AUC Score :
Short-term Tactic1 :
Dominant Strategy :
Time series to forecast n:
ML Model Testing : Modular Neural Network (Speculative Sentiment Analysis)
Hypothesis Testing : Chi-Square
Surveillance : Major exchange and OTC
1Short-term revised.
2Time series is updated based on short-term trends.
Key Points
Based on current market trends and industry analysis, Parker-Hannifin Corporation (PH) is predicted to experience steady growth in the coming period, driven by increasing demand for its diversified products and services, particularly within the aerospace and industrial sectors. This growth is further supported by strategic acquisitions and a focus on innovation, which will enhance its market position. However, potential risks include economic slowdowns impacting industrial output and fluctuations in raw material costs, which could squeeze profit margins. Furthermore, intensified competition from both established and emerging players, alongside geopolitical uncertainties, may present challenges to PH's operational performance. Therefore, while PH exhibits a positive outlook, investors should closely monitor these factors to assess the long-term investment viability.About Parker-Hannifin Corporation
Parker-Hannifin (PH) is a global leader in motion and control technologies. The company provides engineered solutions for a wide array of mobile, industrial, and aerospace markets. These solutions include fluid power systems, electromechanical controls, filtration products, and sealing and shielding technologies. PH serves diverse industries, including aerospace, automotive, construction, agriculture, and material handling. The company's products are critical to the operation and performance of numerous applications across these sectors, contributing to efficiency, safety, and reliability.
With a focus on innovation and engineering excellence, Parker-Hannifin operates through a decentralized structure, with multiple business groups serving specific market segments. The company emphasizes its commitment to sustainability, quality, and customer satisfaction, continuously investing in research and development to meet evolving industry needs. PH's extensive global presence, coupled with its diverse product offerings, allows it to maintain a strong position in the market, delivering value to customers worldwide.
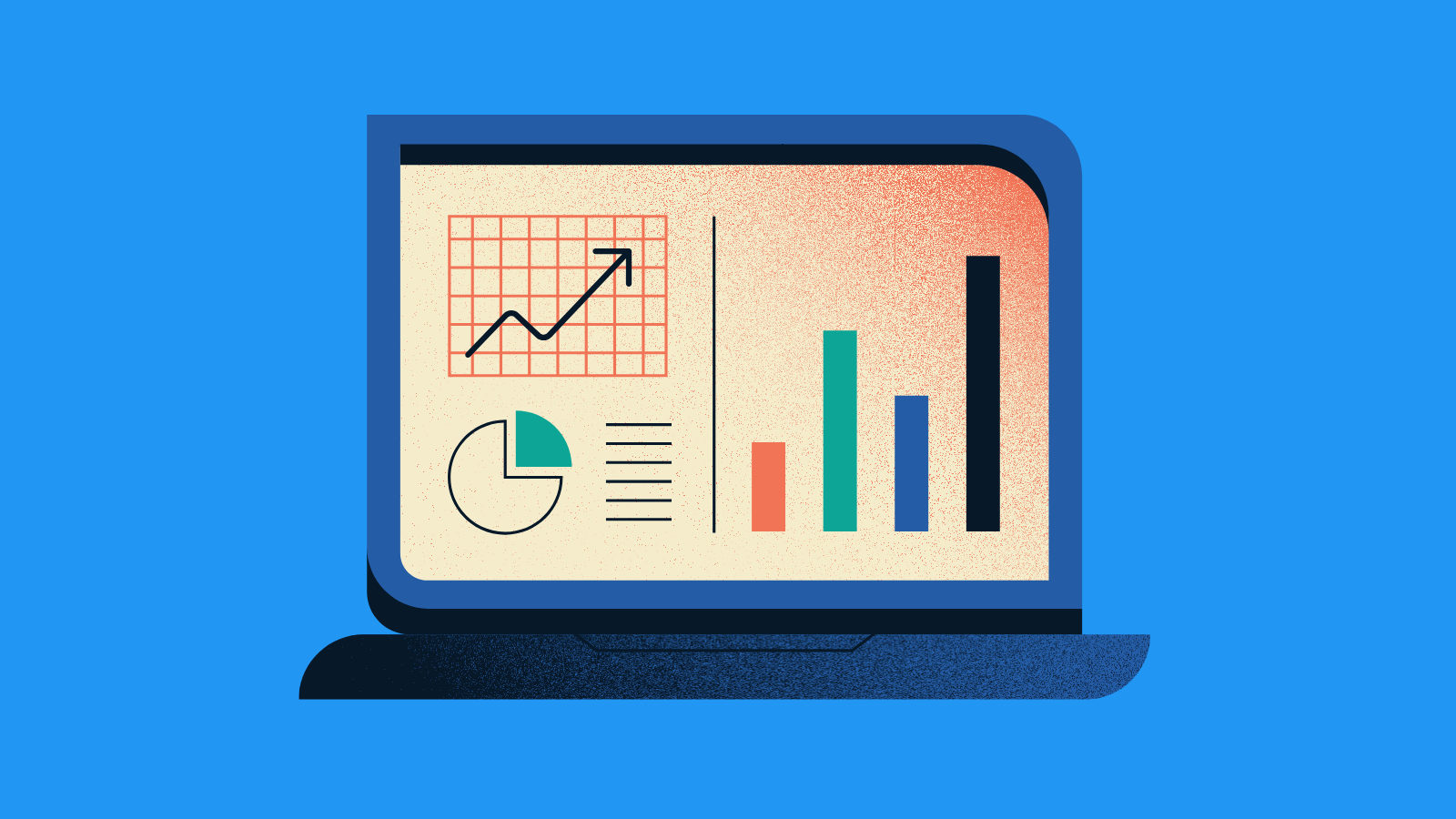
PH Stock Forecast Machine Learning Model
The objective is to develop a robust machine learning model to forecast the performance of Parker-Hannifin Corporation Common Stock (PH). Our approach integrates a comprehensive set of features derived from financial statements, market data, and macroeconomic indicators. Financial features will include, but not limited to, revenue, earnings per share (EPS), debt-to-equity ratio, and gross profit margin, extracted from quarterly and annual reports. Market data encompasses trading volume, volatility, and the stock's correlation with benchmark indices such as the S&P 500. Macroeconomic variables like the Consumer Price Index (CPI), unemployment rates, and industrial production indices will be incorporated to capture broader economic trends. Feature engineering will involve creating lagged variables, calculating rolling averages, and implementing ratio analysis to enhance model performance. The initial model selection will explore several algorithms, including Recurrent Neural Networks (RNNs), specifically Long Short-Term Memory (LSTM) networks, due to their proven capability in handling time-series data.
