AUC Score :
Short-term Tactic1 :
Dominant Strategy :
Time series to forecast n:
ML Model Testing : Active Learning (ML)
Hypothesis Testing : Linear Regression
Surveillance : Major exchange and OTC
1Short-term revised.
2Time series is updated based on short-term trends.
Key Points
Eaton Vance's outlook appears cautiously optimistic, anticipating moderate dividend yield stability due to the fund's focus on tax-advantaged income generation. The firm is expected to experience gradual price appreciation, contingent on broader market performance and investor appetite for dividend-focused funds. Key risks include interest rate volatility impacting the fund's fixed income holdings, potential declines in underlying equity values within its portfolio, and fluctuations in investor demand for tax-advantaged investments.About Eaton Vance
Eaton Vance Tax-Advantaged Dividend Income Fund (EVT) is a closed-end fund managed by Eaton Vance Management. Its primary investment objective is to provide a high level of after-tax total return. The fund typically invests in a diversified portfolio of dividend-paying common and preferred stocks. This approach aims to generate income while minimizing the impact of federal income taxes. EVT may also utilize options strategies, such as writing covered call options, to enhance income and potentially offset declines in the underlying portfolio's value.
The fund's portfolio allocation strategy is designed to take advantage of favorable tax treatment for qualified dividend income. The investment process involves a combination of fundamental analysis and market assessment to identify suitable securities for inclusion in the portfolio. Eaton Vance actively manages the fund with the goal of providing shareholders with a stable stream of income, while also seeking long-term capital appreciation. As a closed-end fund, EVT issues a fixed number of shares through an initial public offering, which then trade on exchanges.
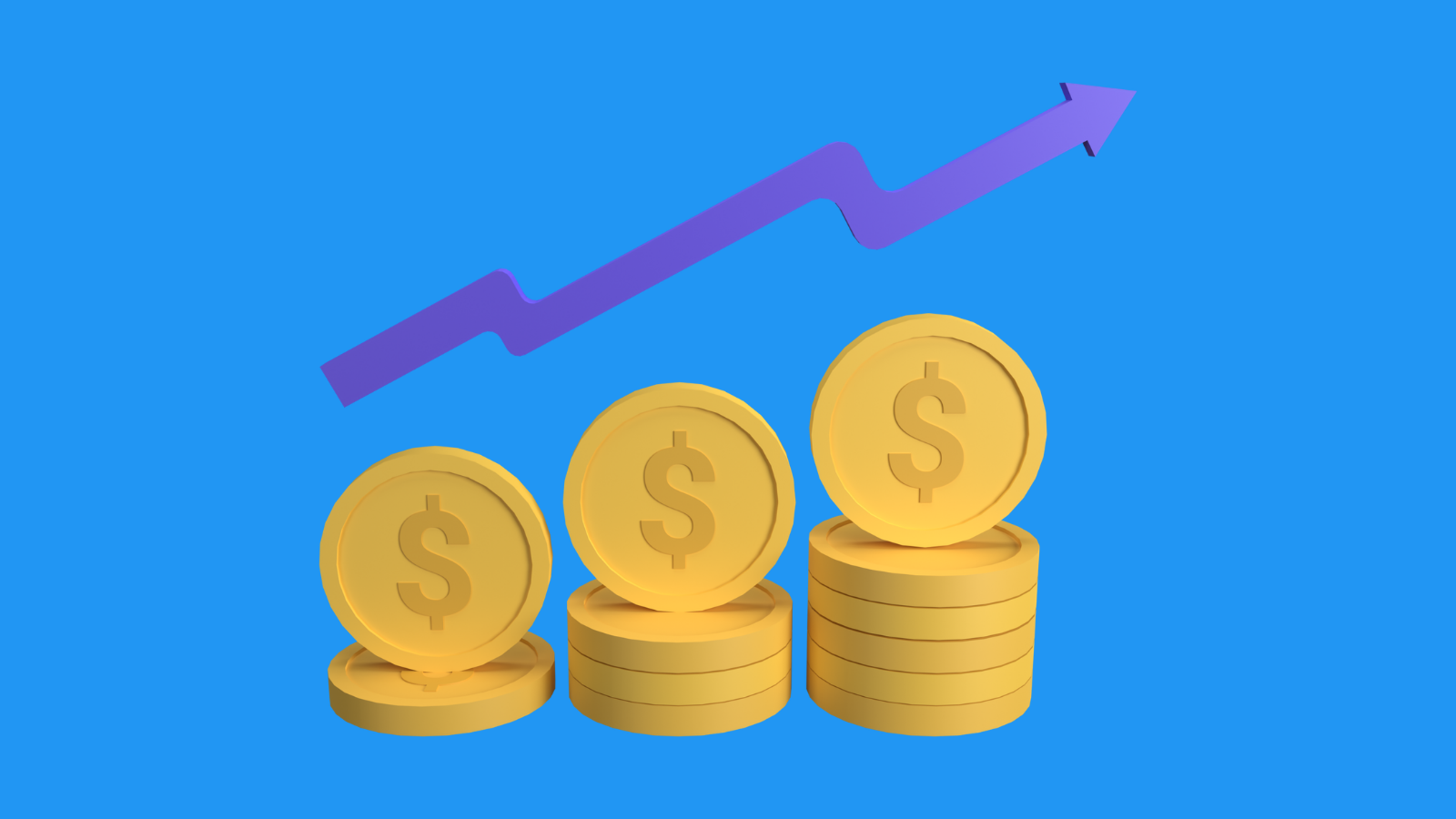
EVT Stock Forecasting Model
Our approach to forecasting the Eaton Vance Tax Advantaged Dividend Income Fund (EVT) utilizes a comprehensive machine learning model, incorporating both fundamental and technical indicators. The fundamental data will include the fund's net asset value (NAV), dividend yield, expense ratio, and portfolio composition (sector allocations and top holdings). Economic indicators such as the US Treasury yield curve, inflation rates (CPI), and changes in Federal Reserve policy are integrated as macroeconomic inputs. The technical analysis component involves processing historical trading data, specifically analyzing the fund's volume, momentum indicators like Relative Strength Index (RSI) and Moving Average Convergence Divergence (MACD), and trend-following signals based on moving averages.
