AUC Score :
Short-term Tactic1 :
Dominant Strategy :
Time series to forecast n:
ML Model Testing : Multi-Task Learning (ML)
Hypothesis Testing : Factor
Surveillance : Major exchange and OTC
1Short-term revised.
2Time series is updated based on short-term trends.
Key Points
NEE is projected to demonstrate continued growth, driven by its substantial renewable energy portfolio and strategic investments in infrastructure. Expansion into new markets and favorable regulatory environments supporting clean energy initiatives will likely boost revenue and earnings. However, the company faces risks including potential fluctuations in commodity prices, supply chain disruptions impacting project timelines, and increased competition within the renewable energy sector. Regulatory changes, especially those affecting subsidies or tax credits for renewable projects, could negatively impact profitability and growth. Increased interest rates might make new projects more expensive to finance, potentially slowing down expansion plans.About NextEra Energy
NextEra Energy, Inc. is a prominent American energy company primarily involved in the generation, transmission, and distribution of electricity. It is headquartered in Juno Beach, Florida, and operates through two primary subsidiaries: Florida Power & Light Company (FPL), one of the largest regulated electric utilities in the United States, and NextEra Energy Resources, a leading producer of renewable energy from wind and solar sources. The company's strategic focus is on clean energy and sustainable practices, with a significant portfolio of renewable energy projects across the country.
NextEra Energy is committed to innovation and technological advancements within the energy sector. The company has consistently invested in renewable energy infrastructure, seeking to reduce its carbon footprint and contribute to the transition towards a lower-carbon economy. Moreover, NextEra Energy is known for its strong financial performance and commitment to delivering value to its stakeholders, with a reputation as a leader in the utility industry.
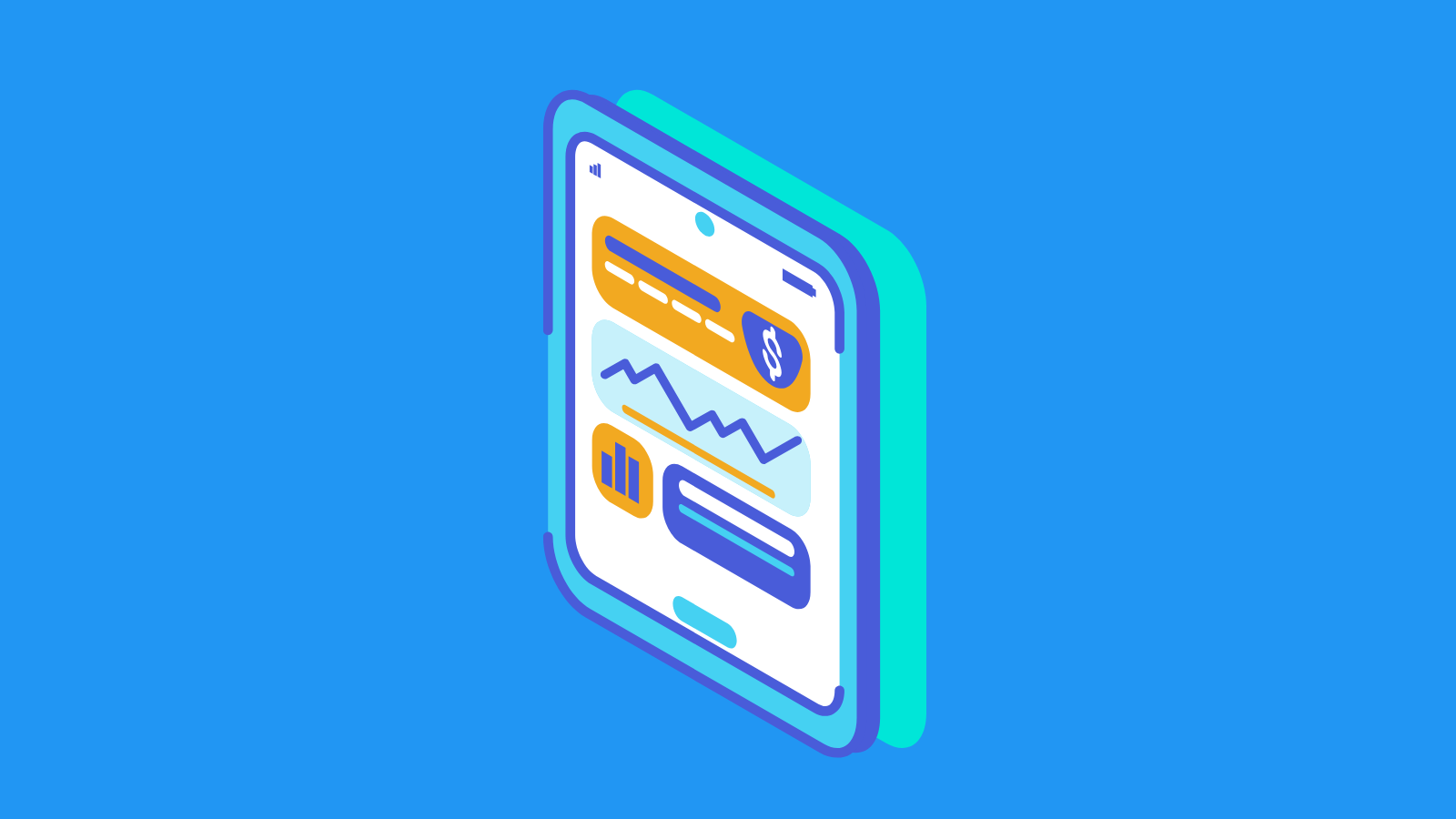
NEE Stock Forecast Machine Learning Model
Our team of data scientists and economists has developed a machine learning model to forecast the performance of NextEra Energy Inc. (NEE) common stock. This model incorporates a diverse range of financial and economic indicators to provide a comprehensive and data-driven prediction. We leverage historical NEE stock data, including daily and weekly trading volumes, closing prices, and volatility measures. Complementing this, we incorporate key economic indicators such as inflation rates, interest rates (specifically the yield on 10-year US Treasury notes), the unemployment rate, and the Consumer Price Index (CPI). Furthermore, we integrate industry-specific data, focusing on trends in renewable energy adoption, regulatory changes impacting the utility sector, and the competitive landscape among energy providers. Feature engineering plays a crucial role, including the creation of technical indicators such as moving averages, relative strength index (RSI), and Bollinger Bands to capture market sentiment and momentum.
