AUC Score :
Short-term Tactic1 :
Dominant Strategy :
Time series to forecast n:
ML Model Testing : Statistical Inference (ML)
Hypothesis Testing : Pearson Correlation
Surveillance : Major exchange and OTC
1Short-term revised.
2Time series is updated based on short-term trends.
Key Points
IMCC's future hinges on its ability to secure further lunar missions and successfully execute them. Successful mission outcomes and sustained technological advancements in lunar infrastructure and data services would likely drive significant revenue growth, potentially leading to positive investor sentiment and a rising stock price. Conversely, the company faces considerable risks. Delays or failures in future lunar missions, increased competition from established aerospace companies and emerging space startups, and potential cost overruns on existing contracts could negatively impact financial performance, investor confidence, and subsequently, the stock's valuation. Furthermore, the company is exposed to risks associated with government contracts, technological obsolescence, and the overall volatility of the space exploration market.About Intuitive Machines Inc.
Intuitive Machines, Inc. (LUNR) is a space exploration company focused on the development and deployment of lunar infrastructure and services. Founded to provide lunar access, the company aims to offer end-to-end services, including lunar surface access, lunar payload delivery, and data services. Its core business revolves around designing, building, and operating lunar landers to transport commercial and government payloads to the Moon. The company is also involved in developing technologies relevant to space exploration, such as in-space transportation and lunar resource utilization.
The company's mission is to establish a sustained presence on the Moon to further scientific discovery and enable commercial activities in space. Intuitive Machines is publicly traded and is involved in various partnerships with governmental agencies and private companies. By combining technological innovation with a strategic business model, LUNR endeavors to be a key player in the expanding space economy and contribute to the future of lunar exploration and utilization.
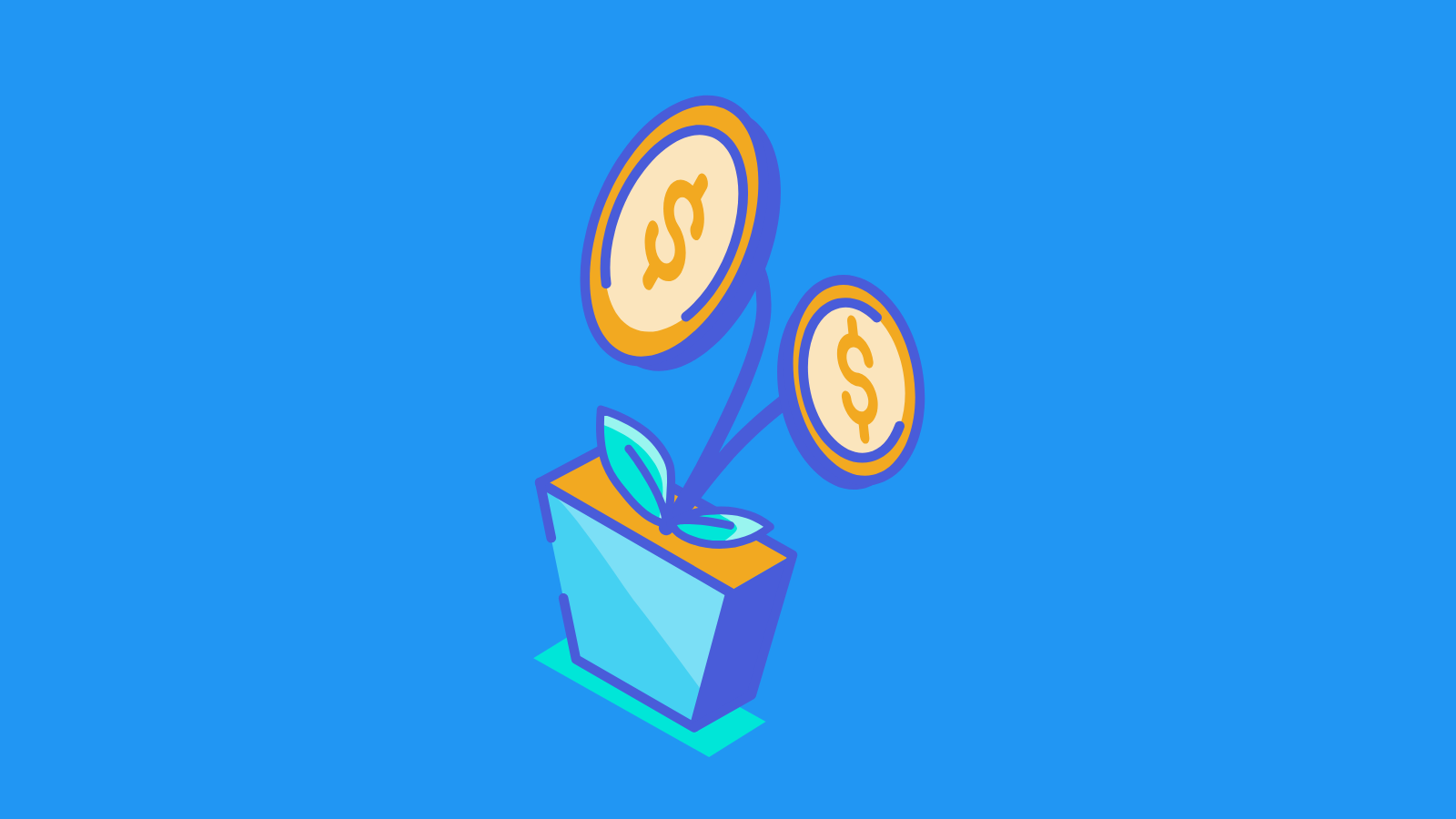
LUNR Stock Prediction Model for Intuitive Machines Inc.
Our team proposes a sophisticated machine learning model to forecast the performance of Intuitive Machines Inc. (LUNR) Class A Common Stock. This model integrates both technical and fundamental analysis techniques. We will leverage a multi-faceted approach, considering factors that influence stock price movements. The technical analysis component will incorporate historical price data, trading volume, moving averages, and various technical indicators like the Relative Strength Index (RSI), Moving Average Convergence Divergence (MACD), and Bollinger Bands. These