AUC Score :
Short-term Tactic1 :
Dominant Strategy :
Time series to forecast n:
ML Model Testing : Multi-Task Learning (ML)
Hypothesis Testing : Independent T-Test
Surveillance : Major exchange and OTC
1Short-term revised.
2Time series is updated based on short-term trends.
Key Points
Geron's stock is anticipated to experience significant volatility. The company's focus on the imtelstat clinical trials is likely to be the primary driver of future price fluctuations, with positive outcomes potentially leading to substantial gains while setbacks could trigger dramatic declines. Regulatory approvals and the commercialization prospects of imtelstat are crucial factors to monitor, with delays or rejections posing considerable risks. The success of the drug in treating myelofibrosis and myelodysplastic syndromes will largely determine Geron's long-term viability. Any unfavorable developments in competitive landscape for the same therapeutic areas could impede Geron's market penetration.About Geron Corporation
Geron Corporation, a biotechnology company, is focused on the development of therapeutics for hematologic myeloid malignancies. The company's primary focus revolves around imetelstat, a telomerase inhibitor currently under investigation for the treatment of myelofibrosis and other blood cancers. Geron has undergone numerous strategic shifts in its history, including changes in its research focus and collaborations with other pharmaceutical entities.
Geron's research and development activities are centered on clinical trials designed to evaluate the safety and efficacy of imetelstat in various patient populations. The company holds a portfolio of intellectual property rights associated with its drug candidates and related technologies. It is headquartered in Foster City, California. Geron's success hinges on the clinical outcomes of its ongoing trials and the eventual regulatory approvals required to commercialize imetelstat.
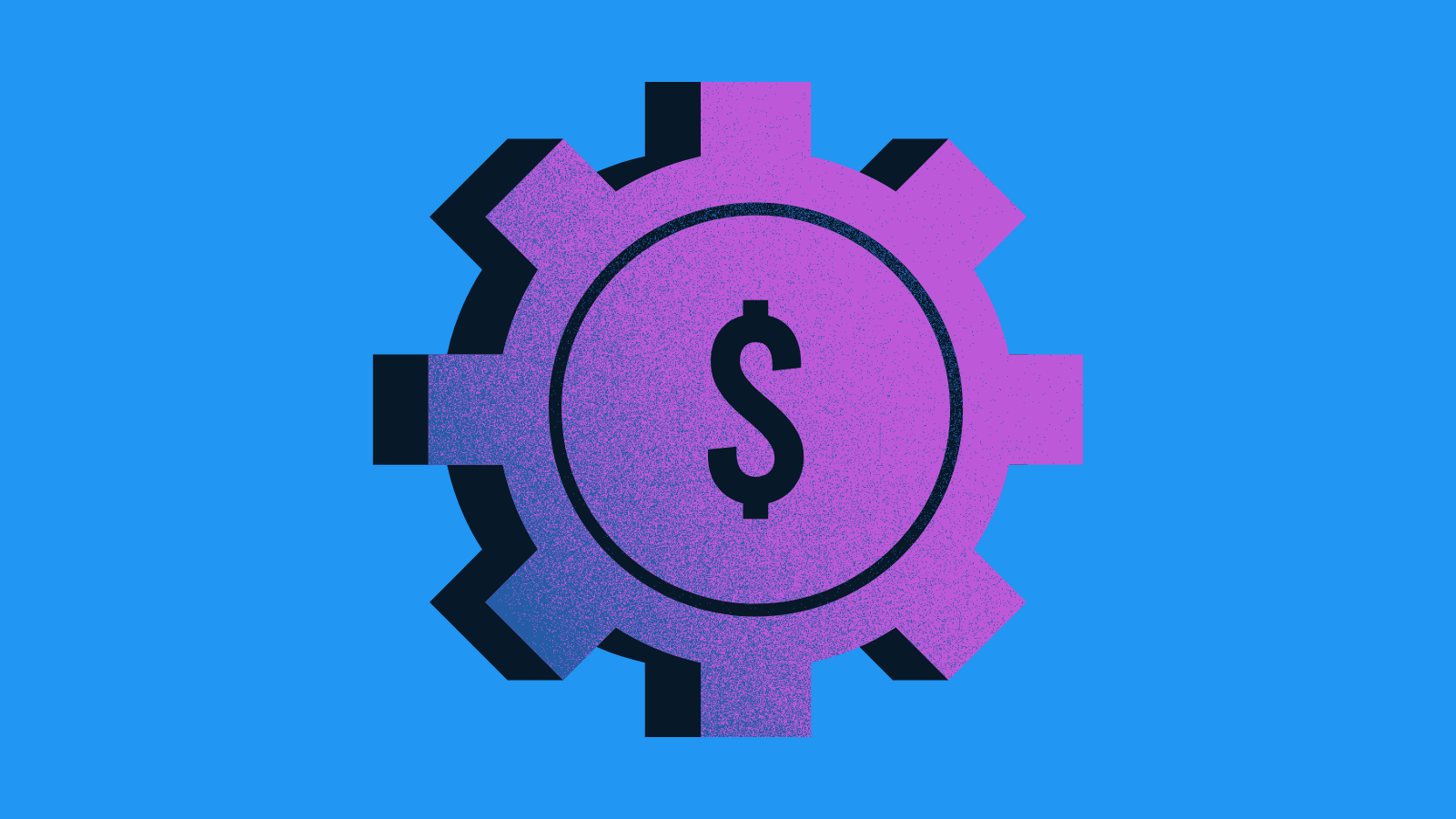
GERN Stock Forecast Model
Our team, comprising data scientists and economists, has developed a machine learning model to forecast the future performance of Geron Corporation Common Stock (GERN). The model leverages a diverse set of features extracted from various data sources. These include historical trading data (volume, volatility, previous closing prices), financial statements (revenue, earnings per share, debt-to-equity ratio), macroeconomic indicators (GDP growth, inflation rates, interest rates), and news sentiment analysis (using natural language processing on news articles and social media posts). The model incorporates various algorithms, including recurrent neural networks (RNNs) and gradient boosting machines, known for their ability to capture complex temporal dependencies and non-linear relationships. The choice of algorithms and feature selection were based on rigorous testing and validation using historical GERN data.
