AUC Score :
Short-term Tactic1 :
Dominant Strategy :
Time series to forecast n:
ML Model Testing : Deductive Inference (ML)
Hypothesis Testing : Statistical Hypothesis Testing
Surveillance : Major exchange and OTC
1Short-term revised.
2Time series is updated based on short-term trends.
Key Points
CRC faces a mixed outlook. Production levels are expected to remain relatively stable, driven by existing assets and ongoing operational efficiencies. However, fluctuations in oil and natural gas prices pose a significant risk, as revenue and profitability are directly tied to commodity market dynamics; a downturn in prices could substantially impact CRC's financial performance. Furthermore, the company's heavy debt load presents a continuing challenge, increasing financial risk. Regulatory changes and environmental policies, especially concerning emissions and carbon reduction, could add costs and limit future development opportunities.About California Resources Corporation
California Resources Corp (CRC) is a publicly traded oil and natural gas exploration and production company. CRC operates primarily in California, holding a significant portfolio of onshore assets. The company focuses on the development and extraction of crude oil and natural gas reserves, employing various drilling and production techniques. CRC's operations contribute substantially to California's energy supply, focusing on sustainable and responsible practices within the state's regulatory framework. It navigates environmental regulations and market fluctuations common in the energy sector.
CRC emphasizes operational efficiency and cost management across its assets. The company aims to optimize production while investing in technologies to improve environmental performance and reduce its carbon footprint. It routinely engages in strategic planning to maintain and enhance its financial position amidst the dynamic energy industry. Further, CRC is subject to evolving environmental policies and regulations, which shape its operational strategies and capital investments within California.
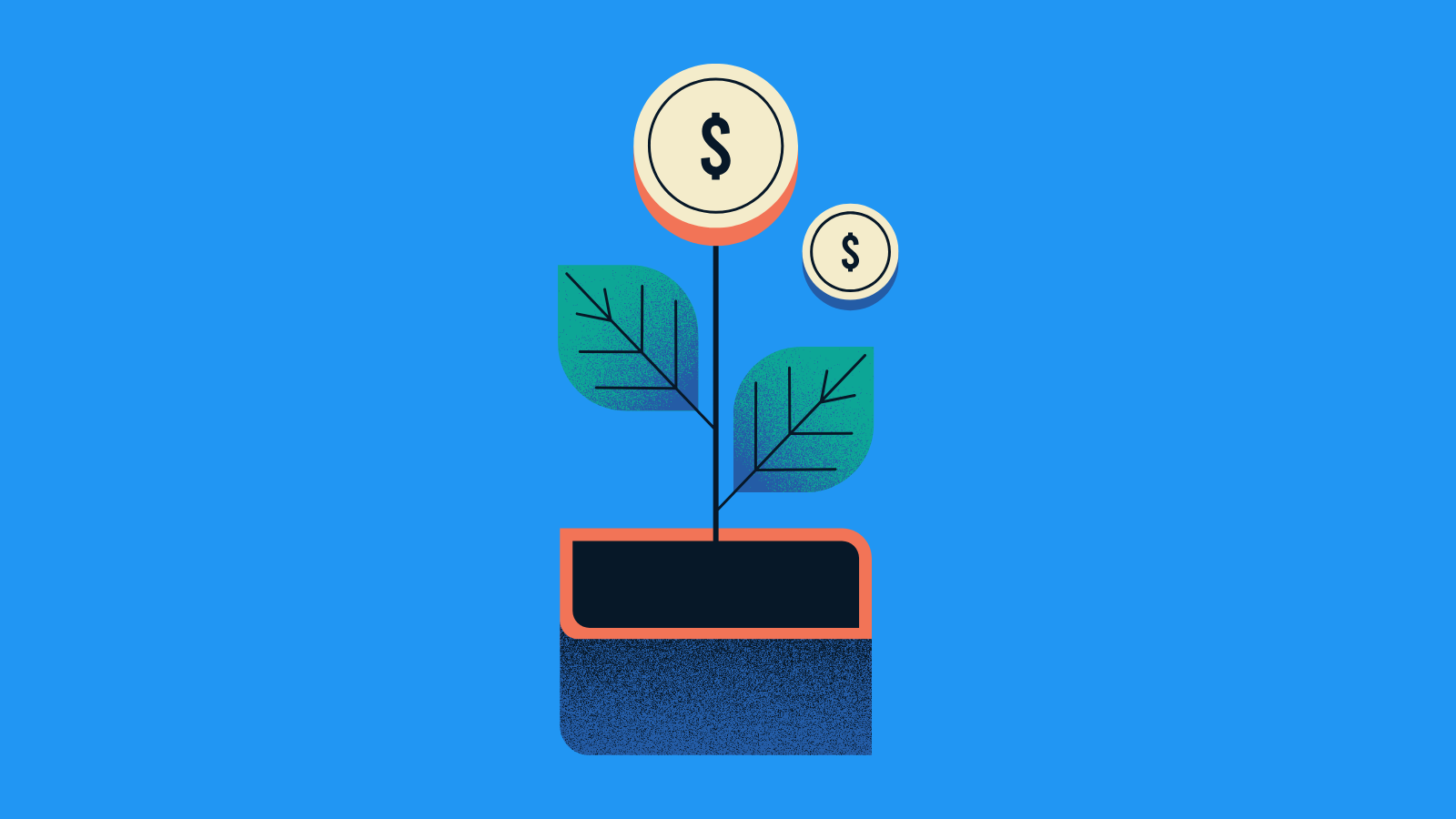
CRC Stock Forecast Model
As a team of data scientists and economists, we propose a comprehensive machine learning model to forecast the performance of California Resources Corporation (CRC) common stock. Our approach integrates diverse data sources, encompassing both internal company data and external macroeconomic indicators. Internal data includes CRC's quarterly and annual financial statements, production volumes, operating expenses, and exploration activities. External data will involve industry-specific indicators like crude oil prices, natural gas prices, refining margins, and the overall energy sector indices. Furthermore, we intend to incorporate macroeconomic variables such as GDP growth, inflation rates, interest rates, and exchange rates to capture the broader economic environment's influence on CRC's performance. The model will be designed to incorporate all of these factors to provide the most accurate forecast possible.
