AUC Score :
Short-term Tactic1 :
Dominant Strategy :
Time series to forecast n:
ML Model Testing : Ensemble Learning (ML)
Hypothesis Testing : Chi-Square
Surveillance : Major exchange and OTC
1Short-term revised.
2Time series is updated based on short-term trends.
Key Points
KinderCare's near-term prospects appear cautiously optimistic, driven by sustained demand for childcare services and potential for expansion. Predictions suggest modest revenue growth, supported by strategic investments in existing centers and potential acquisitions. Risk factors include challenges in staff retention and wage inflation, which could squeeze profit margins. Economic downturns and reduced government funding for childcare assistance programs pose additional threats to KinderCare's financial stability. Competitive pressures from other childcare providers and evolving parental preferences could also impact the company's performance.About KinderCare Learning
KinderCare Learning Companies, Inc. is a prominent provider of early childhood education and care services in the United States. The company operates through various brands, including KinderCare, Champions, and CCLC, offering a diverse range of programs for infants through school-age children. These programs focus on fostering children's social, emotional, physical, and cognitive development. KinderCare's curriculum is designed to be age-appropriate and engaging, aiming to prepare children for future academic success. The company emphasizes creating safe and nurturing environments staffed by qualified educators.
KinderCare focuses on providing convenient and accessible childcare solutions for working families. Its centers are strategically located throughout the country, providing parents with reliable care while they work. The company is committed to complying with all state and local regulations regarding childcare standards. KinderCare also places a significant emphasis on employee training and development, seeking to maintain a high level of quality across its network of centers. The company aims to support children, families, and its employees within its business structure.
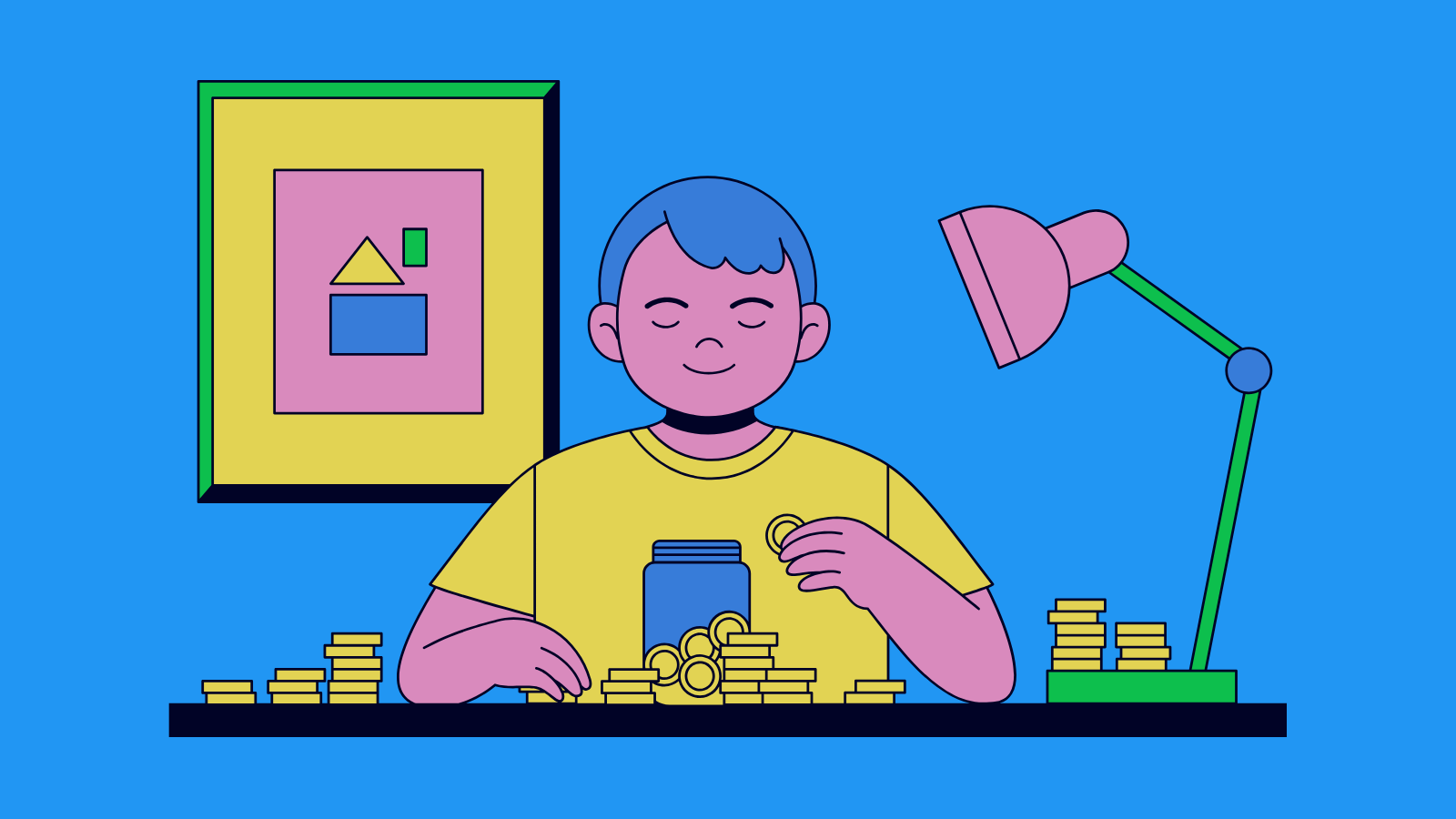
Machine Learning Model for KLC Stock Forecast
Our team of data scientists and economists proposes a comprehensive machine learning model to forecast the future performance of KinderCare Learning Companies Inc. (KLC) common stock. The core of our model will leverage a diverse set of features, categorized broadly into financial, economic, and sentiment data. Financial data will encompass KLC's historical revenue, earnings per share (EPS), debt levels, and operating margins. Economic indicators, such as GDP growth, unemployment rates, and consumer confidence, will be incorporated to reflect the broader economic environment affecting childcare demand. Crucially, sentiment analysis will be used to gauge market perception of KLC. This will be accomplished by examining news articles, social media feeds, and analyst reports to identify shifts in investor sentiment, which can significantly impact stock performance. Feature engineering is also vital; we will create new variables such as year-over-year growth rates and ratios.
