AUC Score :
Short-term Tactic1 :
Dominant Strategy :
Time series to forecast n:
ML Model Testing : Reinforcement Machine Learning (ML)
Hypothesis Testing : Spearman Correlation
Surveillance : Major exchange and OTC
1Short-term revised.
2Time series is updated based on short-term trends.
Key Points
Arvinas is poised for significant growth driven by its PROTAC platform and pipeline. Success in late-stage clinical trials for its oncology programs, particularly those targeting breast and prostate cancers, would likely lead to substantial revenue increases and solidify its market position. However, clinical trial failures or delays for its lead candidates represent a major risk that could severely impact the stock's valuation. Furthermore, increased competition from other companies developing PROTACs, or alternative targeted protein degradation technologies, presents additional challenges to Arvinas' long-term success. The company's ability to secure partnerships and effectively manage its cash flow are also critical factors influencing future performance.About Arvinas Inc.
Arvinas is a biotechnology company focused on developing novel therapies based on its PROTAC® (Proteolysis Targeting Chimera) technology platform. This platform utilizes a cell's natural protein disposal system to degrade disease-causing proteins. The company's approach aims to address challenging disease targets and offer potential advantages over traditional small molecule drugs and antibody therapies. Arvinas has a pipeline of investigational medicines targeting a variety of cancers and neurological disorders, including breast cancer, prostate cancer, and Alzheimer's disease.
The company's strategy revolves around advancing its proprietary PROTAC® platform, discovering new drug candidates, and progressing them through clinical development. Arvinas collaborates with other pharmaceutical companies to expand its reach and commercialize its therapies. Research and development are key aspects of the company's activities, requiring significant investment in scientific innovation and clinical trials. The company is continually focused on the development and commercialization of its therapeutics to provide new options for patients.
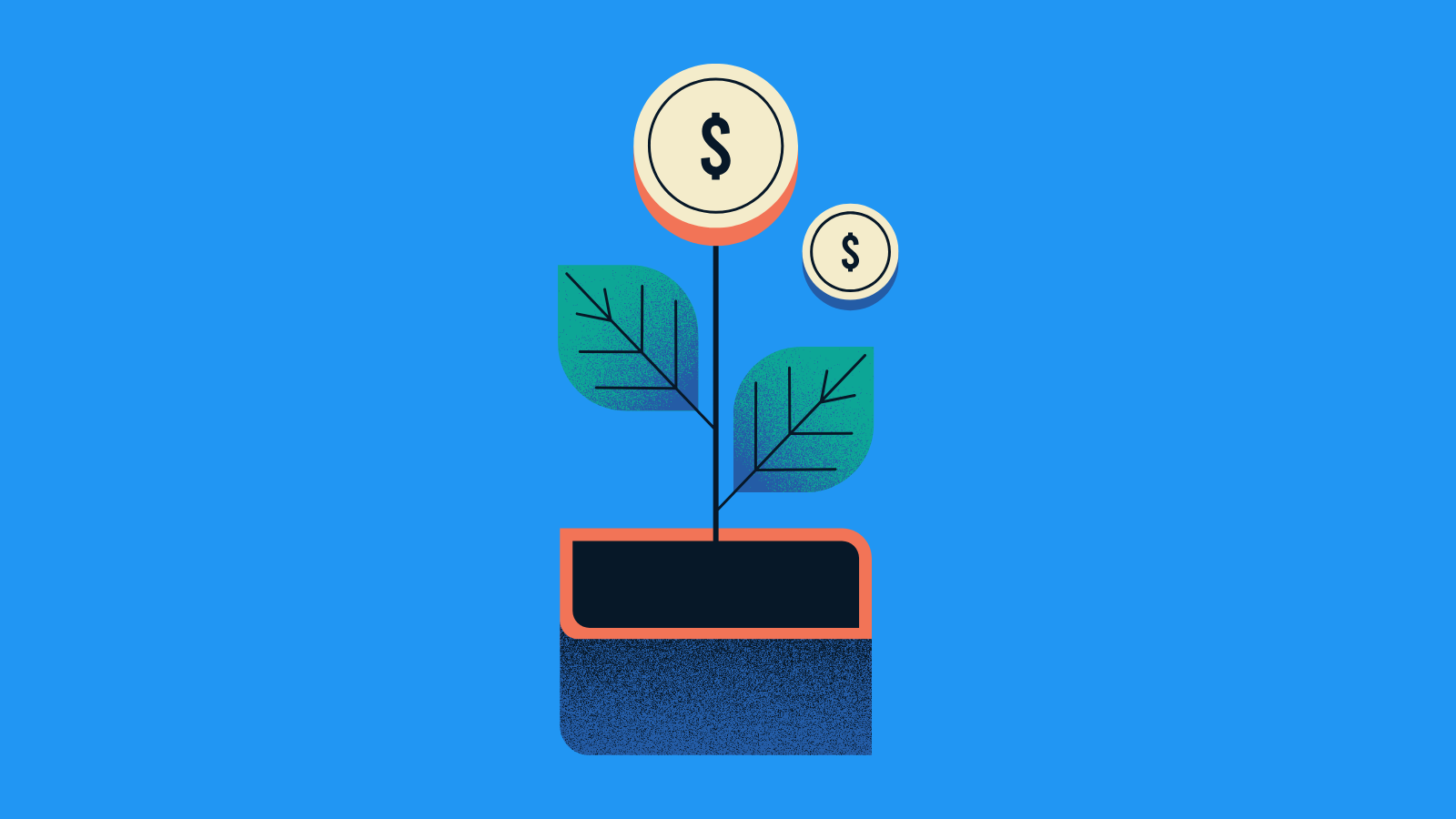
ARVN Stock Forecasting Model
Our interdisciplinary team has developed a machine learning model for forecasting Arvinas Inc. (ARVN) stock performance. This model leverages a combination of time-series analysis, fundamental analysis, and sentiment analysis techniques. The time-series component incorporates historical trading data, including daily trading volume, moving averages, and momentum indicators, to identify patterns and trends. Fundamental analysis incorporates financial statements, such as revenue, earnings per share (EPS), debt levels, and cash flow, to assess the company's underlying financial health and valuation. Sentiment analysis analyzes news articles, social media posts, and analyst reports to gauge market sentiment and its potential impact on ARVN stock.
