AUC Score :
Short-term Tactic1 :
Dominant Strategy :
Time series to forecast n:
ML Model Testing : Modular Neural Network (Market Direction Analysis)
Hypothesis Testing : Multiple Regression
Surveillance : Major exchange and OTC
1Short-term revised.
2Time series is updated based on short-term trends.
Key Points
The KOSPI index is expected to experience moderate growth, driven by a stabilization in global semiconductor demand and resilient domestic consumption, leading to incremental gains. However, this positive outlook faces risks, primarily stemming from potential inflationary pressures and further interest rate hikes by the Bank of Korea, which could dampen investor sentiment and slow economic expansion. Geopolitical instability, particularly in the region, along with uncertainties surrounding Chinese economic performance, could also trigger volatility and negatively impact the index's performance. Furthermore, significant shifts in the value of the Korean won against major currencies pose another risk, potentially affecting earnings of export-oriented companies and overall market confidence.About KOSPI Index
The KOSPI, or the Korea Composite Stock Price Index, is the primary stock market index of South Korea. It serves as a benchmark for the performance of the South Korean stock market, reflecting the overall economic health and investment sentiment within the country. This index tracks the performance of all listed companies on the Korea Exchange (KRX), providing a comprehensive overview of the market.
The KOSPI's fluctuations are influenced by a multitude of factors, including global economic trends, domestic policies, and corporate earnings. Investors and analysts closely monitor the KOSPI to gauge market trends, assess investment opportunities, and make informed decisions. Its movements can signal shifts in consumer confidence, industrial output, and the overall economic climate of South Korea, making it a crucial indicator for both domestic and international stakeholders.
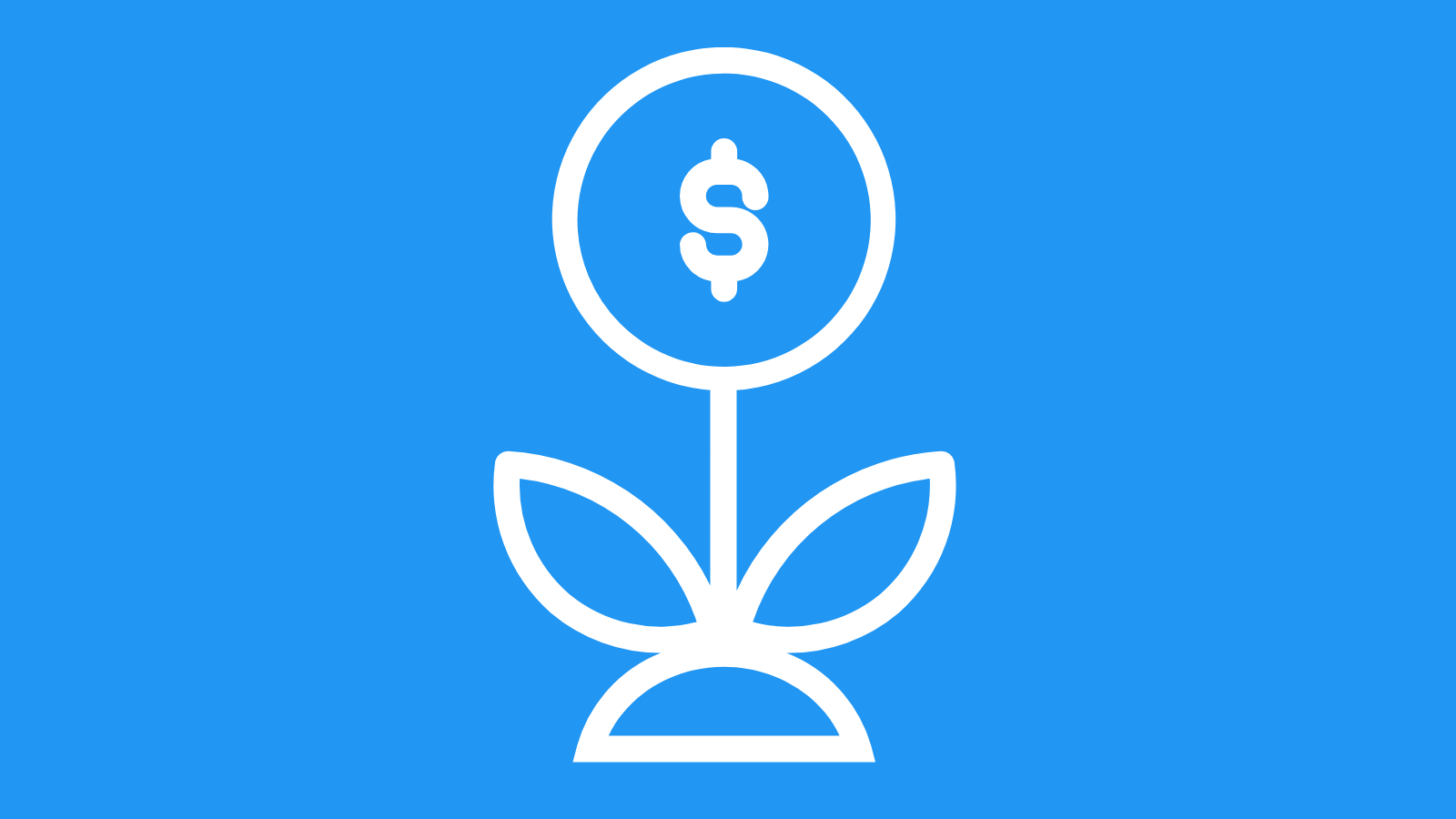
KOSPI Index Forecasting Model
Our team of data scientists and economists has developed a sophisticated machine learning model for forecasting the KOSPI index. This model leverages a comprehensive dataset encompassing various economic indicators, market sentiment data, and historical price movements. We have incorporated macroeconomic variables like GDP growth, inflation rates, interest rate changes, and exchange rate fluctuations, recognizing their significant influence on the Korean stock market. Additionally, the model integrates market-specific factors such as trading volumes, volatility indices (VIX), and industry-specific performance metrics. To gauge investor sentiment, we incorporate data from news articles, social media sentiment analysis, and option market activity. The historical price data serves as a crucial input, enabling the model to learn patterns and trends over time, capturing cyclical behavior and any persistence effects within the KOSPI.
