AUC Score :
Short-term Tactic1 :
Dominant Strategy :
Time series to forecast n:
ML Model Testing : Deductive Inference (ML)
Hypothesis Testing : Stepwise Regression
Surveillance : Major exchange and OTC
1Short-term revised.
2Time series is updated based on short-term trends.
Key Points
Natural Gas Futures x3 Short Leveraged Index is anticipated to experience increased volatility. A likely scenario involves a sharp downward movement reflecting anticipated decreases in natural gas prices. This is based on the leveraged nature and inverse relationship of the index. A primary risk factor is the potential for a rapid market reversal. In which a sudden increase in natural gas prices could lead to substantial losses for the index. Another concern revolves around the decay inherent in leveraged products; the daily rebalancing required to maintain the three-times short exposure can erode value over time, especially during periods of sideways price action or extended volatility. Therefore, trading this index warrants careful monitoring and strict risk management, especially due to its amplified exposure and sensitivity to even minor price swings.About Natural Gas Futures x3 Short Levera Index
The Natural Gas Futures x3 Short Leveraged Index is designed to provide a return that is approximately three times the inverse (-3x) of the daily performance of a benchmark natural gas futures contract. This index aims to profit from a decrease in the price of natural gas futures contracts. This means that if the price of the underlying natural gas futures contract falls by a certain percentage on a given day, the index is designed to increase by approximately three times that percentage, minus fees and expenses. It's important to note that the leveraged nature of the index amplifies both potential gains and losses.
Due to its leverage, this index is intended for short-term trading strategies and is not suitable for long-term investment. The daily reset mechanism is critical to understand. The index rebalances its positions daily, meaning it seeks to achieve its leveraged exposure each day. This daily compounding effect can lead to significant deviations from the -3x target return over periods longer than one day, particularly during volatile market conditions. Investors should carefully consider the associated risks, including the impact of compounding, and understand that this index is best suited for sophisticated investors with a high-risk tolerance.
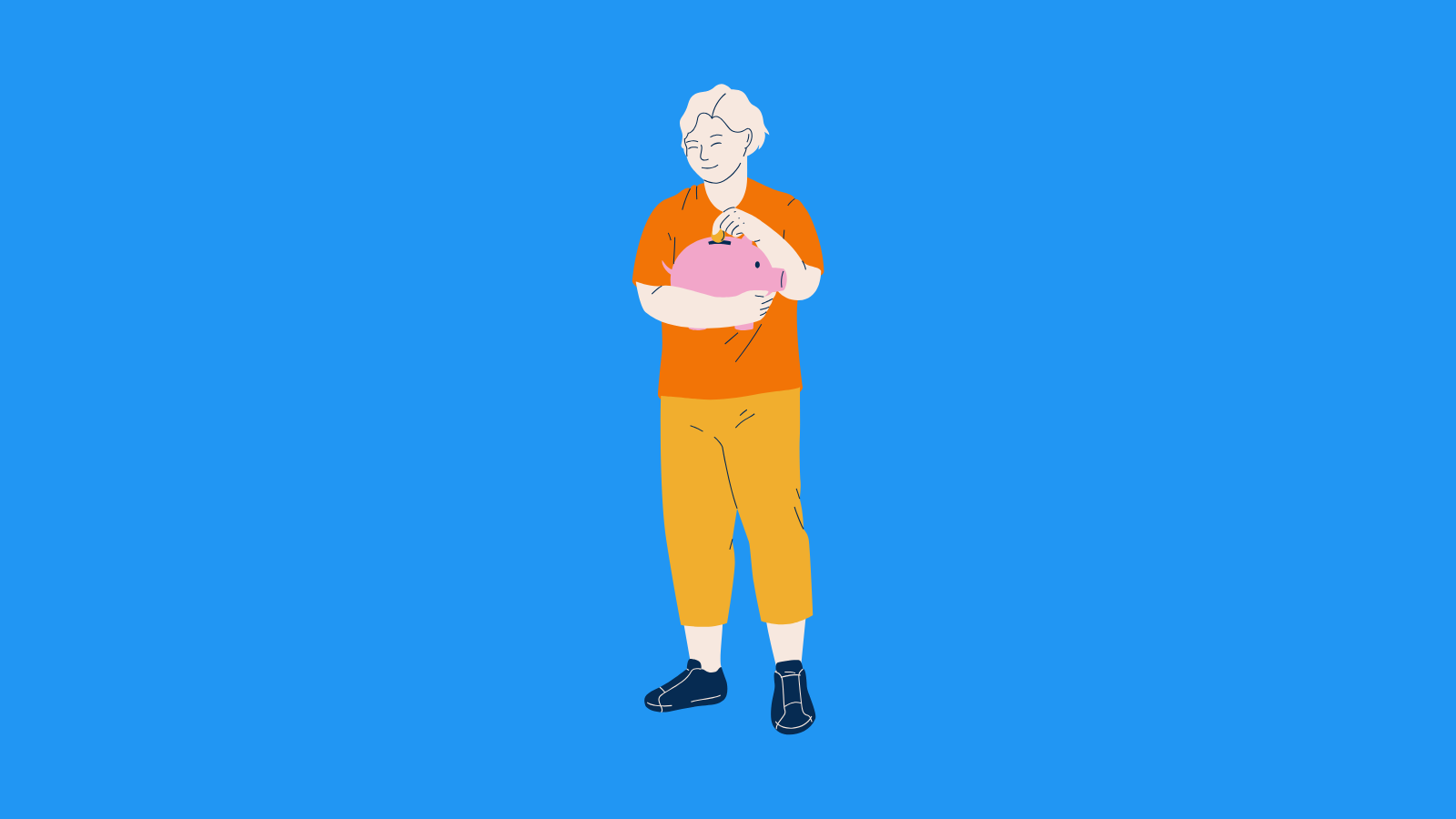
Natural Gas Futures x3 Short Leveraged Index Forecasting Model
Our team of data scientists and economists proposes a machine learning model to forecast the Natural Gas Futures x3 Short Leveraged Index. This index presents unique challenges due to its leveraged nature and inverse relationship with natural gas futures. To address these complexities, we will employ a hybrid approach combining various machine learning techniques. First, we will gather and preprocess historical data, including not only the index's historical values but also relevant market data. This comprehensive dataset will encompass daily and potentially intraday data on natural gas futures contracts (e.g., Henry Hub), volatility indices (like the VIX), economic indicators (e.g., industrial production, weather patterns, and inventory levels), and any announcements related to the natural gas market. The preprocessing stage will involve cleaning the data, handling missing values, and transforming the data using techniques like feature scaling, time series decomposition, and lagged variables to capture temporal dependencies.
