AUC Score :
Short-term Tactic1 :
Dominant Strategy :
Time series to forecast n:
ML Model Testing : Modular Neural Network (Market Volatility Analysis)
Hypothesis Testing : Linear Regression
Surveillance : Major exchange and OTC
1Short-term revised.
2Time series is updated based on short-term trends.
Key Points
Ligand's near term performance is likely to experience moderate volatility due to evolving partnerships and drug development pipelines. The company may see modest revenue growth as new partnerships solidify and existing collaborations advance towards clinical milestones. There is a possibility of increased market sentiment should Ligand secure successful regulatory approvals for its partnered products. However, risks exist, especially concerning dependency on partners for commercialization and success of novel drug candidates. Further risk is present around the impact of potential clinical trial failures or delays, which could significantly affect investor confidence and stock valuation. In addition, the company remains exposed to ongoing shifts in the pharmaceutical landscape.About Ligand Pharmaceuticals
Ligand Pharmaceuticals (LGND) is a biotechnology company focused on discovering and developing innovative medicines. It employs a business model centered on collaborations, partnering its drug discovery platforms and technologies with other pharmaceutical and biotechnology companies. LGND does not typically develop or market its own drugs directly, instead, it licenses its technologies and intellectual property to partners who then take responsibility for clinical development, regulatory approval, and commercialization. This approach allows LGND to diversify its portfolio and mitigate the risks associated with drug development.
The company's core technologies include Captisol, a solubilizing agent used to improve the solubility and bioavailability of drugs, and OmniAb, a platform for generating fully human monoclonal antibodies. LGND generates revenue through royalties, milestone payments, and upfront payments from its partners. Their strategy prioritizes the creation of value for its shareholders by fostering collaborations and creating a portfolio of royalty streams from successful drug products.
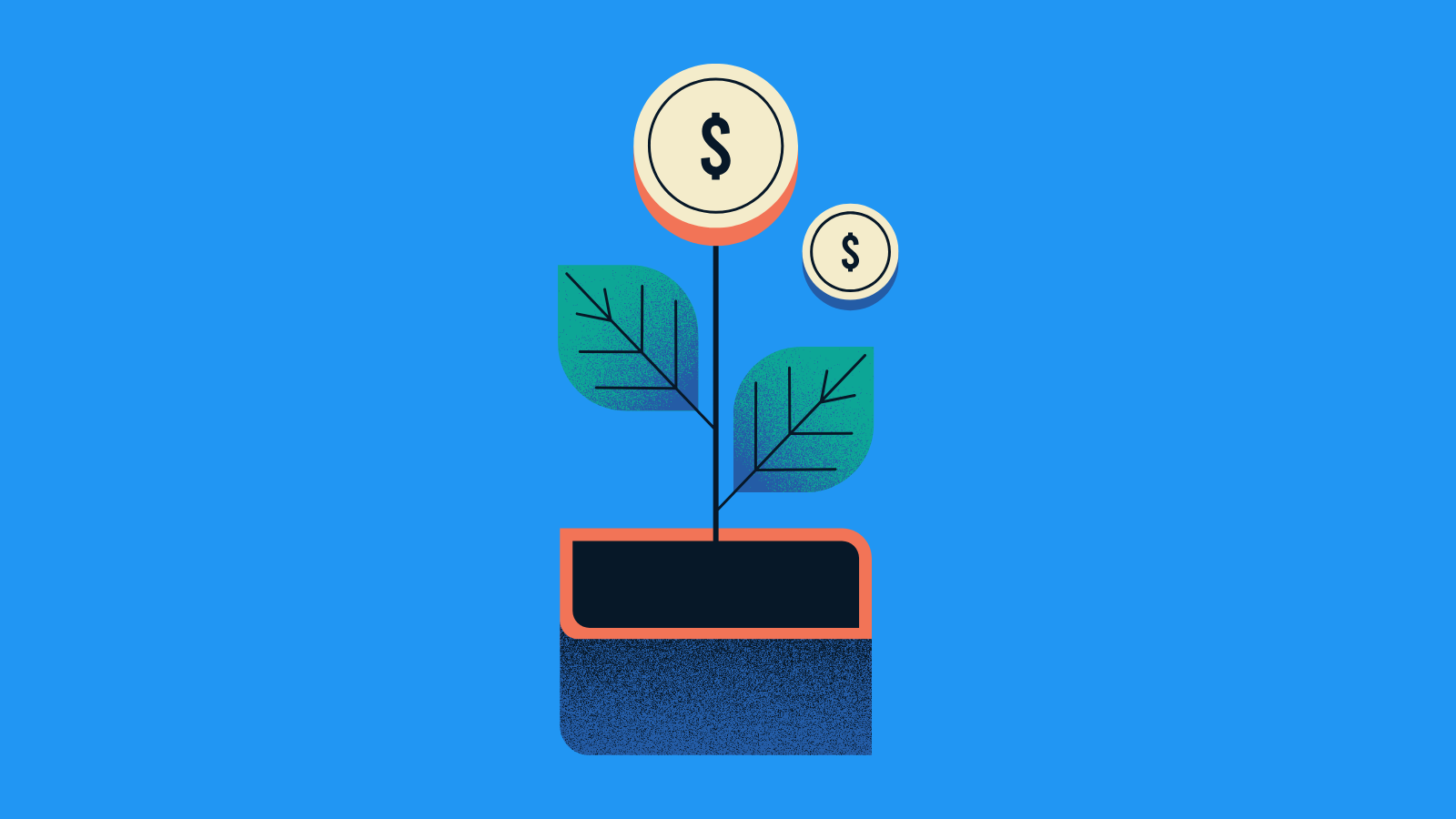
Machine Learning Model for LGND Stock Forecast
Our team of data scientists and economists proposes a comprehensive machine learning model for forecasting Ligand Pharmaceuticals Incorporated (LGND) stock performance. The core of our model centers around a hybrid approach that integrates diverse data sources. We will leverage a combination of time-series data, fundamental analysis, and sentiment analysis. Time-series data will incorporate historical stock prices, trading volumes, and technical indicators such as moving averages and Relative Strength Index (RSI). Fundamental data will encompass financial statements, including revenue, earnings per share (EPS), and debt-to-equity ratios, as well as industry-specific metrics. Furthermore, we will incorporate sentiment analysis, processing news articles, social media feeds, and financial reports to gauge market sentiment toward LGND. This multi-faceted approach aims to capture both the internal dynamics of the company and the external factors influencing its stock price.