The model utilizes a hybrid approach combining the strengths of different machine learning algorithms. We employed a combination of time series models (like ARIMA and its variations) to capture linear dependencies and trends in historical data. We have implemented various ensemble methods, such as Gradient Boosting and Random Forests, to address nonlinear relationships between the features and the KOSPI index. These algorithms can capture complex patterns and interactions within the dataset, leading to improved predictive accuracy. To further refine the model, we incorporated deep learning techniques, specifically Recurrent Neural Networks (RNNs), and its variants like LSTMs, which are particularly adept at analyzing sequential data, enabling us to model the temporal dependencies in the market.
The model's performance is rigorously evaluated using a variety of metrics, including Mean Squared Error (MSE), Root Mean Squared Error (RMSE), and Mean Absolute Error (MAE). We employed rigorous cross-validation techniques to ensure the model's robustness and generalizability to out-of-sample data. The model's output will include both point forecasts and confidence intervals, providing a range of possible future values and enabling risk assessment. Regular model updates, incorporating the latest economic data and market developments, are essential to maintain accuracy and relevance. The model undergoes ongoing monitoring and adjustment to minimize the risk of overfitting, ensuring that it provides consistent and reliable forecasts. Continuous feedback is obtained to improve its performance and adapt to changing market dynamics.
```ML Model Testing
n:Time series to forecast
p:Price signals of KOSPI index
j:Nash equilibria (Neural Network)
k:Dominated move of KOSPI index holders
a:Best response for KOSPI target price
For further technical information as per how our model work we invite you to visit the article below:
How do KappaSignal algorithms actually work?
KOSPI Index Forecast Strategic Interaction Table
Strategic Interaction Table Legend:
X axis: *Likelihood% (The higher the percentage value, the more likely the event will occur.)
Y axis: *Potential Impact% (The higher the percentage value, the more likely the price will deviate.)
Z axis (Grey to Black): *Technical Analysis%
KOSPI Index: Financial Outlook and Forecast
The South Korean stock market, represented by the KOSPI index, is currently navigating a complex global economic landscape, influencing its financial outlook. The Korean economy is significantly export-driven, making it highly susceptible to fluctuations in international trade and global demand. Recent trends suggest a moderate growth trajectory, influenced by factors such as semiconductor demand, technology innovation, and government policies. The performance of key sectors like technology, manufacturing, and automobiles will be crucial in determining the overall direction of the index. Domestic consumption, while showing signs of recovery, remains a factor, and its sustained growth is vital for a robust economic expansion. Furthermore, interest rate decisions by both the Bank of Korea and the US Federal Reserve exert considerable influence on investor sentiment and capital flows.