The core of our model will leverage a combination of advanced machine learning algorithms. We plan to utilize a stacked ensemble approach, which blends the strengths of several models. This might involve training individual models such as recurrent neural networks (RNNs), particularly Long Short-Term Memory (LSTM) networks, known for their effectiveness in time series forecasting. We will also consider tree-based models like Gradient Boosting Machines (GBM) or XGBoost, which can capture non-linear relationships and feature interactions. Another critical element is to incorporate external economic factors. We aim to build a fundamental-based model for forecasting. The outputs from these individual models will then be combined using a meta-learner, like a linear regression or another machine learning algorithm, to produce a final forecast. The model performance will be rigorously evaluated using appropriate metrics, including Mean Absolute Error (MAE), Mean Squared Error (MSE), and the Directional Accuracy.
To ensure the robustness and reliability of our model, we will incorporate several measures. Firstly, we will conduct a thorough backtesting procedure, simulating the model's performance on historical data, to assess its ability to capture the index's dynamics and identify potential biases. Secondly, we will implement a rolling window approach in model training to ensure the model adapts to changing market conditions. Moreover, we intend to perform a sensitivity analysis to analyze the importance of different features and identify the key drivers of the index movements. The model will also be regularly retrained with the most recent data to maintain accuracy. Finally, we will construct a real-time monitoring system that alerts us if the actual index values differ significantly from the model's predictions, ensuring that we can adapt the model or adjust the trading strategies promptly.
```
ML Model Testing
n:Time series to forecast
p:Price signals of Natural Gas Futures x3 Short Levera index
j:Nash equilibria (Neural Network)
k:Dominated move of Natural Gas Futures x3 Short Levera index holders
a:Best response for Natural Gas Futures x3 Short Levera target price
For further technical information as per how our model work we invite you to visit the article below:
How do KappaSignal algorithms actually work?
Natural Gas Futures x3 Short Levera Index Forecast Strategic Interaction Table
Strategic Interaction Table Legend:
X axis: *Likelihood% (The higher the percentage value, the more likely the event will occur.)
Y axis: *Potential Impact% (The higher the percentage value, the more likely the price will deviate.)
Z axis (Grey to Black): *Technical Analysis%
Financial Outlook and Forecast for Natural Gas Futures x3 Short Leveraged Index
The Natural Gas Futures x3 Short Leveraged Index is designed to deliver triple the inverse daily performance of a benchmark natural gas futures contract. This means that when natural gas futures prices decline, the index aims to generate profits three times greater than the underlying futures' percentage decrease. Conversely, if natural gas futures prices rise, the index is designed to incur losses three times greater than the futures' percentage increase. This leveraged approach amplifies both potential gains and losses, making it a high-risk, high-reward investment instrument. Understanding the mechanics of this index requires close attention to the behavior of natural gas futures, influenced by supply and demand dynamics, seasonal trends, and geopolitical factors. The index's value resets daily, compounding returns over time. Therefore, the index's performance is significantly impacted by the volatility of the underlying natural gas futures. Investors considering this index must be prepared for significant price swings.
