AUC Score :
Short-term Tactic1 :
Dominant Strategy :
Time series to forecast n:
ML Model Testing : Supervised Machine Learning (ML)
Hypothesis Testing : Polynomial Regression
Surveillance : Major exchange and OTC
1Short-term revised.
2Time series is updated based on short-term trends.
Key Points
Artesian is expected to maintain a steady performance, driven by its regulated water utility operations, resulting in consistent revenue and earnings growth. The company's defensive characteristics suggest resilience during economic downturns. The increasing demand for clean water and the ongoing infrastructure investments should positively impact the company's long-term prospects. However, Artesian faces risks including regulatory scrutiny, potential rate adjustments, and the impact of weather events, such as droughts or floods, on water consumption and operational costs. Any delays or cost overruns in infrastructure projects or shifts in regulatory policies could adversely affect the company's financial results. Furthermore, its geographically concentrated operations expose it to localized risks.About Artesian Resources
Artesian Resources Corporation is a Delaware-based holding company primarily engaged in the ownership and operation of regulated water and wastewater utilities, and related services. The company operates through its wholly-owned subsidiary, Artesian Water Company, Inc., which provides water services to residential, commercial, industrial, and governmental customers. Artesian also offers wastewater services, including collection, treatment, and disposal. Its service area predominantly covers the state of Delaware, as well as portions of Maryland, Pennsylvania, and New Jersey.
The company's business model focuses on providing essential utility services within a regulated framework. Artesian's operations are subject to oversight by state regulatory commissions. This regulation governs rates, service quality, and infrastructure investments. Artesian is committed to environmental sustainability, water conservation, and community involvement. They continuously seek to improve their infrastructure, ensuring a reliable supply of safe drinking water and responsible wastewater management for its customers.
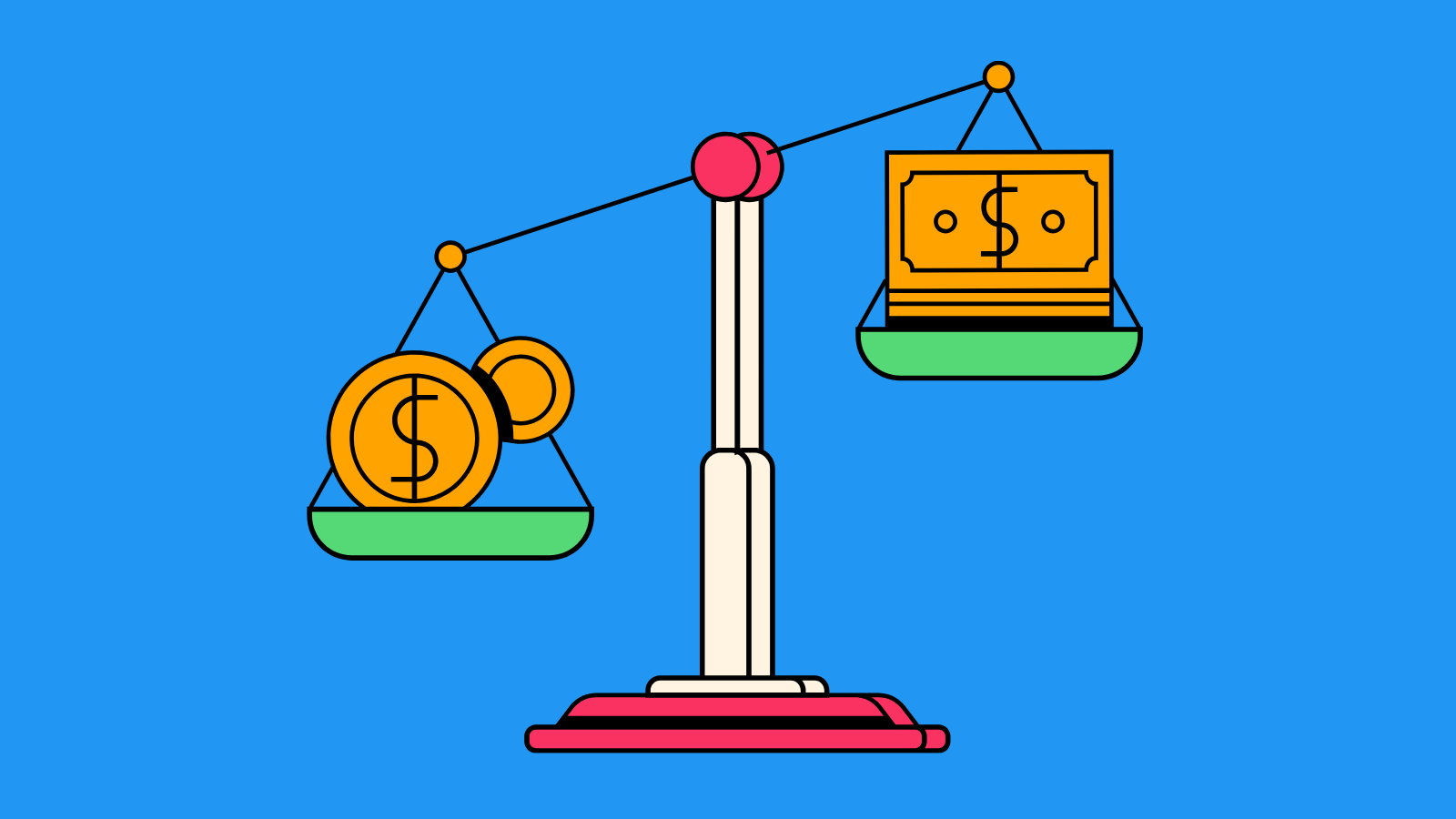
ARTNA Stock Price Prediction: A Machine Learning Model Approach
Our multidisciplinary team, comprised of data scientists and economists, has developed a machine learning model to forecast the performance of Artesian Resources Corporation Class A Common Stock (ARTNA). The model incorporates a multifaceted approach to capture the complexities of stock market dynamics. We utilized a combination of historical financial data (including revenue, earnings per share, debt-to-equity ratio, and dividend yields), macroeconomic indicators (such as