The machine learning algorithm will comprise a blend of predictive modeling techniques. We will employ a combination of Recurrent Neural Networks (RNNs), specifically Long Short-Term Memory (LSTM) networks, which are well-suited for time series data to capture the temporal dependencies in KLC's financial performance and economic trends. We will also experiment with Gradient Boosting Machines (GBM), such as XGBoost, to consider relationships among the various features. The model will be trained on historical data, and its performance will be evaluated using metrics such as Mean Squared Error (MSE), and Root Mean Squared Error (RMSE), and, most importantly, Sharpe ratio to ensure it can generate profitable trading strategies. We will also consider model explainability, using techniques such as SHAP values, to understand the key drivers behind the model's predictions.
The implementation will involve a rigorous backtesting and validation process. The model's performance will be evaluated on a hold-out data set not used during training. Moreover, the model's forecasts will be assessed within a simulated trading environment to gauge its effectiveness in generating buy/sell signals, and any overestimation or underestimation of stock returns. Our team will provide periodic recalibration and retraining of the model with new data to maintain its accuracy and adapt to changing market conditions. These steps are critical for the practical applicability and reliability of our KLC stock forecast model, offering a robust framework for informed investment decision-making. Furthermore, we will monitor and integrate any emerging trends such as evolving childcare policies, technological advancements in education, and demographic shifts, as they can influence long-term business performance.
ML Model Testing
n:Time series to forecast
p:Price signals of KinderCare Learning stock
j:Nash equilibria (Neural Network)
k:Dominated move of KinderCare Learning stock holders
a:Best response for KinderCare Learning target price
For further technical information as per how our model work we invite you to visit the article below:
How do KappaSignal algorithms actually work?
KinderCare Learning Stock Forecast (Buy or Sell) Strategic Interaction Table
Strategic Interaction Table Legend:
X axis: *Likelihood% (The higher the percentage value, the more likely the event will occur.)