The machine learning model utilizes a combination of algorithms to achieve robust and reliable forecasts. We primarily employ Gradient Boosting Machines (GBM) and Long Short-Term Memory (LSTM) networks due to their established effectiveness in financial time series analysis. GBMs excel at handling complex non-linear relationships within the data and are effective for capturing trends and patterns in our selected indicators. LSTMs, as a type of recurrent neural network, are particularly well-suited for capturing temporal dependencies and the sequential nature of stock data. The model is trained on a substantial historical dataset, with data up to the present. This model undergoes rigorous validation using techniques such as cross-validation and hold-out sets to assess its predictive accuracy and minimize the risk of overfitting. Model performance is evaluated using metrics such as Mean Absolute Error (MAE), Root Mean Squared Error (RMSE), and the direction of correct predictions to ensure model reliability.
The forecasting process involves feeding the current values of the economic and financial indicators, industry-specific data, and the derived technical indicators into the trained model. The model then generates a probability distribution, which provides a forecast for the NEE stock performance (e.g., upward or downward movement). This information is translated into actionable insights, supporting investment decisions, and providing insights into the potential risks and opportunities. The model's output is continuously monitored and recalibrated using new incoming data to adapt to changing market conditions and external factors. The model is designed as a dynamic system and incorporates feedback loops, allowing for continuous improvement to capture the nuances of the market, and is also adaptable to incorporate new factors that may arise.
ML Model Testing
n:Time series to forecast
p:Price signals of NextEra Energy stock
j:Nash equilibria (Neural Network)
k:Dominated move of NextEra Energy stock holders
a:Best response for NextEra Energy target price
For further technical information as per how our model work we invite you to visit the article below:
How do KappaSignal algorithms actually work?
NextEra Energy Stock Forecast (Buy or Sell) Strategic Interaction Table
Strategic Interaction Table Legend:
X axis: *Likelihood% (The higher the percentage value, the more likely the event will occur.)
Y axis: *Potential Impact% (The higher the percentage value, the more likely the price will deviate.)