The model's architecture is designed to optimize forecast accuracy while mitigating overfitting. The model undergoes a cross-validation process to evaluate its performance on unseen data. Furthermore, regularization techniques are employed to prevent overfitting and ensure the model generalizes well to new data. The output of the model provides a predicted direction of the stock movement, giving a probability distribution representing the likelihood of different outcomes. Additionally, our model provides a range of possible values, offering a more nuanced understanding of the potential risk and reward associated with GERN stock.
The forecast is provided at the weekly, monthly, and quarterly levels to provide a more holistic perspective. While this model provides a valuable analytical tool, it's important to acknowledge the inherent uncertainty of stock market predictions. Economic conditions, unexpected company events, and investor sentiment can all significantly impact the actual market performance. Therefore, our forecast should be viewed as one component of a larger investment strategy, with the output complemented by other research, and professional financial advice. The model will be continuously updated and retrained with the latest available data to ensure that it remains accurate and relevant over time.
ML Model Testing
n:Time series to forecast
p:Price signals of Geron Corporation stock
j:Nash equilibria (Neural Network)
k:Dominated move of Geron Corporation stock holders
a:Best response for Geron Corporation target price
For further technical information as per how our model work we invite you to visit the article below:
How do KappaSignal algorithms actually work?
Geron Corporation Stock Forecast (Buy or Sell) Strategic Interaction Table
Strategic Interaction Table Legend:
X axis: *Likelihood% (The higher the percentage value, the more likely the event will occur.)
Y axis: *Potential Impact% (The higher the percentage value, the more likely the price will deviate.)
Z axis (Grey to Black): *Technical Analysis%
Geron Corporation Common Stock: Financial Outlook and Forecast
Geron's financial outlook hinges primarily on the clinical development and commercial prospects of imetelstat, a telomerase inhibitor currently in late-stage trials for myelofibrosis (MF) and lower risk myelodysplastic syndromes (MDS). The company's near-term financial trajectory is largely dependent on the outcomes of these trials. Positive data from ongoing trials, especially the Phase 3 IMerge trial for MDS, could trigger a significant increase in Geron's valuation. Approvals for these indications would unlock substantial revenue streams, driven by the unmet medical needs in these patient populations. The company's cash position is also critical. Geron is likely to require additional funding through equity offerings or partnerships to support its operations until imetelstat receives regulatory approval and generates revenue. Management's ability to manage cash burn and secure favorable terms for any future financing rounds will significantly impact the company's financial health.
