AUC Score :
Short-term Tactic1 :
Dominant Strategy :
Time series to forecast n:
ML Model Testing : Modular Neural Network (Financial Sentiment Analysis)
Hypothesis Testing : Linear Regression
Surveillance : Major exchange and OTC
1Short-term revised.
2Time series is updated based on short-term trends.
Key Points
LQR's future hinges on successfully navigating the competitive spirits market and scaling its operations. Predictions include potential revenue growth stemming from increased brand awareness and expanded distribution channels, alongside the risk of market saturation and evolving consumer preferences. Achieving profitability may be delayed if the company fails to control costs effectively and secure adequate funding for expansion. Moreover, regulatory changes impacting alcohol sales and distribution pose a significant threat to LQR's business model, along with the risk of negative publicity impacting brand reputation and sales. Success will depend on the company's ability to adapt to market dynamics and execute its growth strategy effectively.About LQR House
LQR House Inc. is a company focused on the alcoholic beverage industry. The firm operates primarily as a technology-driven platform, facilitating the distribution and sale of premium alcoholic beverages directly to consumers and businesses. LQR House leverages digital channels and e-commerce solutions to connect producers with retailers and end-users, streamlining the supply chain and expanding market reach. They often emphasize providing curated selections of high-end spirits, wines, and other alcoholic products, targeting consumers seeking specialized and exclusive offerings.
The company aims to disrupt traditional alcohol distribution models by implementing innovative technological capabilities and data analytics. LQR House emphasizes its ability to improve the customer experience. It also focuses on building strong relationships with both suppliers and consumers. The company's operations typically involve managing inventory, processing orders, and handling logistics related to the distribution of alcoholic beverages across various jurisdictions, complying with relevant regulatory requirements that are specific to the alcohol industry.
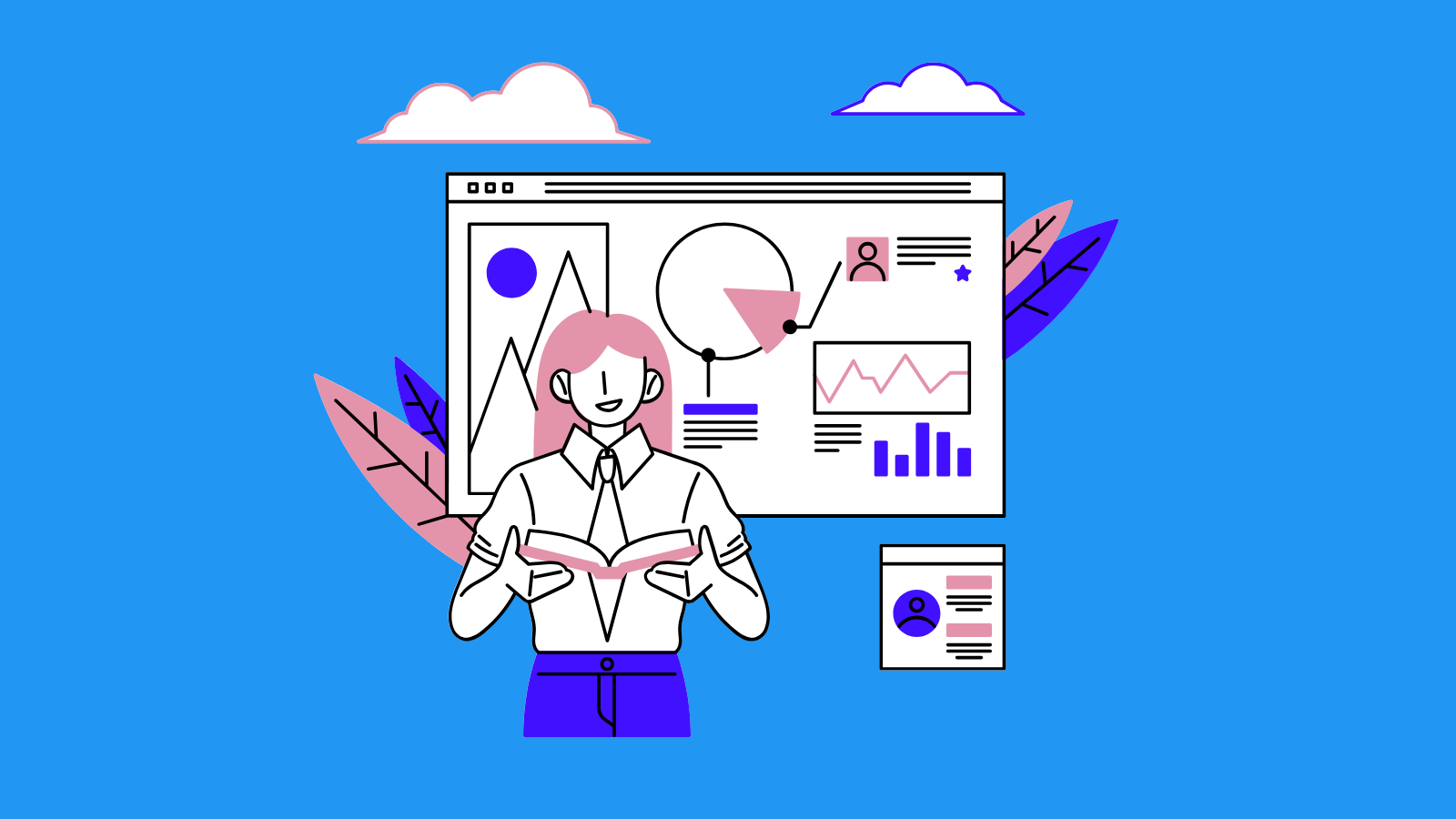
YHC Stock Forecasting: A Machine Learning and Econometrics Model
Our data science and economics team proposes a comprehensive machine learning model for forecasting LQR House Inc. (YHC) common stock performance. The model will leverage a combination of technical indicators, fundamental data, and macroeconomic variables. Technical indicators will include moving averages (SMA, EMA), Relative Strength Index (RSI), Moving Average Convergence Divergence (MACD), and Bollinger Bands to capture price trends and volatility. Fundamental data such as revenue, earnings per share (EPS), debt-to-equity ratio, and price-to-earnings ratio (P/E) will be incorporated to assess the company's financial health and valuation. We will also consider macroeconomic indicators like GDP growth, inflation rates, interest rates, and consumer confidence to gauge the overall economic environment's impact on the stock's performance.