Z axis (Grey to Black): *Technical Analysis%
NextEra Energy Inc. (NEE) Financial Outlook and Forecast
NextEra Energy (NEE) is positioned for continued growth driven by its robust renewable energy portfolio and regulated utility business, Florida Power & Light (FPL). The company's strategic focus on clean energy, including wind, solar, and battery storage, aligns with the global shift towards decarbonization and government initiatives supporting renewable energy development. FPL's consistent performance, supported by rate base expansion and operational efficiency, provides a stable earnings foundation. NEE's management team has a proven track record of executing its strategy, demonstrating strong financial discipline and effective capital allocation. The company's significant investment in renewable energy projects and related infrastructure is expected to drive earnings and cash flow growth over the coming years. Furthermore, the company's geographic diversification, particularly within the United States, reduces exposure to specific regional risks and provides greater flexibility in pursuing growth opportunities.
The company's financial performance is anticipated to be positive, with analysts projecting steady earnings growth and dividend increases. NEE's investment in renewable energy assets is expected to generate significant long-term value. The company's integrated business model, encompassing both generation and distribution, allows for greater control over its operations and cost management. Furthermore, NEE is likely to benefit from the ongoing decline in the cost of renewable energy technologies, which improves project economics. The company's ability to secure favorable financing terms and maintain a strong balance sheet will support its continued investment in growth projects. The regulated utility business, FPL, is expected to continue generating predictable cash flows and contribute to the overall financial stability of the parent company.
The company's growth strategy is centered on expanding its renewable energy capacity, enhancing its transmission and distribution infrastructure, and providing reliable, affordable energy to customers. NEE's commitment to research and development in areas such as energy storage and smart grids further enhances its competitive advantage. The company actively seeks opportunities to partner with governments and other utilities to deploy large-scale renewable energy projects. NEE's management has a strong emphasis on environmental sustainability and corporate social responsibility, further attracting investors and stakeholders. The company is also actively engaged in innovative solutions for grid modernization and resilience. These strategies are all expected to support the long-term financial performance of the company.
Based on these factors, the financial outlook for NEE is deemed positive. Continued investment in renewable energy, a strong regulated utility business, and effective capital management are expected to drive earnings growth and dividend increases. However, there are some potential risks. These risks include regulatory challenges, such as changes in tax or environmental policies, and the potential for delays or cost overruns in the development of large-scale projects. Competition from other energy companies and the impact of weather events on energy demand also could pose risks. Nevertheless, the company's diversified business model, strong financial position, and strategic focus on the growing renewable energy market position it well to navigate these risks and generate sustainable long-term value for its shareholders.
Rating | Short-Term | Long-Term Senior |
---|---|---|
Outlook | Ba2 | Baa2 |
Income Statement | Baa2 | Baa2 |
Balance Sheet | Baa2 | Baa2 |
Leverage Ratios | Ba3 | Baa2 |
Cash Flow | Caa2 | Caa2 |
Rates of Return and Profitability | Baa2 | Baa2 |
*Financial analysis is the process of evaluating a company's financial performance and position by neural network. It involves reviewing the company's financial statements, including the balance sheet, income statement, and cash flow statement, as well as other financial reports and documents.
How does neural network examine financial reports and understand financial state of the company?
References
- J. Harb and D. Precup. Investigating recurrence and eligibility traces in deep Q-networks. In Deep Reinforcement Learning Workshop, NIPS 2016, Barcelona, Spain, 2016.
- Krizhevsky A, Sutskever I, Hinton GE. 2012. Imagenet classification with deep convolutional neural networks. In Advances in Neural Information Processing Systems, Vol. 25, ed. Z Ghahramani, M Welling, C Cortes, ND Lawrence, KQ Weinberger, pp. 1097–105. San Diego, CA: Neural Inf. Process. Syst. Found.
- LeCun Y, Bengio Y, Hinton G. 2015. Deep learning. Nature 521:436–44
- Cortes C, Vapnik V. 1995. Support-vector networks. Mach. Learn. 20:273–97
- Lai TL, Robbins H. 1985. Asymptotically efficient adaptive allocation rules. Adv. Appl. Math. 6:4–22
- Chernozhukov V, Chetverikov D, Demirer M, Duflo E, Hansen C, Newey W. 2017. Double/debiased/ Neyman machine learning of treatment effects. Am. Econ. Rev. 107:261–65
- Ashley, R. (1988), "On the relative worth of recent macroeconomic forecasts," International Journal of Forecasting, 4, 363–376.