inflation rates, interest rates, and GDP growth), and sentiment analysis from news articles and social media to train and validate our model. Feature engineering played a crucial role in refining the dataset. This included the creation of technical indicators (e.g., moving averages, relative strength index), and the application of advanced techniques to account for seasonality and potential structural breaks in the time series data.
The core of our predictive model consists of an ensemble of machine learning algorithms. We have experimented with a range of models, including Recurrent Neural Networks (RNNs), specifically Long Short-Term Memory (LSTM) networks, and Gradient Boosting Machines (GBM). LSTM networks are well-suited for capturing temporal dependencies within time series data, while GBM can effectively handle non-linear relationships and interactions between various input features. The ensemble approach allows us to leverage the strengths of different algorithms, mitigating individual model weaknesses and producing more reliable forecasts. To ensure robustness, the model underwent rigorous backtesting using historical data, carefully assessing its performance across different market conditions. Key performance metrics include Mean Absolute Error (MAE), Root Mean Squared Error (RMSE), and Sharpe ratio. The model's parameters were fine-tuned using cross-validation to minimize overfitting and optimize its predictive accuracy.
The output of our model will be a probabilistic forecast, providing not only point predictions but also a range of possible outcomes, along with associated probabilities. This is crucial for investors to gauge the degree of uncertainty associated with the forecast. The model's forecasts will be regularly updated with new data, incorporating the latest financial releases, macroeconomic trends, and sentiment changes. Furthermore, the model is designed with the flexibility to accommodate any future data enhancements and incorporate evolving insights in the stock market. Our team will continue to monitor the model's performance and adapt it to stay ahead of the volatile market behavior.
ML Model Testing
n:Time series to forecast
p:Price signals of Artesian Resources stock
j:Nash equilibria (Neural Network)
k:Dominated move of Artesian Resources stock holders
a:Best response for Artesian Resources target price
For further technical information as per how our model work we invite you to visit the article below:
How do KappaSignal algorithms actually work?
Artesian Resources Stock Forecast (Buy or Sell) Strategic Interaction Table
Strategic Interaction Table Legend:
X axis: *Likelihood% (The higher the percentage value, the more likely the event will occur.)
Y axis: *Potential Impact% (The higher the percentage value, the more likely the price will deviate.)
