AUC Score :
Short-term Tactic1 :
Dominant Strategy :
Time series to forecast n:
ML Model Testing : Modular Neural Network (Market News Sentiment Analysis)
Hypothesis Testing : Linear Regression
Surveillance : Major exchange and OTC
1Short-term revised.
2Time series is updated based on short-term trends.
Key Points
Indivior's shares are projected to experience moderate volatility due to their reliance on the opioid addiction treatment market. Continued market competition and potential regulatory changes pose significant risks, potentially impacting revenue from key products like Suboxone. The company is likely to explore diversification strategies; however, such initiatives carry execution risk. Successful outcomes in clinical trials for new products would be a positive catalyst, but failure could lead to further declines. Overall, Indivior stock may exhibit sideways movement with possible dips, contingent upon the evolving regulatory landscape and the successful commercialization of its pipeline.About Indivior PLC
Indivior PLC is a specialty pharmaceutical company focused on developing and marketing innovative therapies for substance use disorders and serious mental illnesses. The company's primary focus is on opioid use disorder (OUD) and alcohol use disorder (AUD) treatments. Its portfolio includes treatments like Suboxone, used to manage opioid dependence, and Sublocade, an extended-release injectable buprenorphine formulation. Indivior operates globally, with its products available in various countries and is dedicated to addressing unmet medical needs in behavioral health.
Indivior invests heavily in research and development to expand its product pipeline and explore new treatment approaches. The company faces challenges related to market competition, regulatory changes, and evolving healthcare policies. It strives to collaborate with healthcare providers, advocacy groups, and government agencies to improve patient access to life-saving treatments and promote better outcomes for individuals suffering from substance use disorders and mental illnesses.
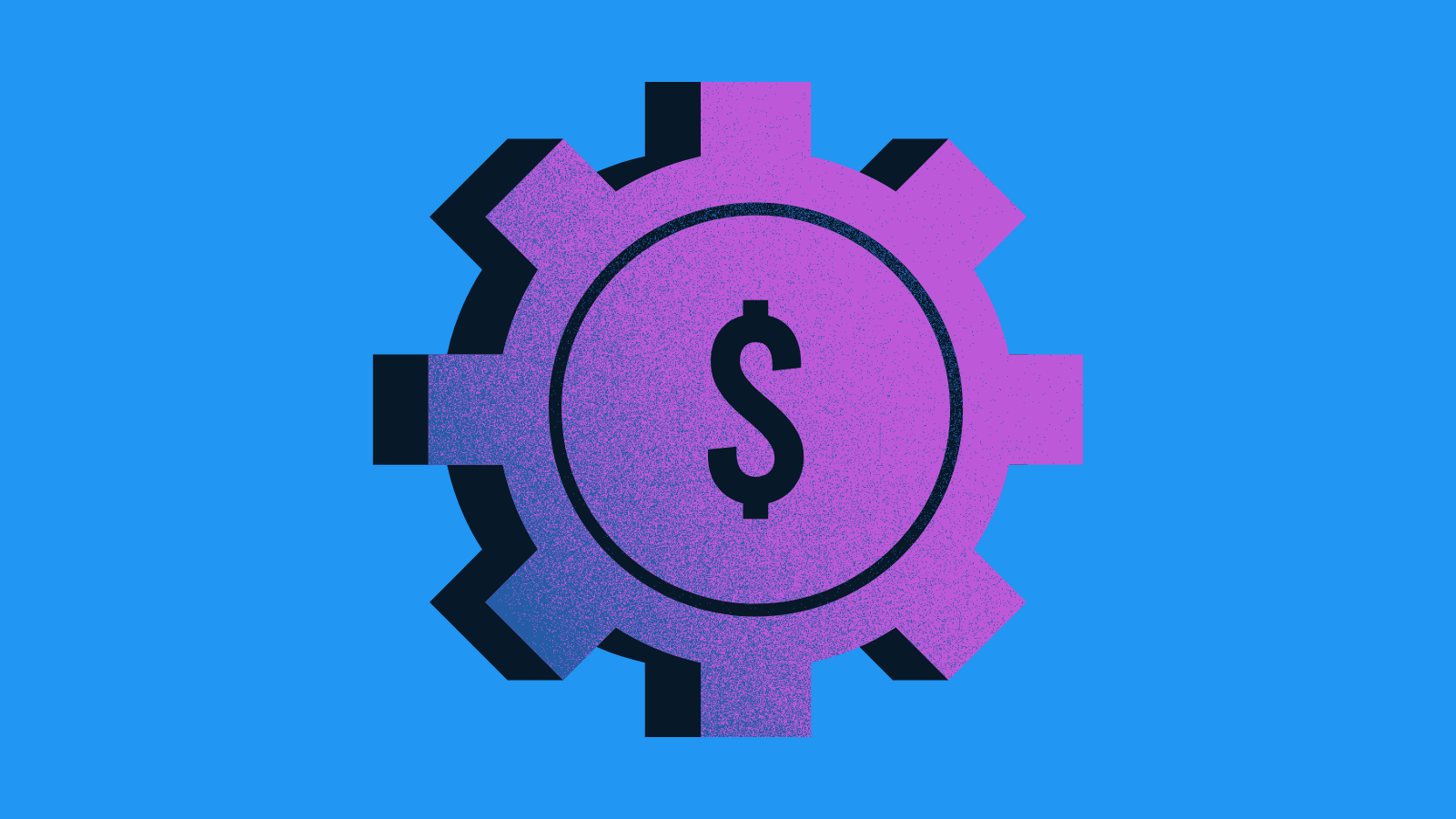
INDV Stock Prediction Machine Learning Model
Our team of data scientists and economists proposes a machine learning model for forecasting the performance of Indivior PLC Ordinary Shares (INDV). The model will be built upon a comprehensive dataset encompassing various financial and macroeconomic indicators. Key features will include historical stock data (price, volume), financial statements (revenue, earnings, debt), macroeconomic factors (GDP growth, inflation rates, interest rates, currency exchange rates), and relevant industry-specific data (market