AUC Score :
Short-term Tactic1 :
Dominant Strategy :
Time series to forecast n:
ML Model Testing : Modular Neural Network (Emotional Trigger/Responses Analysis)
Hypothesis Testing : Wilcoxon Sign-Rank Test
Surveillance : Major exchange and OTC
1Short-term revised.
2Time series is updated based on short-term trends.
Key Points
The DJ Commodity Heating Oil is anticipated to experience a period of heightened volatility, potentially driven by fluctuations in global demand and supply dynamics. We foresee a possible upward trend due to anticipated increases in seasonal demand and supply constraints influenced by geopolitical factors. However, the price is vulnerable to corrections, particularly should there be a significant easing of supply-side pressures or weaker than expected economic indicators. Risk factors to consider include shifts in crude oil prices, disruptions in refining capacity, and unexpected weather events that could drastically alter demand patterns.About DJ Commodity Heating Oil Index
The Dow Jones Commodity Heating Oil Index serves as a benchmark reflecting the performance of the heating oil market. It is a component of the broader Dow Jones Commodity Indexes, providing a specific measure of price fluctuations within the heating oil sector. The index is calculated based on futures contracts, primarily those traded on the New York Mercantile Exchange (NYMEX). Its construction methodology ensures that the index accurately represents the real-time market dynamics of heating oil prices, offering a transparent and reliable tool for market participants.
This index is crucial for investors, traders, and analysts who need to understand and track trends in the heating oil market. It is frequently used for investment strategies, including the creation of index-linked financial products. The index's sensitivity to factors influencing the demand and supply of heating oil, such as seasonal changes, geopolitical events, and economic activity, makes it a valuable indicator for assessing market volatility and informing strategic decisions within the energy sector.
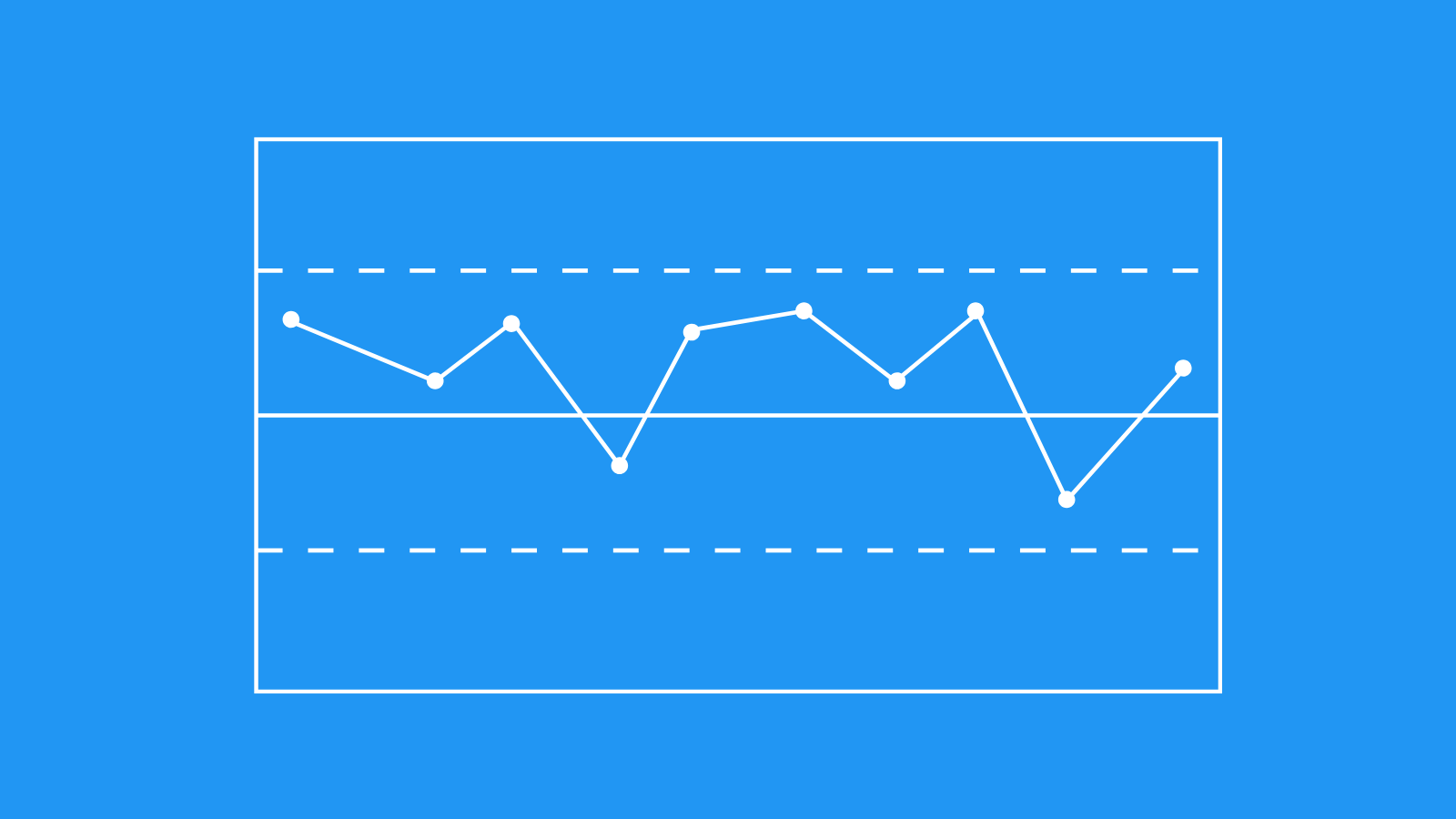
DJ Commodity Heating Oil Index Forecasting Machine Learning Model
Our team of data scientists and economists proposes a robust machine learning model for forecasting the DJ Commodity Heating Oil index. The model will employ a time-series approach, leveraging historical data on heating oil prices, alongside pertinent economic indicators. Key features we intend to incorporate include crude oil prices, natural gas prices (as a substitute fuel), inventory levels (both domestic and global), seasonal demand patterns, and macroeconomic variables like inflation rates and GDP growth. The choice of variables is informed by economic theory and prior empirical research, ensuring the model captures the complex interplay of factors influencing heating oil prices. Data preprocessing will involve cleaning, handling missing values, and feature scaling to optimize model performance. This approach will involve data transformation and feature engineering.
