AUC Score :
Short-term Tactic1 :
Dominant Strategy :
Time series to forecast n:
ML Model Testing : Ensemble Learning (ML)
Hypothesis Testing : Linear Regression
Surveillance : Major exchange and OTC
1Short-term revised.
2Time series is updated based on short-term trends.
Key Points
The DJ Commodity Gold index is anticipated to exhibit a cautiously optimistic trend, with potential for moderate gains driven by persistent global economic uncertainties, including inflation concerns and geopolitical tensions. The index could be susceptible to volatility based on fluctuations in the US dollar and shifts in interest rate policies. Risks include significant corrections should the dollar experience robust strengthening or if there's a surprising easing of geopolitical pressures. Furthermore, investor sentiment and demand, particularly from major markets, can significantly impact the index's direction.About DJ Commodity Gold Index
The Dow Jones Commodity Index (DJCI) Gold is a specialized index that tracks the performance of gold futures contracts. It is designed to offer investors a benchmark for the gold market, providing a way to assess the movement of gold prices over time. The index's composition is solely focused on gold, making it a straightforward and easily understandable tool for monitoring gold's price fluctuations and trends. This narrow focus allows investors to concentrate on the specific dynamics of the gold market without the complexities introduced by broader commodity indices.
The DJCI Gold is a crucial tool for various investment strategies, including tracking market trends, comparing investment returns, and developing financial products. Financial professionals and investors commonly use the index to understand gold's role in portfolio diversification and inflation hedging. Its transparency and straightforward methodology make it a valuable resource for those interested in the gold market. Furthermore, changes to the index's underlying methodologies are infrequent to maintain consistency and allow investors to analyze market trends through the lens of the DJCI Gold.
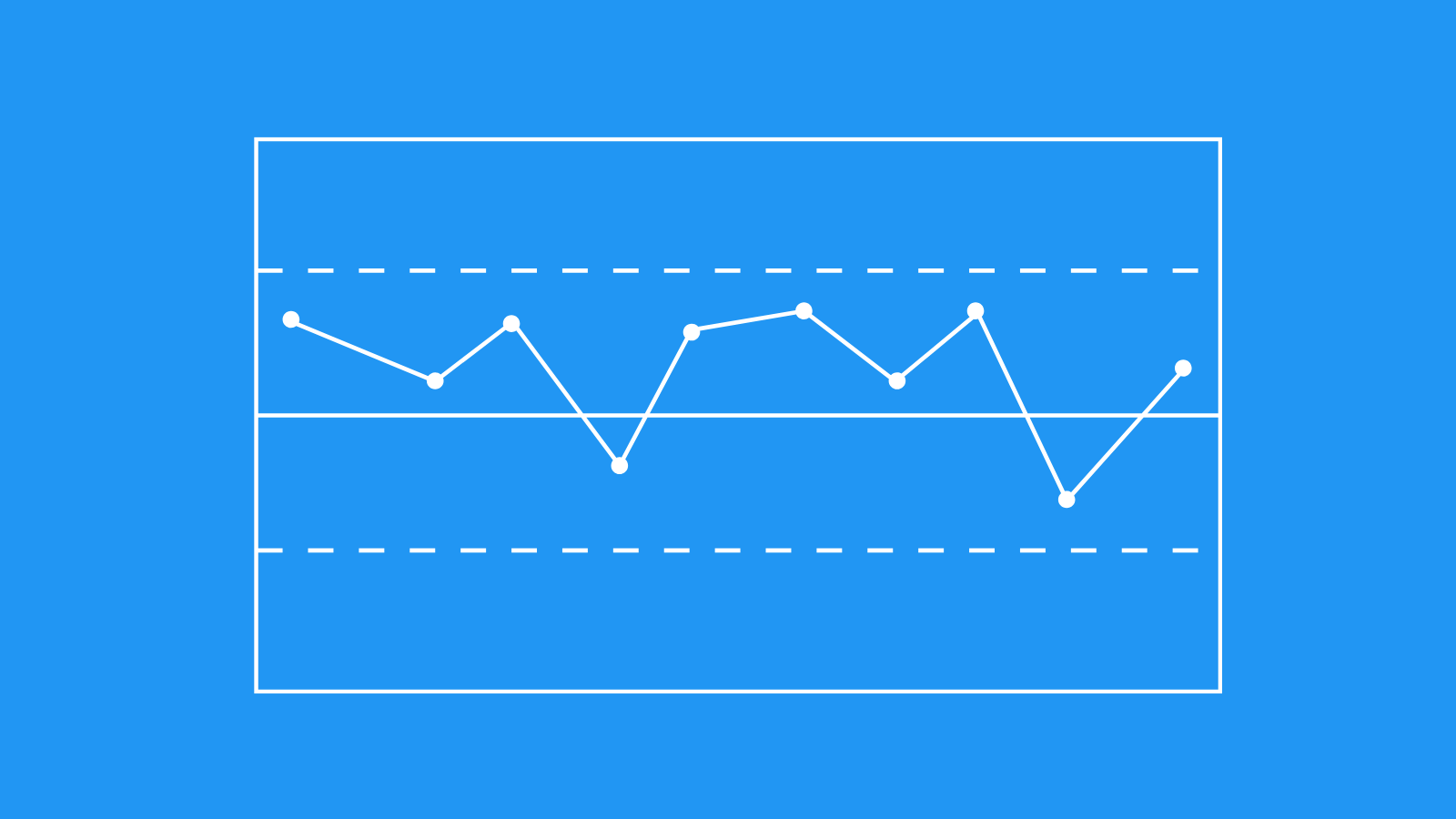
DJ Commodity Gold Index Forecast Model
Our team of data scientists and economists has developed a machine learning model to forecast the DJ Commodity Gold Index. The model leverages a comprehensive dataset encompassing a multitude of economic and market indicators. These include historical gold prices, interest rates from key central banks (like the Federal Reserve and the European Central Bank), inflation data (CPI and PPI), currency exchange rates (particularly the US Dollar), geopolitical risk indices, global economic growth indicators (such as GDP growth rates), and supply-demand dynamics for gold. We employ a combination of feature engineering techniques to extract meaningful insights from these raw data points. This involves creating lagged variables to capture time-series dependencies and generating interaction terms to model complex relationships between different variables. The model aims to predict the DJ Commodity Gold Index's movement over a specified forecasting horizon, potentially ranging from short-term (daily or weekly) to medium-term (monthly or quarterly).