The machine learning algorithm used is a hybrid model integrating both Long Short-Term Memory (LSTM) networks and gradient boosting. LSTM networks are employed to capture complex temporal dependencies in the time-series data, allowing the model to understand the dynamics of past trends and predict future behavior. Gradient boosting models, such as XGBoost, are used to incorporate fundamental and sentiment factors, helping the model to understand how those factors influence stock movement. Data preprocessing is crucial, with the use of techniques such as feature scaling, data cleaning, and handling of missing values. Regularization techniques, such as L1 and L2 regularization, are employed to prevent overfitting and improve generalization performance.
The model's output is a probabilistic forecast for ARVN stock, providing an estimated probability of the stock rising, falling, or staying the same over a specified time horizon. The model's performance is continuously monitored and refined by utilizing metrics such as Mean Absolute Error (MAE), Root Mean Squared Error (RMSE), and Sharpe Ratio. Backtesting is also done on historical data to validate the model's performance. This model provides actionable insights and aids in a more data-driven approach to decision making, contributing to our analysis to assess ARVN's investment potential.
```
ML Model Testing
n:Time series to forecast
p:Price signals of Arvinas Inc. stock
j:Nash equilibria (Neural Network)
k:Dominated move of Arvinas Inc. stock holders
a:Best response for Arvinas Inc. target price
For further technical information as per how our model work we invite you to visit the article below:
How do KappaSignal algorithms actually work?
Arvinas Inc. Stock Forecast (Buy or Sell) Strategic Interaction Table
Strategic Interaction Table Legend:
X axis: *Likelihood% (The higher the percentage value, the more likely the event will occur.)
Y axis: *Potential Impact% (The higher the percentage value, the more likely the price will deviate.)
Z axis (Grey to Black): *Technical Analysis%
Arvinas Inc. (ARVN) Financial Outlook and Forecast
The financial outlook for ARVN appears promising, driven by its innovative protein degradation platform and a pipeline of novel therapies targeting previously undruggable diseases. The company's core strategy centers on developing targeted protein degraders (TPDs), which utilize the body's own cellular machinery to eliminate disease-causing proteins. This approach offers the potential for greater efficacy and improved safety compared to traditional small molecule inhibitors. ARVN's focus on oncology, particularly in areas like breast cancer, prostate cancer, and lung cancer, positions it in a market with significant unmet medical needs and substantial commercial opportunities. The company's clinical trials, including its lead product candidate, vepdegestrant, for breast cancer, have demonstrated encouraging preliminary results, fueling optimism for its future success. The robust pipeline, coupled with strategic partnerships, provides a foundation for sustained growth.