Analyzing the financial outlook for the Natural Gas Futures x3 Short Leveraged Index necessitates a forward-looking assessment of the natural gas market. The price of natural gas is driven by several factors, including production levels from shale plays, storage capacity, weather patterns (particularly heating and cooling demands), pipeline infrastructure constraints, and international demand, especially from Asia and Europe. In the short term, fluctuations in these factors will significantly influence the price movements of the underlying natural gas futures contracts, thereby influencing the index's performance. Furthermore, geopolitical events, such as disruptions in natural gas supply chains, can cause volatility. Seasonal demand patterns, with increased demand during winter heating seasons and summer cooling seasons, are major drivers, influencing storage levels and spot prices. Any changes to these market dynamics would immediately affect the index performance. Traders and investors are strongly advised to monitor these factors continuously.
The technical outlook involves examining historical price movements and indicators to predict future trends. Using technical analysis, one must consider the existing price channels, support and resistance levels, and key moving averages for both the natural gas futures contract and the leveraged index itself. For example, if the natural gas futures price breaks below a significant support level, this may indicate a downward trend. A short leveraged index would then be expected to perform positively. Moreover, trading volume can support trend confirmations, with higher volumes backing up the bearish move. Traders frequently utilize indicators like the Relative Strength Index (RSI) and Moving Average Convergence Divergence (MACD) to identify overbought or oversold conditions and gauge market momentum. Combining technical analysis with fundamental analysis provides a more comprehensive outlook, enhancing accuracy in decision-making. Investors must also be well-versed in the concept of decay, as leveraged products lose value over time due to compounding and volatility.
The forecast for the Natural Gas Futures x3 Short Leveraged Index is cautiously optimistic, contingent on bearish trends in natural gas prices. A mild winter or unexpected increase in natural gas production would positively impact the index. However, risks are abundant. One significant risk involves sudden reversals in natural gas prices, such as those induced by unexpected weather events or disruptions in supply. Leverage amplifies these risks, leading to potentially rapid and substantial losses. The inherent decay associated with leveraged products is another risk. The index's daily reset mechanism and compounding effects might lead to significant divergence from the inverse three times daily performance of the underlying assets over longer holding periods. Therefore, this index is best suited for sophisticated investors with a short-term investment horizon and a high tolerance for risk. Constant monitoring of market dynamics and risk management strategies are indispensable.
```Rating | Short-Term | Long-Term Senior |
---|---|---|
Outlook | Ba2 | B3 |
Income Statement | Caa2 | Caa2 |
Balance Sheet | Ba2 | Caa2 |
Leverage Ratios | Baa2 | Caa2 |
Cash Flow | Ba3 | C |
Rates of Return and Profitability | Baa2 | Baa2 |
*An aggregate rating for an index summarizes the overall sentiment towards the companies it includes. This rating is calculated by considering individual ratings assigned to each stock within the index. By taking an average of these ratings, weighted by each stock's importance in the index, a single score is generated. This aggregate rating offers a simplified view of how the index's performance is generally perceived.
How does neural network examine financial reports and understand financial state of the company?
References
- J. Peters, S. Vijayakumar, and S. Schaal. Natural actor-critic. In Proceedings of the Sixteenth European Conference on Machine Learning, pages 280–291, 2005.
- N. B ̈auerle and J. Ott. Markov decision processes with average-value-at-risk criteria. Mathematical Methods of Operations Research, 74(3):361–379, 2011
- Andrews, D. W. K. (1993), "Tests for parameter instability and structural change with unknown change point," Econometrica, 61, 821–856.
- M. Petrik and D. Subramanian. An approximate solution method for large risk-averse Markov decision processes. In Proceedings of the 28th International Conference on Uncertainty in Artificial Intelligence, 2012.
- Brailsford, T.J. R.W. Faff (1996), "An evaluation of volatility forecasting techniques," Journal of Banking Finance, 20, 419–438.
- A. Shapiro, W. Tekaya, J. da Costa, and M. Soares. Risk neutral and risk averse stochastic dual dynamic programming method. European journal of operational research, 224(2):375–391, 2013
- Jorgenson, D.W., Weitzman, M.L., ZXhang, Y.X., Haxo, Y.M. and Mat, Y.X., 2023. Can Neural Networks Predict Stock Market?. AC Investment Research Journal, 220(44).