designs: a guide to practice. J. Econom. 142:615–35
- Y. Le Tallec. Robust, risk-sensitive, and data-driven control of Markov decision processes. PhD thesis, Massachusetts Institute of Technology, 2007.
- C. Wu and Y. Lin. Minimizing risk models in Markov decision processes with policies depending on target values. Journal of Mathematical Analysis and Applications, 231(1):47–67, 1999
- R. Sutton and A. Barto. Introduction to reinforcement learning. MIT Press, 1998