The core of the model will be a hybrid approach, combining time series analysis with machine learning algorithms. We will employ an ensemble approach, testing and comparing the performance of several algorithms, including Recurrent Neural Networks (RNNs), specifically LSTMs (Long Short-Term Memory), for capturing time-dependent patterns in the stock price data, as well as Gradient Boosting algorithms such as XGBoost, which can handle complex relationships and non-linear patterns. A crucial component involves careful feature engineering. We will transform raw data into meaningful features by constructing lagged variables, ratio features, and interaction terms to improve model accuracy and interpretability. The model's performance will be rigorously validated using techniques such as cross-validation and backtesting, with evaluation metrics like Mean Squared Error (MSE) and R-squared to measure the prediction accuracy and compare against a benchmark.
To enhance the model's robustness, we will incorporate economic insights and risk management strategies. Econometric techniques will be used to identify and quantify the impact of economic factors on YHC's stock returns, ensuring the model considers the impact of macroeconomic conditions. The model output will include probability distributions and confidence intervals, allowing for risk assessment and the construction of trading signals with associated levels of uncertainty. Furthermore, the model will be continuously monitored and updated with fresh data to maintain its forecasting accuracy. We will create a system for model retraining and parameter tuning to adapt to any change in market dynamics. This continuous improvement process ensures the model's ongoing effectiveness for forecasting and optimizing investment strategies for YHC stock.
ML Model Testing
n:Time series to forecast
p:Price signals of LQR House stock
j:Nash equilibria (Neural Network)
k:Dominated move of LQR House stock holders
a:Best response for LQR House target price
For further technical information as per how our model work we invite you to visit the article below:
How do KappaSignal algorithms actually work?
LQR House Stock Forecast (Buy or Sell) Strategic Interaction Table
Strategic Interaction Table Legend:
X axis: *Likelihood% (The higher the percentage value, the more likely the event will occur.)
Y axis: *Potential Impact% (The higher the percentage value, the more likely the price will deviate.)