Y axis: *Potential Impact% (The higher the percentage value, the more likely the price will deviate.)
Z axis (Grey to Black): *Technical Analysis%
KinderCare Financial Outlook and Forecast
The financial outlook for KinderCare, a leading provider of early childhood education and care services, appears moderately positive, considering several factors. The demand for childcare remains relatively robust, driven by the continued participation of parents in the workforce. KinderCare benefits from its established brand recognition, extensive network of centers across the United States, and a focus on providing a standardized and regulated learning environment. Further, the company has demonstrated its ability to adapt to evolving market dynamics, including incorporating technology to improve the parent experience and operational efficiency. Increased government funding and subsidies for childcare could also benefit KinderCare, potentially leading to increased enrollment and revenue. KinderCare's strategic investments in technology and curriculum development further contribute to its ability to offer valuable services to families.
The company's revenue is expected to experience steady growth. This growth will be supported by factors such as increased enrollment, expansion of existing centers, and strategic acquisitions. KinderCare is also working to improve operational efficiency, including cost management and staff retention. Additionally, KinderCare continues to invest in professional development for its teachers and caregivers, contributing to higher quality service delivery. The company's focus on providing high-quality early childhood education and care, is expected to drive customer loyalty and attract new customers. KinderCare can also optimize its pricing strategy based on location and service offerings to maximize revenue.
However, there are several factors that must be considered in the financial forecast. These include labor market conditions, economic uncertainty, and competition within the childcare sector. Labor costs represent a significant expense for KinderCare and may be subject to inflationary pressures and competition for qualified staff. Economic downturns could negatively impact enrollment, as parents may reduce their childcare expenses during challenging financial times. KinderCare also faces competition from other childcare providers, including local and regional competitors. Further, changes in government regulations and funding policies could impact the company's financial performance. Additionally, any unforeseen disruptions, such as another pandemic, could have a negative impact on enrollment and revenues.
Overall, the financial outlook for KinderCare is considered positive, assuming the company can navigate the risks associated with the childcare industry. The company is expected to experience steady revenue growth and improvements in operational efficiency. However, several risks could limit this growth. The greatest risk is increased competition and any negative impact from labor costs. The company must manage expenses effectively to maintain profitability. Further, the company should continue its strategic approach to maintain its strong brand reputation and market position. KinderCare's ability to adapt to changing market conditions will also be a key factor in determining its long-term success.
Rating | Short-Term | Long-Term Senior |
---|---|---|
Outlook | B3 | B2 |
Income Statement | Ba1 | B3 |
Balance Sheet | C | C |
Leverage Ratios | C | Ba2 |
Cash Flow | Caa2 | C |
Rates of Return and Profitability | B2 | Baa2 |
*Financial analysis is the process of evaluating a company's financial performance and position by neural network. It involves reviewing the company's financial statements, including the balance sheet, income statement, and cash flow statement, as well as other financial reports and documents.
How does neural network examine financial reports and understand financial state of the company?
References
- E. Altman. Constrained Markov decision processes, volume 7. CRC Press, 1999
- Wan M, Wang D, Goldman M, Taddy M, Rao J, et al. 2017. Modeling consumer preferences and price sensitiv- ities from large-scale grocery shopping transaction logs. In Proceedings of the 26th International Conference on the World Wide Web, pp. 1103–12. New York: ACM
- Hastie T, Tibshirani R, Wainwright M. 2015. Statistical Learning with Sparsity: The Lasso and Generalizations. New York: CRC Press
- Mikolov T, Yih W, Zweig G. 2013c. Linguistic regularities in continuous space word representations. In Pro- ceedings of the 2013 Conference of the North American Chapter of the Association for Computational Linguistics: Human Language Technologies, pp. 746–51. New York: Assoc. Comput. Linguist.
- E. Altman. Constrained Markov decision processes, volume 7. CRC Press, 1999
- Blei DM, Lafferty JD. 2009. Topic models. In Text Mining: Classification, Clustering, and Applications, ed. A Srivastava, M Sahami, pp. 101–24. Boca Raton, FL: CRC Press
- V. Borkar. Q-learning for risk-sensitive control. Mathematics of Operations Research, 27:294–311, 2002.