The model will be built on a Random Forest algorithm due to its robustness in handling diverse data types and its ability to capture non-linear relationships between variables. The data will be preprocessed through normalization to ensure that all variables are on the same scale, and missing values will be addressed using imputation techniques appropriate for each indicator. We will construct a training dataset using historical data, then split the dataset into training, validation, and testing sets. Hyperparameter tuning, such as the number of trees in the forest and the maximum depth of the trees, will be optimized using the validation set to minimize prediction error. The model will be evaluated using appropriate metrics such as Mean Absolute Error (MAE) and Root Mean Squared Error (RMSE) to provide the prediction accuracy. The model output would focus on percentage change forecast over short-term time horizons.
After model training and validation, the final evaluation will be conducted on the independent testing set to assess the model's generalization capability. The final forecasts will be accompanied by confidence intervals to provide a measure of uncertainty. Moreover, we plan to implement a backtesting strategy to simulate the model's performance over historical data with the aim of evaluating its performance during different market phases. Furthermore, we intend to integrate the model into a real-time environment by feeding it updated market data, and continually monitor the model's performance. Regular model retraining and feature engineering will be critical to maintain its forecasting accuracy in the volatile market environment.
ML Model Testing
n:Time series to forecast
p:Price signals of Eaton Vance stock
j:Nash equilibria (Neural Network)
k:Dominated move of Eaton Vance stock holders
a:Best response for Eaton Vance target price
For further technical information as per how our model work we invite you to visit the article below:
How do KappaSignal algorithms actually work?
Eaton Vance Stock Forecast (Buy or Sell) Strategic Interaction Table
Strategic Interaction Table Legend:
X axis: *Likelihood% (The higher the percentage value, the more likely the event will occur.)
Y axis: *Potential Impact% (The higher the percentage value, the more likely the price will deviate.)
Z axis (Grey to Black): *Technical Analysis%
Eaton Vance Tax-Advantaged Dividend Income Fund Financial Outlook and Forecast
The financial outlook for EVT is largely dependent on its ability to generate and distribute a steady stream of income from its investment portfolio, which primarily consists of dividend-paying stocks and municipal bonds. The fund's structure, as a closed-end fund, allows it to employ leverage, potentially amplifying both gains and losses. This leverage can contribute to higher yields but also increases the fund's sensitivity to market fluctuations and interest rate changes. Furthermore, the fund's performance is intricately linked to the overall health of the equity and fixed-income markets. During periods of economic expansion and rising asset prices, EVT may experience positive returns, but during economic downturns or market corrections, the fund could face declines in its net asset value (NAV) and potentially experience reduced dividend payouts. The fund's tax-advantaged status, which aims to provide attractive after-tax returns, is a key selling point and relies on its ability to efficiently manage its portfolio to minimize taxable income.