The core of our model employs a Gradient Boosting Machine (GBM) algorithm. GBMs are well-suited for handling complex relationships and non-linear patterns commonly found in financial markets. The GBM is trained on historical data, with a portion of the data reserved for validation and testing to ensure the model's generalizability. We use a range of evaluation metrics, including Mean Absolute Error (MAE), Root Mean Squared Error (RMSE), and R-squared, to assess the model's performance. Furthermore, the model incorporates robust techniques to prevent overfitting, such as cross-validation and regularization. Parameter tuning is a crucial aspect, where we optimize hyperparameters (e.g., learning rate, number of trees, and tree depth) using grid search or random search to enhance the model's predictive accuracy.
The model's output will be presented in a clear and interpretable format, including point forecasts, prediction intervals, and key drivers of the gold price movements. The primary application of this model is to provide actionable insights for investment strategies, risk management, and economic analysis related to the gold market. We plan to continuously monitor and update the model with fresh data and incorporate new features as market conditions evolve. We will perform regular backtesting to assess the model's performance, identify its limitations, and refine it. We also plan to provide regular reports. The team will also investigate alternative models, such as Recurrent Neural Networks (RNNs), specifically Long Short-Term Memory (LSTM) networks, which are designed to capture sequential data, to further improve the prediction accuracy of the DJ Commodity Gold index.
ML Model Testing
n:Time series to forecast
p:Price signals of DJ Commodity Gold index
j:Nash equilibria (Neural Network)
k:Dominated move of DJ Commodity Gold index holders
a:Best response for DJ Commodity Gold target price
For further technical information as per how our model work we invite you to visit the article below:
How do KappaSignal algorithms actually work?
DJ Commodity Gold Index Forecast Strategic Interaction Table
Strategic Interaction Table Legend:
X axis: *Likelihood% (The higher the percentage value, the more likely the event will occur.)
Y axis: *Potential Impact% (The higher the percentage value, the more likely the price will deviate.)
Z axis (Grey to Black): *Technical Analysis%
DJ Commodity Gold Index: Outlook and Forecast
The Dow Jones Commodity Index (DJCI) Gold sub-index reflects the performance of gold futures contracts. Understanding its financial outlook necessitates a comprehensive analysis of the fundamental drivers impacting gold prices. Key elements influencing the index's trajectory include global economic conditions, specifically inflation rates and interest rate policies implemented by major central banks such as the Federal Reserve. Periods of heightened inflation often boost gold's appeal as a hedge against declining purchasing power. Conversely, aggressive interest rate hikes, designed to curb inflation, can strengthen the U.S. dollar, making gold more expensive for international buyers and potentially dampening demand. Furthermore, geopolitical instability, such as wars or heightened political tensions, tends to drive investors towards safe-haven assets like gold, resulting in price increases. Shifts in demand from major consumers like India and China, and fluctuations in supply from mining activities also contribute to the index's performance. Analyzing these interconnected factors provides insights into potential future movements.