Several key macroeconomic indicators provide insight into the potential future performance of KOSPI. Inflation rates, though moderated in recent months, remain a concern, dictating the central bank's monetary policy stance. The manufacturing Purchasing Managers' Index (PMI) offers a barometer of activity within the industrial sector, and its movements can indicate the overall health of the economy. Corporate earnings reports from leading companies will also be critical, offering signals of profitability and future growth potential. Furthermore, geopolitical developments, including trade tensions and regional conflicts, can exert significant pressure on the stock market. The government's fiscal policies, encompassing tax incentives and infrastructure spending, may also provide impetus for growth within particular sectors. Investor sentiment, influenced by global economic conditions and market valuations, is another important factor to consider.
The technological sector, including the semiconductor industry, is a significant contributor to the KOSPI's performance. Demand for semiconductors, driven by trends like artificial intelligence (AI) and data centres, can have a substantial effect on the index. Automobile manufacturing, another prominent sector, is also influenced by consumer demand and global supply chains. Any shift in these variables will be of critical importance to the stock market. Government initiatives promoting innovation and supporting strategic industries will shape the overall investment environment, and the regulatory environment and policy changes can affect the market dynamics. Foreign investment flows, influenced by both economic conditions and currency valuations, will also have a substantial effect on the KOSPI's performance.
Overall, the outlook for the KOSPI index leans towards cautiously optimistic with a moderately positive outlook over the next 12-18 months. This prediction is based on the expectation of a gradual global economic recovery and continued growth in key sectors like technology and manufacturing. However, several risks could impact this forecast. These include an escalation in geopolitical tensions, a more severe-than-expected global economic slowdown, and unexpected rises in inflation. Potential risks include abrupt changes in government policies or regulations, as well as unforeseen disruptions in global supply chains that might impact the South Korean economy's exports. The possibility of faster-than-anticipated interest rate hikes by major central banks represents another significant downside risk that could hurt investor sentiment and market performance. Therefore, investors should approach the market with prudence and monitor the economic indicators.
```
Rating | Short-Term | Long-Term Senior |
---|---|---|
Outlook | B1 | Ba3 |
Income Statement | C | Baa2 |
Balance Sheet | C | B3 |
Leverage Ratios | Baa2 | B1 |
Cash Flow | Baa2 | Caa2 |
Rates of Return and Profitability | Ba3 | Baa2 |
*An aggregate rating for an index summarizes the overall sentiment towards the companies it includes. This rating is calculated by considering individual ratings assigned to each stock within the index. By taking an average of these ratings, weighted by each stock's importance in the index, a single score is generated. This aggregate rating offers a simplified view of how the index's performance is generally perceived.
How does neural network examine financial reports and understand financial state of the company?
References
- Athey S, Imbens GW. 2017a. The econometrics of randomized experiments. In Handbook of Economic Field Experiments, Vol. 1, ed. E Duflo, A Banerjee, pp. 73–140. Amsterdam: Elsevier
- Breusch, T. S. A. R. Pagan (1979), "A simple test for heteroskedasticity and random coefficient variation," Econometrica, 47, 1287–1294.
- Li L, Chen S, Kleban J, Gupta A. 2014. Counterfactual estimation and optimization of click metrics for search engines: a case study. In Proceedings of the 24th International Conference on the World Wide Web, pp. 929–34. New York: ACM
- S. Devlin, L. Yliniemi, D. Kudenko, and K. Tumer. Potential-based difference rewards for multiagent reinforcement learning. In Proceedings of the Thirteenth International Joint Conference on Autonomous Agents and Multiagent Systems, May 2014
- M. J. Hausknecht. Cooperation and Communication in Multiagent Deep Reinforcement Learning. PhD thesis, The University of Texas at Austin, 2016
- N. B ̈auerle and A. Mundt. Dynamic mean-risk optimization in a binomial model. Mathematical Methods of Operations Research, 70(2):219–239, 2009.
- Mnih A, Kavukcuoglu K. 2013. Learning word embeddings efficiently with noise-contrastive estimation. In Advances in Neural Information Processing Systems, Vol. 26, ed. Z Ghahramani, M Welling, C Cortes, ND Lawrence, KQ Weinberger, pp. 2265–73. San Diego, CA: Neural Inf. Process. Syst. Found.