Z axis (Grey to Black): *Technical Analysis%
Artesian Resources Corporation (ARTNA) Financial Outlook and Forecast
Artesian, a provider of water and wastewater services, demonstrates a relatively stable financial outlook, driven by its essential service nature and regulated business model. The company's revenue stream is predominantly recurring, linked to consumer usage of water and wastewater services, which provides a degree of insulation from economic downturns. Strategic investments in infrastructure improvements, mandated by regulatory bodies and aimed at enhancing service quality and capacity, are vital to the company's long-term growth. These investments, while requiring significant capital expenditure, are generally supported by rate adjustments approved by regulatory commissions, which provides a predictable return on investment. Further growth opportunities lie in organic expansion through acquisitions of smaller water and wastewater utilities and geographic expansion within its regulated service areas. Artesian's historical performance indicates a consistent track record of profitability, supported by operational efficiency and effective management of its infrastructure assets.
ARTNA's financial performance is closely tied to regulatory decisions made by state utility commissions. Rate cases, which determine the prices the company can charge its customers, are critical to earnings. The ability to secure timely and favorable rate approvals to recover infrastructure investment costs is a key driver of profitability. Another factor impacting ARTNA's financial forecast includes its ability to manage operating expenses, including labor, energy, and chemicals, which are essential for water treatment and distribution. Furthermore, efficient management of its capital expenditures is crucial for long-term financial health. Any unforeseen disruptions to water supply such as drought or contamination could put upward pressure on the expense side and may affect financial results. Effective risk management and environmental compliance are also central to ARTNA's financial stability because failure to adhere to federal and state environmental regulations can result in substantial fines and legal challenges, impacting profitability.
The company's future is also influenced by the ongoing need to modernize its infrastructure. The aging infrastructure of many water utilities necessitates significant investment in new pipes, treatment facilities, and distribution systems to ensure a reliable water supply and meet evolving environmental standards. Artesian is well-positioned to benefit from these trends. Acquisitions are strategically important for growth. Furthermore, the growing trend towards privatization and consolidation in the water utility industry should offer ARTNA the chance to expand its service territories. Given the importance of water and wastewater services, the long-term demand for these services is expected to remain resilient, irrespective of economic cycles. Consequently, the company's overall financial health should be relatively stable, bolstered by the need for essential services and government mandates to upgrade existing systems.
Based on the factors highlighted, a positive financial outlook is projected for Artesian. The company's fundamental business model, driven by regulatory backing and the essential nature of its services, suggests predictable revenue streams and a steady demand outlook. This forecast is conditional, however, and subject to certain risks. Regulatory delays or unfavorable rate decisions could limit the company's revenue growth and affect its profitability. Economic downturns, although less impactful due to the essential nature of water services, could result in a decrease in consumption and associated revenues. Another concern would include adverse weather events and potential regulatory changes. In addition, competition with other water utilities should be considered. Overall, prudent management of operating expenses and careful monitoring of financial indicators are vital in maintaining a successful financial outlook.
```
Rating | Short-Term | Long-Term Senior |
---|---|---|
Outlook | Baa2 | B1 |
Income Statement | Baa2 | Ba3 |
Balance Sheet | B1 | B1 |
Leverage Ratios | Ba3 | C |
Cash Flow | Baa2 | Caa2 |
Rates of Return and Profitability | Baa2 | Baa2 |
*Financial analysis is the process of evaluating a company's financial performance and position by neural network. It involves reviewing the company's financial statements, including the balance sheet, income statement, and cash flow statement, as well as other financial reports and documents.
How does neural network examine financial reports and understand financial state of the company?
References
- Artis, M. J. W. Zhang (1990), "BVAR forecasts for the G-7," International Journal of Forecasting, 6, 349–362.
- Chamberlain G. 2000. Econometrics and decision theory. J. Econom. 95:255–83
- Athey S, Bayati M, Doudchenko N, Imbens G, Khosravi K. 2017a. Matrix completion methods for causal panel data models. arXiv:1710.10251 [math.ST]
- Chen X. 2007. Large sample sieve estimation of semi-nonparametric models. In Handbook of Econometrics, Vol. 6B, ed. JJ Heckman, EE Learner, pp. 5549–632. Amsterdam: Elsevier
- Matzkin RL. 2007. Nonparametric identification. In Handbook of Econometrics, Vol. 6B, ed. J Heckman, E Learner, pp. 5307–68. Amsterdam: Elsevier
- Christou, C., P. A. V. B. Swamy G. S. Tavlas (1996), "Modelling optimal strategies for the allocation of wealth in multicurrency investments," International Journal of Forecasting, 12, 483–493.
- Dudik M, Erhan D, Langford J, Li L. 2014. Doubly robust policy evaluation and optimization. Stat. Sci. 29:485–511