Current market dynamics paint a mixed picture for the DJCI Gold sub-index. Inflation, while showing signs of moderation in some regions, remains a persistent concern globally. The ongoing war in Ukraine and other geopolitical risks contribute to elevated uncertainty, potentially supporting gold prices due to its safe-haven status. However, the strength of the U.S. dollar, influenced by the Federal Reserve's monetary policy, presents a headwind. The Fed's commitment to tackling inflation could result in further interest rate increases, strengthening the dollar and making gold less attractive. Additionally, increased risk appetite among investors, as evidenced by rallies in equity markets, might diminish gold's allure as a safe haven. Therefore, the index's performance will likely be determined by a tug-of-war between inflationary pressures and the strength of the dollar, along with the evolving geopolitical landscape and any unexpected shifts in supply or demand.
Analyzing the technical indicators and historical trends offers further context. Examining moving averages, relative strength indexes (RSIs), and other technical tools can help identify potential support and resistance levels. The performance of gold in the past, especially during similar economic climates and geopolitical events, offers a basis for understanding potential future patterns. Examining the correlation between gold prices and other asset classes, like government bonds and equities, aids in assessing risk. The current trading volume and open interest in gold futures contracts provide clues about the prevailing market sentiment and the commitment of traders. Examining the trend of these metrics helps confirm and refine the overall outlook. Observing these technical details along with the fundamental analysis provides a well-rounded picture for investors.
Given the multifaceted factors at play, the outlook for the DJCI Gold index is cautiously optimistic. The continued risk of inflation and the persistent presence of geopolitical tensions will probably keep gold prices relatively supported. However, the strength of the U.S. dollar and the potential for interest rate hikes introduce downside risks. Therefore, a sideways to slightly upward trend is anticipated. Key risks to this prediction include a faster-than-expected decline in inflation that would diminish gold's safe-haven appeal. Also, unexpected diplomatic breakthroughs resolving geopolitical conflicts would also decrease prices. Lastly, a major surprise or shift in monetary policy from the Fed could also significantly impact the gold index. Careful monitoring of these risk factors and market adjustments will be important for investors.
Rating | Short-Term | Long-Term Senior |
---|---|---|
Outlook | B2 | Ba3 |
Income Statement | Ba2 | Caa2 |
Balance Sheet | Baa2 | Caa2 |
Leverage Ratios | C | Baa2 |
Cash Flow | C | Baa2 |
Rates of Return and Profitability | B3 | B2 |
*An aggregate rating for an index summarizes the overall sentiment towards the companies it includes. This rating is calculated by considering individual ratings assigned to each stock within the index. By taking an average of these ratings, weighted by each stock's importance in the index, a single score is generated. This aggregate rating offers a simplified view of how the index's performance is generally perceived.
How does neural network examine financial reports and understand financial state of the company?
References
- Firth JR. 1957. A synopsis of linguistic theory 1930–1955. In Studies in Linguistic Analysis (Special Volume of the Philological Society), ed. JR Firth, pp. 1–32. Oxford, UK: Blackwell
- Wan M, Wang D, Goldman M, Taddy M, Rao J, et al. 2017. Modeling consumer preferences and price sensitiv- ities from large-scale grocery shopping transaction logs. In Proceedings of the 26th International Conference on the World Wide Web, pp. 1103–12. New York: ACM
- Thomas P, Brunskill E. 2016. Data-efficient off-policy policy evaluation for reinforcement learning. In Pro- ceedings of the International Conference on Machine Learning, pp. 2139–48. La Jolla, CA: Int. Mach. Learn. Soc.
- Armstrong, J. S. M. C. Grohman (1972), "A comparative study of methods for long-range market forecasting," Management Science, 19, 211–221.
- Thomas P, Brunskill E. 2016. Data-efficient off-policy policy evaluation for reinforcement learning. In Pro- ceedings of the International Conference on Machine Learning, pp. 2139–48. La Jolla, CA: Int. Mach. Learn. Soc.
- Ruiz FJ, Athey S, Blei DM. 2017. SHOPPER: a probabilistic model of consumer choice with substitutes and complements. arXiv:1711.03560 [stat.ML]
- A. Tamar and S. Mannor. Variance adjusted actor critic algorithms. arXiv preprint arXiv:1310.3697, 2013.