For model selection, we will evaluate several machine learning algorithms. Recurrent Neural Networks (RNNs), particularly LSTMs (Long Short-Term Memory), are ideal for time-series data. ARIMA models, with or without exogenous variables (ARIMAX), will provide a strong statistical baseline. Furthermore, we will also test gradient boosting algorithms, such as XGBoost or LightGBM, to explore the predictive power of non-linear relationships within the data. The model's performance will be assessed using appropriate evaluation metrics like Mean Absolute Error (MAE), Root Mean Squared Error (RMSE), and R-squared. Cross-validation techniques will be employed to ensure the model's generalizability and mitigate the risk of overfitting.
The model will be deployed to generate forecasts for a specified time horizon, such as daily or weekly projections. The system will be designed to ingest new data automatically, enabling continuous model retraining and improvement. We will regularly monitor the model's performance and conduct retrospective analysis to identify areas of improvement and potential shifts in market dynamics. Furthermore, we will integrate the model into a comprehensive analytical dashboard for easy visualization of forecasts. This dashboard will include confidence intervals and scenario analysis, to help end-users understand the uncertainty associated with the projections. Our combined expertise will ensure the model is both accurate and useful for strategic decision-making related to the DJ Commodity Heating Oil index.
ML Model Testing
n:Time series to forecast
p:Price signals of DJ Commodity Heating Oil index
j:Nash equilibria (Neural Network)
k:Dominated move of DJ Commodity Heating Oil index holders
a:Best response for DJ Commodity Heating Oil target price
For further technical information as per how our model work we invite you to visit the article below:
How do KappaSignal algorithms actually work?
DJ Commodity Heating Oil Index Forecast Strategic Interaction Table
Strategic Interaction Table Legend:
X axis: *Likelihood% (The higher the percentage value, the more likely the event will occur.)
Y axis: *Potential Impact% (The higher the percentage value, the more likely the price will deviate.)
Z axis (Grey to Black): *Technical Analysis%
DJ Commodity Heating Oil Index: Financial Outlook and Forecast
The financial outlook for the DJ Commodity Heating Oil Index is intricately tied to a confluence of global economic factors, geopolitical events, and supply-demand dynamics within the energy sector. Increased industrial activity, particularly in regions with harsh winters, directly fuels demand for heating oil. Economic expansion in developed nations and emerging markets often translates to higher energy consumption, positively influencing the index. Conversely, economic slowdowns or recessions can depress demand, leading to price corrections. Furthermore, the index is highly sensitive to geopolitical instability, as supply disruptions stemming from conflicts, sanctions, or political unrest in major oil-producing regions can significantly impact prices. These events often trigger volatility and introduce considerable uncertainty into the market, making forecasting a complex undertaking. Factors such as inventory levels, the availability of alternative fuels, and government policies related to energy consumption also play critical roles.