Z axis (Grey to Black): *Technical Analysis%
LQR House Inc. Common Stock: Financial Outlook and Forecast
LQR House (LQRH) operates within the alcoholic beverage e-commerce sector, a market exhibiting consistent growth fueled by evolving consumer preferences and the convenience of online purchasing. The company's business model centers on facilitating the online sale of spirits, wines, and beers, primarily through its proprietary platforms and partnerships. A key aspect of its outlook is tied to the expansion of its product offerings, including higher-margin premium and luxury alcohol brands. LQR House is actively pursuing strategic partnerships with established brands and distributors to increase its product catalog and reach a broader customer base. Additionally, the company is focused on enhancing its digital presence and marketing strategies to improve customer acquisition and retention rates. This encompasses investments in website optimization, targeted advertising campaigns, and leveraging social media platforms to drive sales growth.
The financial forecast for LQRH depends significantly on several key factors. Firstly, the overall growth trajectory of the e-commerce alcohol market is crucial. Any slowdown in this sector would directly impact the company's revenue growth. Secondly, the effectiveness of LQR House's marketing and sales initiatives will determine its ability to attract new customers and increase the frequency of repeat purchases. The company's ability to manage operating expenses, including fulfillment costs and marketing spend, will significantly influence its profitability. Furthermore, the company's ability to secure favorable terms with suppliers and maintain a healthy inventory management system will contribute to its financial stability. The forecast must account for the ongoing competitive landscape, including established players in the alcohol e-commerce market and emerging competitors.
Analyzing publicly available information provides a limited glimpse into LQRH's financials, considering it's a relatively new entrant in the public markets. Investors should monitor the company's quarterly and annual financial reports for significant performance changes. Key metrics to watch include revenue growth, gross profit margins, operating expenses, and customer acquisition cost. The company's balance sheet, particularly its cash position and debt levels, is important to assess its financial health. Tracking market trends in the e-commerce sector, consumer behavior, and regulatory changes related to alcohol sales are also vital. Research reports from financial analysts, if available, can offer additional insights into the company's performance and outlook. It is important to review all of this information over a period of time to make an informed investment decision.
Based on current market dynamics and LQR House's stated strategies, a positive outlook is possible. The e-commerce alcohol market's continued growth should provide a tailwind for the company's expansion. The success hinges on LQRH executing its growth plan, managing expenses, and securing strategic partnerships. However, several risks exist. Intense competition in the e-commerce market could erode profit margins or hamper revenue growth. Changes in regulations regarding alcohol sales could disrupt operations. Economic downturns could reduce consumer spending on discretionary items like alcohol, impacting sales. Investors should conduct thorough due diligence and consider the inherent risks before making an investment decision.
```
Rating | Short-Term | Long-Term Senior |
---|---|---|
Outlook | B1 | B2 |
Income Statement | Baa2 | C |
Balance Sheet | B2 | C |
Leverage Ratios | C | Caa2 |
Cash Flow | B1 | B1 |
Rates of Return and Profitability | B2 | Ba3 |
*Financial analysis is the process of evaluating a company's financial performance and position by neural network. It involves reviewing the company's financial statements, including the balance sheet, income statement, and cash flow statement, as well as other financial reports and documents.
How does neural network examine financial reports and understand financial state of the company?
References
- Matzkin RL. 1994. Restrictions of economic theory in nonparametric methods. In Handbook of Econometrics, Vol. 4, ed. R Engle, D McFadden, pp. 2523–58. Amsterdam: Elsevier
- Zubizarreta JR. 2015. Stable weights that balance covariates for estimation with incomplete outcome data. J. Am. Stat. Assoc. 110:910–22
- R. Sutton, D. McAllester, S. Singh, and Y. Mansour. Policy gradient methods for reinforcement learning with function approximation. In Proceedings of Advances in Neural Information Processing Systems 12, pages 1057–1063, 2000
- Christou, C., P. A. V. B. Swamy G. S. Tavlas (1996), "Modelling optimal strategies for the allocation of wealth in multicurrency investments," International Journal of Forecasting, 12, 483–493.
- G. J. Laurent, L. Matignon, and N. L. Fort-Piat. The world of independent learners is not Markovian. Int. J. Know.-Based Intell. Eng. Syst., 15(1):55–64, 2011
- M. Petrik and D. Subramanian. An approximate solution method for large risk-averse Markov decision processes. In Proceedings of the 28th International Conference on Uncertainty in Artificial Intelligence, 2012.
- Zou H, Hastie T. 2005. Regularization and variable selection via the elastic net. J. R. Stat. Soc. B 67:301–20