Our machine learning model will leverage several advanced techniques. We will explore time series analysis methods like ARIMA and its variants to capture temporal dependencies within the financial data. We will also experiment with advanced machine learning algorithms, including Support Vector Machines (SVMs), Random Forests, and Gradient Boosting Machines (GBMs), to accommodate the complex relationships between various influencing variables. Feature engineering will be a crucial part of the process. This may involve creating lagged variables, calculating moving averages, and exploring ratios to extract relevant information from the raw data. Model selection and hyperparameter tuning will be performed using cross-validation techniques to ensure robustness and generalization ability. Ultimately, the model will be trained on historical data, validated using a held-out dataset, and then used to forecast the stock's future performance.
The final model will deliver forecasts over multiple time horizons (e.g., daily, weekly, monthly). The output will include the predicted CRC stock performance in different categories (e.g. price movement direction or expected changes). The model will also provide confidence intervals to quantify the uncertainty associated with the predictions. Regular evaluation and refinement of the model will be undertaken to account for changes in market dynamics, including the addition of more current data, and adjusting the model based on performance evaluation. We will create a model that is highly accurate for forecasting, as well as easy to understand and interpret for stakeholders.
ML Model Testing
n:Time series to forecast
p:Price signals of California Resources Corporation stock
j:Nash equilibria (Neural Network)
k:Dominated move of California Resources Corporation stock holders
a:Best response for California Resources Corporation target price
For further technical information as per how our model work we invite you to visit the article below:
How do KappaSignal algorithms actually work?
California Resources Corporation Stock Forecast (Buy or Sell) Strategic Interaction Table
Strategic Interaction Table Legend:
X axis: *Likelihood% (The higher the percentage value, the more likely the event will occur.)
Y axis: *Potential Impact% (The higher the percentage value, the more likely the price will deviate.)
Z axis (Grey to Black): *Technical Analysis%
Rating | Short-Term | Long-Term Senior |
---|---|---|
Outlook | B3 | Ba3 |
Income Statement | Ba3 | B3 |
Balance Sheet | B3 | Ba3 |
Leverage Ratios | B2 | Baa2 |
Cash Flow | C | B3 |
Rates of Return and Profitability | B3 | Baa2 |
*Financial analysis is the process of evaluating a company's financial performance and position by neural network. It involves reviewing the company's financial statements, including the balance sheet, income statement, and cash flow statement, as well as other financial reports and documents.
How does neural network examine financial reports and understand financial state of the company?
References
- M. Colby, T. Duchow-Pressley, J. J. Chung, and K. Tumer. Local approximation of difference evaluation functions. In Proceedings of the Fifteenth International Joint Conference on Autonomous Agents and Multiagent Systems, Singapore, May 2016
- Dietterich TG. 2000. Ensemble methods in machine learning. In Multiple Classifier Systems: First International Workshop, Cagliari, Italy, June 21–23, pp. 1–15. Berlin: Springer
- E. Collins. Using Markov decision processes to optimize a nonlinear functional of the final distribution, with manufacturing applications. In Stochastic Modelling in Innovative Manufacturing, pages 30–45. Springer, 1997
- Mikolov T, Yih W, Zweig G. 2013c. Linguistic regularities in continuous space word representations. In Pro- ceedings of the 2013 Conference of the North American Chapter of the Association for Computational Linguistics: Human Language Technologies, pp. 746–51. New York: Assoc. Comput. Linguist.
- Nie X, Wager S. 2019. Quasi-oracle estimation of heterogeneous treatment effects. arXiv:1712.04912 [stat.ML]
- M. J. Hausknecht. Cooperation and Communication in Multiagent Deep Reinforcement Learning. PhD thesis, The University of Texas at Austin, 2016
- R. Sutton, D. McAllester, S. Singh, and Y. Mansour. Policy gradient methods for reinforcement learning with function approximation. In Proceedings of Advances in Neural Information Processing Systems 12, pages 1057–1063, 2000