AUC Score :
Short-term Tactic1 :
Dominant Strategy :
Time series to forecast n:
ML Model Testing : Modular Neural Network (Speculative Sentiment Analysis)
Hypothesis Testing : Linear Regression
Surveillance : Major exchange and OTC
1Short-term revised.
2Time series is updated based on short-term trends.
Key Points
The China A50 Index is projected to experience moderate volatility, with an overall bullish trend anticipated. Increased government stimulus and economic recovery efforts are likely to provide support, potentially leading to gains. However, the index faces risks stemming from the global economic slowdown, trade tensions, and the ongoing property sector challenges. Further weakening of the yuan currency could also negatively impact the index. Geopolitical uncertainties remain a key factor, capable of generating sudden and significant market corrections.About China A50 Index
The FTSE China A50 Index is a benchmark index that tracks the performance of the top 50 largest and most liquid stocks listed on the Shanghai and Shenzhen stock exchanges. These companies represent a significant portion of the overall market capitalization of the A-share market, providing a broad reflection of the performance of the Chinese economy. The index is designed to be readily investable, allowing institutional investors to gain exposure to the Chinese equity market through various financial instruments, such as futures and exchange-traded funds (ETFs). The constituents are selected based on a combination of market capitalization and liquidity criteria, ensuring that the index accurately reflects the performance of the most influential companies in China.
The FTSE China A50 Index is often used as a key indicator of investor sentiment towards the Chinese market. Its performance is closely watched by global investors and financial analysts as it provides valuable insights into the economic health and growth prospects of China. Changes in the index are heavily influenced by factors such as government policies, macroeconomic data releases, and shifts in global market conditions. Consequently, fluctuations in the China A50 index can be highly sensitive to political developments and economic news, and are frequently used as a tool for analyzing trends and making investment decisions in Chinese equities.
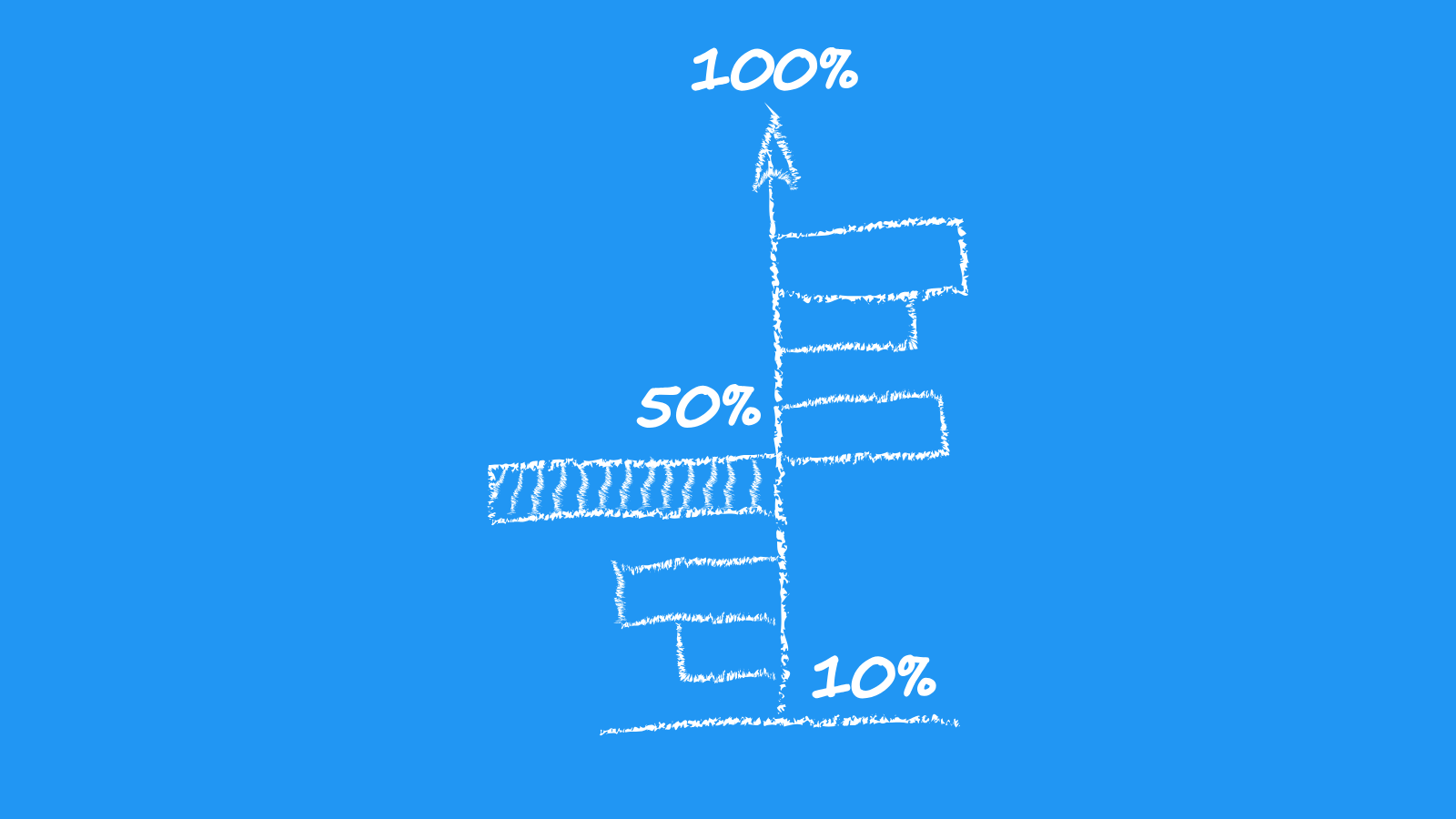
China A50 Index Forecasting Model
Our team, comprised of data scientists and economists, has developed a machine learning model to forecast the China A50 index. The model leverages a comprehensive dataset encompassing a wide range of factors, including macroeconomic indicators such as GDP growth, inflation rates, and industrial production, and market-specific variables like trading volume, volatility, and investor sentiment proxies. Additionally, the model incorporates global economic