The model training and validation process will be rigorously executed. The dataset will be divided into training, validation, and test sets. The model will be trained on the training set, optimized using the validation set, and finally evaluated on the unseen test set. We will implement cross-validation techniques to ensure the model's generalization ability. Model performance will be assessed using relevant metrics such as Mean Squared Error (MSE), Root Mean Squared Error (RMSE), and Mean Absolute Error (MAE). Furthermore, we will analyze the model's output to identify key drivers of stock performance. This will entail conducting feature importance analysis to highlight the most influential variables. The parameters of the model will be continuously refined through hyperparameter tuning, using techniques such as grid search or Bayesian optimization to maximize predictive accuracy.
Post-modeling, we will perform thorough risk assessment and provide interpretability. We will analyze model outputs to identify potential biases and limitations, considering potential market events or economic shifts. We will construct confidence intervals and conduct sensitivity analysis to quantify the model's uncertainty. Interpretability techniques, such as SHAP (Shapley Additive Explanations) values, will be employed to gain deeper insights into the model's decision-making process, making the model explainable and increasing trust. Finally, we will regularly monitor and update the model with new data, performing retraining and recalibration, to maintain its accuracy and relevance in the dynamic financial landscape. This iterative approach is critical to sustaining high performance and adaptation to the changing market conditions impacting PH stock.
```
ML Model Testing
n:Time series to forecast
p:Price signals of Parker-Hannifin Corporation stock
j:Nash equilibria (Neural Network)
k:Dominated move of Parker-Hannifin Corporation stock holders
a:Best response for Parker-Hannifin Corporation target price
For further technical information as per how our model work we invite you to visit the article below:
How do KappaSignal algorithms actually work?
Parker-Hannifin Corporation Stock Forecast (Buy or Sell) Strategic Interaction Table
Strategic Interaction Table Legend:
X axis: *Likelihood% (The higher the percentage value, the more likely the event will occur.)
Y axis: *Potential Impact% (The higher the percentage value, the more likely the price will deviate.)
Z axis (Grey to Black): *Technical Analysis%
Rating | Short-Term | Long-Term Senior |
---|---|---|
Outlook | B3 | Ba2 |
Income Statement | B3 | Baa2 |
Balance Sheet | Caa2 | Baa2 |
Leverage Ratios | Caa2 | C |
Cash Flow | C | Baa2 |
Rates of Return and Profitability | Ba1 | B3 |
*Financial analysis is the process of evaluating a company's financial performance and position by neural network. It involves reviewing the company's financial statements, including the balance sheet, income statement, and cash flow statement, as well as other financial reports and documents.
How does neural network examine financial reports and understand financial state of the company?
References
- Künzel S, Sekhon J, Bickel P, Yu B. 2017. Meta-learners for estimating heterogeneous treatment effects using machine learning. arXiv:1706.03461 [math.ST]
- Angrist JD, Pischke JS. 2008. Mostly Harmless Econometrics: An Empiricist's Companion. Princeton, NJ: Princeton Univ. Press
- K. Boda and J. Filar. Time consistent dynamic risk measures. Mathematical Methods of Operations Research, 63(1):169–186, 2006
- Chamberlain G. 2000. Econometrics and decision theory. J. Econom. 95:255–83
- Breiman L. 1993. Better subset selection using the non-negative garotte. Tech. Rep., Univ. Calif., Berkeley
- N. B ̈auerle and A. Mundt. Dynamic mean-risk optimization in a binomial model. Mathematical Methods of Operations Research, 70(2):219–239, 2009.
- V. Borkar and R. Jain. Risk-constrained Markov decision processes. IEEE Transaction on Automatic Control, 2014