trends, competitor performance, regulatory changes). We plan to explore a range of algorithms, including Recurrent Neural Networks (RNNs), particularly Long Short-Term Memory (LSTM) networks, due to their effectiveness in capturing temporal dependencies in time series data. Other algorithms, such as Random Forests and Gradient Boosting, will also be considered for their ability to handle complex relationships and feature interactions.
The model development will involve rigorous data preprocessing, including cleaning, handling missing values, and feature engineering. Feature engineering will focus on creating informative variables from the raw data, such as technical indicators (moving averages, RSI), sentiment analysis scores (from news articles and social media), and ratios derived from financial statements. The dataset will be split into training, validation, and testing sets to ensure the model's robustness and generalizability. Model performance will be evaluated using metrics such as Mean Squared Error (MSE), Root Mean Squared Error (RMSE), and Mean Absolute Error (MAE), along with more stock-specific metrics like Sharpe Ratio. To mitigate overfitting, we will employ techniques like cross-validation, regularization, and hyperparameter tuning using methods such as grid search or Bayesian optimization.
The final model will generate forecasts for the INDV stock, providing insights into potential future performance. The forecasts will be presented with associated confidence intervals to reflect the inherent uncertainty in stock market predictions. Our team will continuously monitor the model's performance and retrain it periodically with updated data to maintain its accuracy and relevance. Furthermore, we will incorporate feedback and insights from financial analysts and industry experts to refine the model and ensure its alignment with real-world market dynamics. The resulting model will be a valuable tool for investors and financial professionals seeking to make informed decisions regarding INDV stock.
ML Model Testing
n:Time series to forecast
p:Price signals of Indivior PLC stock
j:Nash equilibria (Neural Network)
k:Dominated move of Indivior PLC stock holders
a:Best response for Indivior PLC target price
For further technical information as per how our model work we invite you to visit the article below:
How do KappaSignal algorithms actually work?
Indivior PLC Stock Forecast (Buy or Sell) Strategic Interaction Table
Strategic Interaction Table Legend:
X axis: *Likelihood% (The higher the percentage value, the more likely the event will occur.)
Y axis: *Potential Impact% (The higher the percentage value, the more likely the price will deviate.)