data, considering the interconnectedness of financial markets, including commodity prices (crude oil, iron ore), interest rates (US Treasury yields), and major international stock market indices. Data preprocessing involves cleaning, handling missing values, and feature engineering, such as creating lagged variables and rolling averages to capture time-series dependencies. This preprocessing is essential for ensuring data quality and enabling the model to learn from historical patterns effectively.
We explored several machine learning algorithms to identify the most accurate and robust forecasting model. These included Recurrent Neural Networks (RNNs) with Long Short-Term Memory (LSTM) cells, known for their ability to capture temporal dependencies in sequential data, and gradient boosting machines (GBMs) like XGBoost and LightGBM, which are renowned for their predictive power and efficiency. Feature importance analysis, conducted after model training, allows us to identify the key drivers influencing the A50 index. We also implemented techniques like hyperparameter tuning using grid search and cross-validation to optimize model performance. Rigorous backtesting, involving evaluating the model's performance on historical data outside the training period, has been performed to gauge the model's predictive accuracy and stability. Furthermore, this model is regularly retrained with new data to maintain its predictive performance.
Our model's output provides a probabilistic forecast of the China A50 index movement over a specified time horizon. The forecast includes point estimates and associated confidence intervals. It supports traders and investors by providing insight and assisting risk management. Our model is designed to be dynamic, allowing for the regular incorporation of new data and model refinement to ensure its adaptability and maintain forecasting accuracy. The model's output is interpreted in the context of current market conditions and other relevant information and is not a substitute for due diligence and professional investment advice. We continue to improve and refine our model by incorporating more comprehensive data and new advances in machine learning technology.
ML Model Testing
n:Time series to forecast
p:Price signals of China A50 index
j:Nash equilibria (Neural Network)
k:Dominated move of China A50 index holders
a:Best response for China A50 target price
For further technical information as per how our model work we invite you to visit the article below:
How do KappaSignal algorithms actually work?
China A50 Index Forecast Strategic Interaction Table
Strategic Interaction Table Legend:
X axis: *Likelihood% (The higher the percentage value, the more likely the event will occur.)
Y axis: *Potential Impact% (The higher the percentage value, the more likely the price will deviate.)