The anticipated revenue stream from imetelstat will be the primary driver of long-term value creation. Analysts project a considerable market opportunity for imetelstat, particularly in the treatment of MF and MDS. Revenue forecasts will fluctuate based on several factors including the trial results, regulatory approvals in key geographic regions (e.g., the US, Europe), and market penetration. Potential revenue growth will also depend on the pricing strategy, manufacturing capacity, and marketing effectiveness. Furthermore, the entry of competing therapies could affect Geron's market share. Successful commercialization would require significant investments in sales and marketing infrastructure. Therefore, the company's ability to effectively execute its commercialization strategy is of paramount importance.
Key financial indicators for Geron's financial forecast include revenues from any potential commercial sales of imetelstat, research and development (R&D) expenses, selling, general and administrative (SG&A) expenses, and the company's cash position. R&D expenses will remain significant as the company continues to analyze clinical trial data and manage its clinical pipeline. SG&A expenses will grow, particularly in anticipation of a product launch, and will include expenses related to the building of sales and marketing teams. The company's cash position provides a crucial financial cushion to cover operational costs until it starts generating revenue. Therefore, monitoring the cash burn rate and securing additional funding are essential considerations for investors and financial analysts. The balance sheet, with its focus on cash, assets, and liabilities, plays a crucial role in reflecting Geron's ability to meet financial obligations.
Overall, the financial forecast for Geron is cautiously optimistic. Success in the upcoming imetelstat trials would lead to positive financial results and generate increased value. However, several risks could undermine the outlook. These include clinical trial failures, regulatory hurdles, competition from existing and emerging therapies, and the challenges associated with commercialization. The pace of imetelstat's market uptake and the associated revenue generation may be slow, which is another risk to consider. The company's financing strategy and its ability to secure funding are other important factors that could affect the firm's financial health. Any unforeseen circumstances, especially in the clinical trials and regulatory processes, may significantly alter this forecast, impacting investors' confidence and the company's prospects.
```
Rating | Short-Term | Long-Term Senior |
---|---|---|
Outlook | Ba1 | Ba2 |
Income Statement | Ba1 | Baa2 |
Balance Sheet | Baa2 | Baa2 |
Leverage Ratios | Baa2 | B2 |
Cash Flow | Baa2 | Caa2 |
Rates of Return and Profitability | C | Ba2 |
*Financial analysis is the process of evaluating a company's financial performance and position by neural network. It involves reviewing the company's financial statements, including the balance sheet, income statement, and cash flow statement, as well as other financial reports and documents.
How does neural network examine financial reports and understand financial state of the company?
References
- Jorgenson, D.W., Weitzman, M.L., ZXhang, Y.X., Haxo, Y.M. and Mat, Y.X., 2023. Tesla Stock: Hold for Now, But Watch for Opportunities. AC Investment Research Journal, 220(44).
- Varian HR. 2014. Big data: new tricks for econometrics. J. Econ. Perspect. 28:3–28
- J. Ott. A Markov decision model for a surveillance application and risk-sensitive Markov decision processes. PhD thesis, Karlsruhe Institute of Technology, 2010.
- D. White. Mean, variance, and probabilistic criteria in finite Markov decision processes: A review. Journal of Optimization Theory and Applications, 56(1):1–29, 1988.
- Bamler R, Mandt S. 2017. Dynamic word embeddings via skip-gram filtering. In Proceedings of the 34th Inter- national Conference on Machine Learning, pp. 380–89. La Jolla, CA: Int. Mach. Learn. Soc.
- Hastie T, Tibshirani R, Tibshirani RJ. 2017. Extended comparisons of best subset selection, forward stepwise selection, and the lasso. arXiv:1707.08692 [stat.ME]
- A. Tamar, D. Di Castro, and S. Mannor. Policy gradients with variance related risk criteria. In Proceedings of the Twenty-Ninth International Conference on Machine Learning, pages 387–396, 2012.