Z axis (Grey to Black): *Technical Analysis%
Indivior PLC: Financial Outlook and Forecast
The financial outlook for Indivior (INDV) appears promising, largely driven by its core business in addiction treatment, specifically for opioid use disorder (OUD). The company's flagship product, Suboxone Film, continues to generate substantial revenue, and the launch of newer formulations, like Sublocade, has further diversified its product portfolio and market presence. Recent clinical trial results and regulatory approvals are demonstrating ongoing success in expanding its product offerings, thus securing the company's position in the market. Furthermore, INDV's strategic focus on strengthening its commercial infrastructure and expanding its geographic reach, particularly in high-growth markets, suggests a commitment to sustainable revenue generation. Management's proactive approach to portfolio optimization, which includes exploring new therapeutic areas and pipeline expansions, is a testament to the company's forward-thinking and growth strategies.
Revenue forecasts for INDV are generally positive, with analysts anticipating continued growth over the next few years. This growth is expected to be supported by increasing demand for opioid addiction treatments and the company's effective execution of its strategic plans. Key factors driving revenue growth include Sublocade's increasing market share, favorable pricing dynamics, and successful uptake of new product launches. The company's ability to manage its cost base effectively, including research and development (R&D) expenses and selling, general, and administrative (SG&A) expenses, is also expected to contribute to improved profitability. The company's emphasis on data-driven decision-making, supported by real-world evidence and market research, is expected to enhance its ability to anticipate and adapt to market changes, thus supporting positive financial performance.
Profitability prospects for INDV are encouraging. The company is expected to leverage its established infrastructure and economies of scale to drive margin expansion. By streamlining operations, optimizing its supply chain, and implementing disciplined cost management practices, the company is likely to improve its operating margins. Furthermore, successful product launches and an effective sales force are likely to provide an improved product mix and better financial performance in upcoming years. Moreover, the ability to maintain its intellectual property rights and defend against generic competition is critical to maintaining profitability. Investors and analysts will be closely monitoring the company's R&D pipeline and its progress in developing innovative treatments. Overall, the company's focus on operational efficiency, combined with its robust product portfolio, should create favorable financial conditions.
Based on the factors above, the financial forecast for INDV is positive. INDV is expected to experience sustained revenue and profit growth in the near to mid-term. The significant risk to this forecast remains in the competition from generic drug manufacturers and future regulatory environments. Furthermore, changes in reimbursement policies, along with the potential impact of legal disputes related to opioid-related litigations, could influence financial performance. Despite these risks, the company's strong market position, its robust product pipeline, and effective management strategies, position INDV to potentially achieve significant financial success, delivering value for its shareholders.
Rating | Short-Term | Long-Term Senior |
---|---|---|
Outlook | B2 | Ba3 |
Income Statement | Baa2 | B3 |
Balance Sheet | C | Baa2 |
Leverage Ratios | Baa2 | Ba1 |
Cash Flow | Caa2 | B3 |
Rates of Return and Profitability | Caa2 | B1 |
*Financial analysis is the process of evaluating a company's financial performance and position by neural network. It involves reviewing the company's financial statements, including the balance sheet, income statement, and cash flow statement, as well as other financial reports and documents.
How does neural network examine financial reports and understand financial state of the company?
References
- Banerjee, A., J. J. Dolado, J. W. Galbraith, D. F. Hendry (1993), Co-integration, Error-correction, and the Econometric Analysis of Non-stationary Data. Oxford: Oxford University Press.
- J. Filar, L. Kallenberg, and H. Lee. Variance-penalized Markov decision processes. Mathematics of Opera- tions Research, 14(1):147–161, 1989
- Holland PW. 1986. Statistics and causal inference. J. Am. Stat. Assoc. 81:945–60
- Dietterich TG. 2000. Ensemble methods in machine learning. In Multiple Classifier Systems: First International Workshop, Cagliari, Italy, June 21–23, pp. 1–15. Berlin: Springer
- S. J. Russell and A. Zimdars. Q-decomposition for reinforcement learning agents. In Machine Learning, Proceedings of the Twentieth International Conference (ICML 2003), August 21-24, 2003, Washington, DC, USA, pages 656–663, 2003.
- V. Borkar. Stochastic approximation: a dynamical systems viewpoint. Cambridge University Press, 2008
- Swaminathan A, Joachims T. 2015. Batch learning from logged bandit feedback through counterfactual risk minimization. J. Mach. Learn. Res. 16:1731–55