elements provide insights into market sentiment and identify potential entry and exit points. For the fundamental analysis, we will analyze the company's financial statements, including revenue, earnings per share (EPS), debt-to-equity ratio, and cash flow. We will also incorporate macroeconomic indicators such as interest rates, inflation, and the overall performance of the space exploration industry. Furthermore, news sentiment analysis will be utilized to capture market reactions to company announcements and industry developments.
The model will employ a hybrid architecture, combining the strengths of different machine learning algorithms. We plan to use Recurrent Neural Networks (RNNs), specifically Long Short-Term Memory (LSTM) networks, to process time-series data effectively and capture complex temporal dependencies. We will also utilize Random Forest and Gradient Boosting algorithms to analyze the relationship between fundamental data and the stock price. These algorithms excel in handling non-linear relationships and high-dimensional datasets. The sentiment analysis will involve Natural Language Processing (NLP) techniques to gauge the market's perception of LUNR based on news articles, social media, and financial reports. Data pre-processing and feature engineering will be crucial steps. This includes data cleaning, handling missing values, scaling features, and creating new features from the existing data. To validate and optimize the model, we will use cross-validation and backtesting on historical data, employing metrics like mean absolute error (MAE), mean squared error (MSE), and R-squared to assess the model's accuracy.
To ensure model robustness and continuous improvement, we will implement a monitoring and retraining strategy. The model's performance will be continuously tracked and analyzed, and regular model retraining is required using the latest available data. We will monitor market conditions and company-specific developments to adjust the model's features and parameters as needed. Furthermore, we will conduct sensitivity analyses to understand the impact of different variables on the model's predictions. The model is designed to provide directional forecasts, identifying potential opportunities and risks. The output would assist in developing informed investment decisions, acknowledging that the stock market remains inherently uncertain. Regular model validation, adaptation, and a focus on risk management are essential for long-term efficacy.
```
ML Model Testing
n:Time series to forecast
p:Price signals of Intuitive Machines Inc. stock
j:Nash equilibria (Neural Network)
k:Dominated move of Intuitive Machines Inc. stock holders
a:Best response for Intuitive Machines Inc. target price
For further technical information as per how our model work we invite you to visit the article below:
How do KappaSignal algorithms actually work?