The model architecture will employ an ensemble of machine learning algorithms to enhance predictive accuracy and robustness. We will initially experiment with a variety of algorithms, including Recurrent Neural Networks (RNNs) such as Long Short-Term Memory (LSTM) models, to capture temporal dependencies within the time-series data. Support Vector Machines (SVMs) will be utilized for their ability to handle high-dimensional data, and Random Forests to capture complex non-linear relationships between features and target variable. The ensemble will then be created by combining the predictions of these individual models, potentially weighting each model based on its historical performance. Feature engineering will be crucial, with careful selection and transformation of variables to optimize model performance. This includes the application of feature scaling techniques to standardize data ranges.
Model evaluation will employ rigorous methodologies. We will utilize a combination of statistical metrics, including Mean Absolute Error (MAE), Mean Squared Error (MSE), and Root Mean Squared Error (RMSE), to assess the accuracy of the model. In addition, we will monitor the model's performance over out-of-sample datasets, split into training, validation, and testing sets, using a rolling window approach. Backtesting will be performed to assess the model's performance in various market conditions. Regular model retraining will be implemented to adapt to evolving market dynamics and incorporate the latest data. Finally, we will conduct a sensitivity analysis to identify the most influential factors impacting the LGND stock forecast. The resulting model will provide a robust and data-driven tool for forecasting LGND's stock performance, aiding in informed investment decisions.
```
ML Model Testing
n:Time series to forecast
p:Price signals of Ligand Pharmaceuticals stock
j:Nash equilibria (Neural Network)
k:Dominated move of Ligand Pharmaceuticals stock holders
a:Best response for Ligand Pharmaceuticals target price
For further technical information as per how our model work we invite you to visit the article below:
How do KappaSignal algorithms actually work?
Ligand Pharmaceuticals Stock Forecast (Buy or Sell) Strategic Interaction Table
Strategic Interaction Table Legend:
X axis: *Likelihood% (The higher the percentage value, the more likely the event will occur.)
Y axis: *Potential Impact% (The higher the percentage value, the more likely the price will deviate.)