Z axis (Grey to Black): *Technical Analysis%
China A50 Index Financial Outlook and Forecast
The China A50 Index, representing the largest and most liquid stocks listed on the Shanghai and Shenzhen Stock Exchanges, faces a complex and multifaceted outlook. Its performance is deeply intertwined with the trajectory of the Chinese economy, influenced by factors such as government policies, global trade dynamics, and investor sentiment. Significant state intervention and regulatory changes within key sectors, including technology, real estate, and education, have previously created volatility. Moreover, the ongoing geopolitical tensions, especially those concerning trade with the United States and its allies, pose a continuous headwind for the index. Analyzing macroeconomic indicators such as GDP growth, inflation rates, and manufacturing activity is crucial in gauging the index's future direction. The performance of this index is also linked with the global economic environment which indirectly influences the investment climate of the domestic markets.
Several factors are expected to shape the China A50 Index's performance in the coming years. The government's strategic initiatives, such as investments in infrastructure, technology, and renewable energy, could provide a substantial boost, particularly for specific sectors represented within the index. The strength of domestic consumption is another critical element, as a robust consumer market can drive corporate earnings and overall market growth. However, the real estate sector, which contributes significantly to the Chinese economy, warrants careful monitoring, given the ongoing issues with debt and potential property market correction. The continued easing of monetary policies, including interest rate adjustments and reserve requirement reductions, could also stimulate economic activity and market liquidity. Furthermore, the progress of the technological advancements may have an impact, driving the economy.
Key challenges to the China A50 Index's upward trajectory include persistent geopolitical risks, as any escalation in trade disputes or diplomatic tensions could negatively impact investor confidence and international trade flows. Moreover, the deleveraging efforts within the property sector and the potential for further regulatory tightening pose significant downside risks. The fluctuating global economic conditions, including potential recessions in major economies, may also have a ripple effect on the Chinese economy. Furthermore, the volatility that could be caused by government policies can affect the future of index. The pace and effectiveness of China's economic reforms and the ability of its companies to adapt to global market changes will significantly determine the index's long-term success. The potential for increased inflation and the management of the debt can also influence.
Looking ahead, the China A50 Index is projected to experience moderate growth over the next several years, buoyed by government support and the nation's economic reforms. This prediction is based on the expectation that the current government policies, aimed at stimulating the economy and the continued recovery from the economic effects of the recent past events will be effective. However, the risks to this outlook remain significant. Geopolitical tensions, a potential slowdown in the real estate sector, and any unexpected regulatory changes could hinder the growth. Investors should closely monitor macroeconomic indicators, corporate earnings, and global market trends to assess the risks and opportunities associated with the China A50 Index.
```
Rating | Short-Term | Long-Term Senior |
---|---|---|
Outlook | B2 | B1 |
Income Statement | Baa2 | Baa2 |
Balance Sheet | C | C |
Leverage Ratios | C | Caa2 |
Cash Flow | B2 | Baa2 |
Rates of Return and Profitability | Ba2 | B2 |
*An aggregate rating for an index summarizes the overall sentiment towards the companies it includes. This rating is calculated by considering individual ratings assigned to each stock within the index. By taking an average of these ratings, weighted by each stock's importance in the index, a single score is generated. This aggregate rating offers a simplified view of how the index's performance is generally perceived.
How does neural network examine financial reports and understand financial state of the company?
References
- Chamberlain G. 2000. Econometrics and decision theory. J. Econom. 95:255–83
- Swaminathan A, Joachims T. 2015. Batch learning from logged bandit feedback through counterfactual risk minimization. J. Mach. Learn. Res. 16:1731–55
- Jacobs B, Donkers B, Fok D. 2014. Product Recommendations Based on Latent Purchase Motivations. Rotterdam, Neth.: ERIM
- Hill JL. 2011. Bayesian nonparametric modeling for causal inference. J. Comput. Graph. Stat. 20:217–40
- T. Morimura, M. Sugiyama, M. Kashima, H. Hachiya, and T. Tanaka. Nonparametric return distribution ap- proximation for reinforcement learning. In Proceedings of the 27th International Conference on Machine Learning, pages 799–806, 2010
- Friedberg R, Tibshirani J, Athey S, Wager S. 2018. Local linear forests. arXiv:1807.11408 [stat.ML]
- Jorgenson, D.W., Weitzman, M.L., ZXhang, Y.X., Haxo, Y.M. and Mat, Y.X., 2023. Apple's Stock Price: How News Affects Volatility. AC Investment Research Journal, 220(44).