Intuitive Machines Inc. Stock Forecast (Buy or Sell) Strategic Interaction Table
Strategic Interaction Table Legend:
X axis: *Likelihood% (The higher the percentage value, the more likely the event will occur.)
Y axis: *Potential Impact% (The higher the percentage value, the more likely the price will deviate.)
Z axis (Grey to Black): *Technical Analysis%
Financial Outlook and Forecast for Intuitive Machines
Intuitive Machines (IM) is a space exploration company focused on delivering lunar surface access and data services to government and commercial customers. The company's financial outlook is heavily influenced by the success of its lunar missions, particularly the delivery of payloads to the Moon for NASA under the Commercial Lunar Payload Services (CLPS) program. Revenue generation hinges on the successful completion of these missions, along with the subsequent data collection and transmission. IM's financial trajectory is, therefore, subject to significant volatility, contingent on the success of its technological endeavors, including launch vehicle performance, lander functionality, and data transmission capabilities. The company's financial performance is also affected by its ability to secure future contracts, manage program costs effectively, and secure funding, as it is a rapidly growing firm with large capital expenditures.
The forecast for IM's financial performance over the next several years is projected to be positive, premised on continued NASA contracts and increasing commercial interest in lunar exploration. Analysts anticipate that the CLPS program will be a significant driver of revenue, with additional revenue streams arising from other commercial space ventures. The company is poised to benefit from the growing demand for lunar services, including payload delivery, resource utilization, and scientific research. However, the company's ability to convert the initial revenue from government contracts into sustainable profit is a crucial factor. Additionally, the long development cycles and substantial capital requirements associated with space exploration will affect its profitability. Any delays or failures in current or planned missions will pose an immediate risk to financial projections.
The company must also demonstrate its capacity to scale its operations effectively and manage costs efficiently as it increases its mission cadence. Profit margins will likely fluctuate significantly, dependent on the contract terms, mission complexity, and technological challenges faced. The company's financial performance is also closely correlated with the broader space industry trends, including government funding levels, commercial investment in space exploration, and technological advancements. Furthermore, IM's long-term financial health will likely depend on its capacity to expand its product and service offerings, maintain strategic partnerships, and remain competitive in a quickly growing sector of space exploration.
Given the ongoing momentum in lunar exploration, and the likelihood of successful mission deliveries, IM is likely to see positive revenue growth over the next five years. However, the nature of the industry, including mission risk and the capital intensity, indicates that the company's profitability will continue to be challenging. Risks to this positive prediction include mission failures, launch delays, increased competition, and unforeseen technical challenges. Furthermore, any alterations in government funding priorities or delays in contractual payments from NASA will influence the company's financial health. The ability of IM to successfully navigate these risks and maintain its competitive edge will determine its financial outlook.
Rating | Short-Term | Long-Term Senior |
---|---|---|
Outlook | B2 | B1 |
Income Statement | Caa2 | Baa2 |
Balance Sheet | Ba1 | Ba3 |
Leverage Ratios | B3 | B3 |
Cash Flow | Caa2 | B1 |
Rates of Return and Profitability | B3 | C |
*Financial analysis is the process of evaluating a company's financial performance and position by neural network. It involves reviewing the company's financial statements, including the balance sheet, income statement, and cash flow statement, as well as other financial reports and documents.
How does neural network examine financial reports and understand financial state of the company?
References
- Dudik M, Erhan D, Langford J, Li L. 2014. Doubly robust policy evaluation and optimization. Stat. Sci. 29:485–511
- Vilnis L, McCallum A. 2015. Word representations via Gaussian embedding. arXiv:1412.6623 [cs.CL]
- Bai J, Ng S. 2017. Principal components and regularized estimation of factor models. arXiv:1708.08137 [stat.ME]
- Abadie A, Cattaneo MD. 2018. Econometric methods for program evaluation. Annu. Rev. Econ. 10:465–503
- R. Sutton and A. Barto. Introduction to reinforcement learning. MIT Press, 1998
- F. A. Oliehoek, M. T. J. Spaan, and N. A. Vlassis. Optimal and approximate q-value functions for decentralized pomdps. J. Artif. Intell. Res. (JAIR), 32:289–353, 2008
- Bottou L. 1998. Online learning and stochastic approximations. In On-Line Learning in Neural Networks, ed. D Saad, pp. 9–42. New York: ACM