Z axis (Grey to Black): *Technical Analysis%
Ligand Pharmaceuticals Incorporated (LGND): Financial Outlook and Forecast
LGND, a company focused on developing and acquiring technologies that support the discovery of new medicines, currently faces a landscape marked by both opportunities and challenges. The company's financial outlook is primarily driven by its robust royalty streams from various partnered drugs and its continued investment in its Captisol technology. This platform, a key component of many approved and in-development pharmaceuticals, provides a stable source of revenue and future growth potential. LGND's strategy of licensing its technology to other pharmaceutical companies offers a less capital-intensive business model, allowing for diversification and reduced risk compared to full-scale drug development. Management's focus on managing operating expenses and allocating capital efficiently will be crucial for sustaining profitability and maximizing shareholder value.
The financial forecast for LGND appears relatively positive in the near to medium term. Analysts anticipate continued revenue growth, primarily stemming from royalty payments associated with successful drugs that incorporate Captisol. The company is also expected to benefit from the ongoing commercial success of several partnered products. Future revenue streams are poised to increase from new partnerships and expansion of existing ones. Furthermore, LGND's existing cash position is healthy, providing the resources to invest in research and development, pursue strategic acquisitions, or return capital to shareholders through dividends or share repurchases. Focus on innovative technologies and strategic partnerships is expected to provide a strong foundation for long-term growth.
Several factors warrant close attention when evaluating LGND's future prospects. The dependence on royalty streams, while providing stability, also introduces risk. The performance of LGND's partners' products directly impacts royalty revenues, making the company susceptible to fluctuations in the pharmaceutical market. Additionally, the company's ability to secure new partnerships and expand the use of Captisol technology is critical to long-term growth. Any delays or failures in the regulatory approval process for Captisol-based drugs could significantly affect future earnings. Furthermore, competition in the pharmaceutical industry is fierce, and LGND must continually innovate and adapt to remain competitive. The company's success will depend on its capacity to execute its strategic plan, maintain strong relationships with its partners, and efficiently manage its capital.
Overall, the financial outlook for LGND is positive. The company's strong royalty streams, Captisol technology, and strategic partnerships position it well for continued revenue growth and profitability. While the pharmaceutical industry inherently involves risk, and LGND faces the potential challenges associated with partner performance and regulatory hurdles, the current forecast suggests a continuation of the company's positive trajectory. However, investors should carefully monitor the performance of partner products, the progress of Captisol-related drug approvals, and any shifts in the competitive landscape.
```
Rating | Short-Term | Long-Term Senior |
---|---|---|
Outlook | B2 | B2 |
Income Statement | Caa2 | B3 |
Balance Sheet | Ba1 | C |
Leverage Ratios | B2 | Ba3 |
Cash Flow | Ba2 | B1 |
Rates of Return and Profitability | C | Caa2 |
*Financial analysis is the process of evaluating a company's financial performance and position by neural network. It involves reviewing the company's financial statements, including the balance sheet, income statement, and cash flow statement, as well as other financial reports and documents.
How does neural network examine financial reports and understand financial state of the company?
References
- L. Panait and S. Luke. Cooperative multi-agent learning: The state of the art. Autonomous Agents and Multi-Agent Systems, 11(3):387–434, 2005.
- Canova, F. B. E. Hansen (1995), "Are seasonal patterns constant over time? A test for seasonal stability," Journal of Business and Economic Statistics, 13, 237–252.
- Chamberlain G. 2000. Econometrics and decision theory. J. Econom. 95:255–83
- Abadie A, Imbens GW. 2011. Bias-corrected matching estimators for average treatment effects. J. Bus. Econ. Stat. 29:1–11
- Künzel S, Sekhon J, Bickel P, Yu B. 2017. Meta-learners for estimating heterogeneous treatment effects using machine learning. arXiv:1706.03461 [math.ST]
- J. G. Schneider, W. Wong, A. W. Moore, and M. A. Riedmiller. Distributed value functions. In Proceedings of the Sixteenth International Conference on Machine Learning (ICML 1999), Bled, Slovenia, June 27 - 30, 1999, pages 371–378, 1999.
- Hastie T, Tibshirani R, Tibshirani RJ. 2017. Extended comparisons of best subset selection, forward stepwise selection, and the lasso. arXiv:1707.08692 [stat.ME]