ARVN's financial forecasts are anticipated to experience substantial increases in the coming years. The realization of revenue growth will be dependent on the progress and potential commercialization of its clinical programs. Successful clinical trials and regulatory approvals of its lead programs are poised to generate significant revenue streams through product sales and potential royalties from partnerships. Further, ARVN's collaboration agreements with major pharmaceutical companies provide financial stability through upfront payments, milestones, and royalty arrangements. These partnerships not only provide capital but also contribute to expertise and resources in clinical development and commercialization. While ARVN is currently operating at a loss due to substantial research and development expenditures, the anticipated revenue from product sales and partnership milestones are expected to help the company reach profitability. Investors must consider the expected increases in research and development expenditures as clinical trials progress, with subsequent commercialization costs adding pressure on financial performance in the short-term.
Financial stability and strategic actions will also play a major role. Management's ability to effectively navigate clinical development, secure regulatory approvals, and execute successful commercialization strategies will be critical. ARVN's current cash position, bolstered by previous financing rounds and collaborations, provides a solid financial foundation for its operations. Diligent management of expenses, including a focus on controlling operating costs, would be beneficial for long-term financial success. Strategic decisions regarding partnerships, acquisitions, and pipeline prioritization would also have a major impact on the financial trajectory. Maintaining a strong cash position is key to mitigating risks associated with clinical development and potential setbacks. Further, the company's ability to secure additional financing, if required, will be important for supporting its long-term strategic goals, especially in the absence of near-term profitability.
The outlook for ARVN is positive, based on its innovative platform, promising clinical pipeline, and partnerships. The company is poised to become a major player in the oncology and protein degradation space. It is predicted that the company will witness long-term revenue growth and financial returns for investors. However, this forecast is subject to inherent risks. The primary risk is the inherent uncertainty involved in clinical trials, with potential for setbacks, trial failures, or delays. Regulatory hurdles, manufacturing challenges, and potential competition from other protein degradation companies are additional threats to the company's success. Furthermore, ARVN's success depends on its ability to commercialize its products effectively. While progress is encouraging, investors should cautiously consider all associated risks before deciding to invest.
Rating | Short-Term | Long-Term Senior |
---|---|---|
Outlook | Ba3 | Baa2 |
Income Statement | C | Baa2 |
Balance Sheet | B2 | Ba3 |
Leverage Ratios | B2 | Baa2 |
Cash Flow | Baa2 | Ba3 |
Rates of Return and Profitability | Baa2 | Baa2 |
*Financial analysis is the process of evaluating a company's financial performance and position by neural network. It involves reviewing the company's financial statements, including the balance sheet, income statement, and cash flow statement, as well as other financial reports and documents.
How does neural network examine financial reports and understand financial state of the company?
References
- H. Kushner and G. Yin. Stochastic approximation algorithms and applications. Springer, 1997.
- Ruiz FJ, Athey S, Blei DM. 2017. SHOPPER: a probabilistic model of consumer choice with substitutes and complements. arXiv:1711.03560 [stat.ML]
- Dudik M, Langford J, Li L. 2011. Doubly robust policy evaluation and learning. In Proceedings of the 28th International Conference on Machine Learning, pp. 1097–104. La Jolla, CA: Int. Mach. Learn. Soc.
- Farrell MH, Liang T, Misra S. 2018. Deep neural networks for estimation and inference: application to causal effects and other semiparametric estimands. arXiv:1809.09953 [econ.EM]
- Blei DM, Lafferty JD. 2009. Topic models. In Text Mining: Classification, Clustering, and Applications, ed. A Srivastava, M Sahami, pp. 101–24. Boca Raton, FL: CRC Press
- Breusch, T. S. A. R. Pagan (1979), "A simple test for heteroskedasticity and random coefficient variation," Econometrica, 47, 1287–1294.
- Bai J, Ng S. 2002. Determining the number of factors in approximate factor models. Econometrica 70:191–221