Forecasting the DJ Commodity Heating Oil Index necessitates careful consideration of both short-term and long-term perspectives. In the short term, weather patterns, particularly during peak heating seasons, exert significant influence. Unusually cold winters typically drive up demand, leading to price increases, while mild winters tend to have the opposite effect. Supply-side dynamics, including production levels from key oil-producing countries and the operational status of refineries, are crucial. Moreover, unexpected events, such as pipeline disruptions or refinery outages, can cause rapid price fluctuations. In the long term, the transition towards renewable energy sources and evolving environmental regulations pose considerable challenges to the heating oil market. The growing adoption of alternative heating technologies and stringent emissions standards could gradually reduce demand for traditional heating oil, potentially leading to a decline in prices over time. Technological advancements in oil extraction and refining could also have a significant impact on the future supply, which can be influenced by the decisions of OPEC+ countries.
Several external factors exert significant influence on the heating oil market. The value of the US dollar plays a crucial role, as heating oil is typically priced in US dollars. A stronger dollar can make heating oil more expensive for buyers using other currencies, potentially dampening demand. Conversely, a weaker dollar can make heating oil more affordable, thus stimulating consumption. Furthermore, the overall price of crude oil serves as a fundamental driver for the index, as heating oil is a derivative product. Any changes in the crude oil market, such as production cuts, geopolitical tensions, or shifts in global demand, can have an immediate impact on the heating oil index. The decisions of OPEC+ countries, which control a significant portion of the world's oil supply, are especially important. Their production quotas and pricing strategies can exert considerable influence on both crude oil and heating oil markets. Regulatory changes, especially regarding emissions and environmental protection, also have an indirect effect on the price.
Based on the current outlook, a moderately positive forecast for the DJ Commodity Heating Oil Index is anticipated over the next 12-18 months. A moderate economic growth is expected in many regions, which can drive the demand for heating oil. The increasing demand could contribute to price increases. However, this forecast is subject to several risks. Significant risks include the possibility of an economic slowdown in key industrial regions, unexpected geopolitical events that could disrupt oil supplies, and rapid shifts in weather patterns. A severe or extended recession could lead to a significant decrease in demand. Geopolitical instability, particularly in key oil-producing regions, could trigger supply disruptions and cause sudden price spikes. Furthermore, the rapid adoption of alternative heating technologies and the enforcement of stricter environmental regulations could put downward pressure on demand. Therefore, while the base case points toward a moderate increase, the inherent volatility of the heating oil market necessitates careful monitoring of these key risks to manage any investment.
Rating | Short-Term | Long-Term Senior |
---|---|---|
Outlook | B2 | B1 |
Income Statement | C | B2 |
Balance Sheet | B2 | Caa2 |
Leverage Ratios | Ba3 | Baa2 |
Cash Flow | B2 | C |
Rates of Return and Profitability | Ba3 | Caa2 |
*An aggregate rating for an index summarizes the overall sentiment towards the companies it includes. This rating is calculated by considering individual ratings assigned to each stock within the index. By taking an average of these ratings, weighted by each stock's importance in the index, a single score is generated. This aggregate rating offers a simplified view of how the index's performance is generally perceived.
How does neural network examine financial reports and understand financial state of the company?
References
- Thompson WR. 1933. On the likelihood that one unknown probability exceeds another in view of the evidence of two samples. Biometrika 25:285–94
- S. Bhatnagar, R. Sutton, M. Ghavamzadeh, and M. Lee. Natural actor-critic algorithms. Automatica, 45(11): 2471–2482, 2009
- J. G. Schneider, W. Wong, A. W. Moore, and M. A. Riedmiller. Distributed value functions. In Proceedings of the Sixteenth International Conference on Machine Learning (ICML 1999), Bled, Slovenia, June 27 - 30, 1999, pages 371–378, 1999.
- S. J. Russell and P. Norvig. Artificial Intelligence: A Modern Approach. Prentice Hall, Englewood Cliffs, NJ, 3nd edition, 2010
- Ashley, R. (1988), "On the relative worth of recent macroeconomic forecasts," International Journal of Forecasting, 4, 363–376.
- M. L. Littman. Friend-or-foe q-learning in general-sum games. In Proceedings of the Eighteenth International Conference on Machine Learning (ICML 2001), Williams College, Williamstown, MA, USA, June 28 - July 1, 2001, pages 322–328, 2001
- J. Filar, D. Krass, and K. Ross. Percentile performance criteria for limiting average Markov decision pro- cesses. IEEE Transaction of Automatic Control, 40(1):2–10, 1995.