The forecast for EVT's future performance hinges on several factors. Firstly, the trajectory of interest rates will significantly influence the value of its fixed-income holdings. Rising interest rates could lead to a decline in the value of the fund's bond portfolio, negatively affecting its NAV. Secondly, the performance of the equity markets will impact the fund's dividend income and capital appreciation. A sustained bull market is likely to benefit EVT, whereas a bear market could lead to reduced returns. Thirdly, the fund's management team's ability to actively manage the portfolio, selecting attractive dividend-paying stocks and municipal bonds while strategically utilizing leverage, will be crucial. Finally, the fund's ability to maintain its tax-advantaged status is critical to attracting and retaining investors. Any changes to tax laws could significantly impact the fund's attractiveness.
Looking ahead, EVT's outlook appears moderately positive, assuming a stable to slightly rising interest rate environment and continued, albeit slower, economic growth. The fund's focus on dividend-paying securities and municipal bonds provides a degree of income stability, particularly in a lower-yield environment. The fund's ability to utilize leverage could offer the potential for enhanced returns if the market continues to perform favorably. However, the fund's performance will likely be sensitive to changes in interest rates and equity market volatility. A stable interest rate environment could provide support for the fund's bond holdings, while modest equity market gains could boost dividend income. The fund's ability to attract and retain investors will depend on its ability to deliver attractive yields and maintain its tax-advantaged status.
The primary risk to this forecast is a rapid increase in interest rates, which could lead to a decline in the value of the fund's bond portfolio and a decrease in its NAV. Another risk is a significant downturn in the equity markets, which would reduce dividend income and negatively impact the fund's performance. Additional risks include changes to tax laws that could diminish the fund's tax-advantaged appeal and management's potential inability to effectively manage the portfolio. In conclusion, while the current environment offers some potential for positive returns, investors should be prepared for potential volatility and remain vigilant regarding changes in economic conditions, interest rates, and tax regulations.
Rating | Short-Term | Long-Term Senior |
---|---|---|
Outlook | Ba3 | B2 |
Income Statement | Ba3 | Caa2 |
Balance Sheet | Baa2 | Ba3 |
Leverage Ratios | Baa2 | C |
Cash Flow | B3 | Ba1 |
Rates of Return and Profitability | C | B3 |
*Financial analysis is the process of evaluating a company's financial performance and position by neural network. It involves reviewing the company's financial statements, including the balance sheet, income statement, and cash flow statement, as well as other financial reports and documents.
How does neural network examine financial reports and understand financial state of the company?
References
- T. Shardlow and A. Stuart. A perturbation theory for ergodic Markov chains and application to numerical approximations. SIAM journal on numerical analysis, 37(4):1120–1137, 2000
- Mikolov T, Yih W, Zweig G. 2013c. Linguistic regularities in continuous space word representations. In Pro- ceedings of the 2013 Conference of the North American Chapter of the Association for Computational Linguistics: Human Language Technologies, pp. 746–51. New York: Assoc. Comput. Linguist.
- Chernozhukov V, Demirer M, Duflo E, Fernandez-Val I. 2018b. Generic machine learning inference on heteroge- nous treatment effects in randomized experiments. NBER Work. Pap. 24678
- Tibshirani R, Hastie T. 1987. Local likelihood estimation. J. Am. Stat. Assoc. 82:559–67
- R. Howard and J. Matheson. Risk sensitive Markov decision processes. Management Science, 18(7):356– 369, 1972
- E. Collins. Using Markov decision processes to optimize a nonlinear functional of the final distribution, with manufacturing applications. In Stochastic Modelling in Innovative Manufacturing, pages 30–45. Springer, 1997
- Wan M, Wang D, Goldman M, Taddy M, Rao J, et al. 2017. Modeling consumer preferences and price sensitiv- ities from large-scale grocery shopping transaction logs. In Proceedings of the 26th International Conference on the World Wide Web, pp. 1103–12. New York: ACM