AUC Score :
Short-term Tactic1 :
Dominant Strategy :
Time series to forecast n:
ML Model Testing : Ensemble Learning (ML)
Hypothesis Testing : Paired T-Test
Surveillance : Major exchange and OTC
1Short-term revised.
2Time series is updated based on short-term trends.
Key Points
Based on current market trends and the company's operational focus, ARCI faces a mixed outlook. A positive prediction suggests the company's strategic investments in infrastructure projects and resource exploration could yield substantial returns, potentially leading to increased revenue and market share. Conversely, risks include dependence on commodity prices, which are inherently volatile, and the inherent regulatory hurdles associated with the mining industry. Furthermore, any project delays or cost overruns could negatively impact financial performance, potentially affecting investor confidence and stock valuation. The company's ability to effectively manage its capital expenditure and adapt to changing market dynamics is crucial for its long-term success.About American Resources Corp.
American Resources Corporation (ARC) is an American company focused on the extraction and processing of metallurgical coal, along with the recovery of rare earth elements and other critical minerals. ARC operates primarily in the Appalachian region of the United States, where it owns and controls a portfolio of metallurgical coal mines and processing facilities. The company aims to supply high-quality coal to the steel industry. ARC emphasizes its commitment to environmentally responsible practices and advanced technologies in its mining operations.
ARC's strategy involves leveraging its existing infrastructure and expertise to expand its production capacity. This includes projects aimed at processing coal more efficiently. They also actively explore opportunities to diversify their revenue streams. ARC is developing initiatives to recover rare earth elements and other valuable minerals from coal waste, contributing to both resource sustainability and economic diversification. ARC aims to position itself as a key player in the metallurgical coal and critical minerals supply chain.
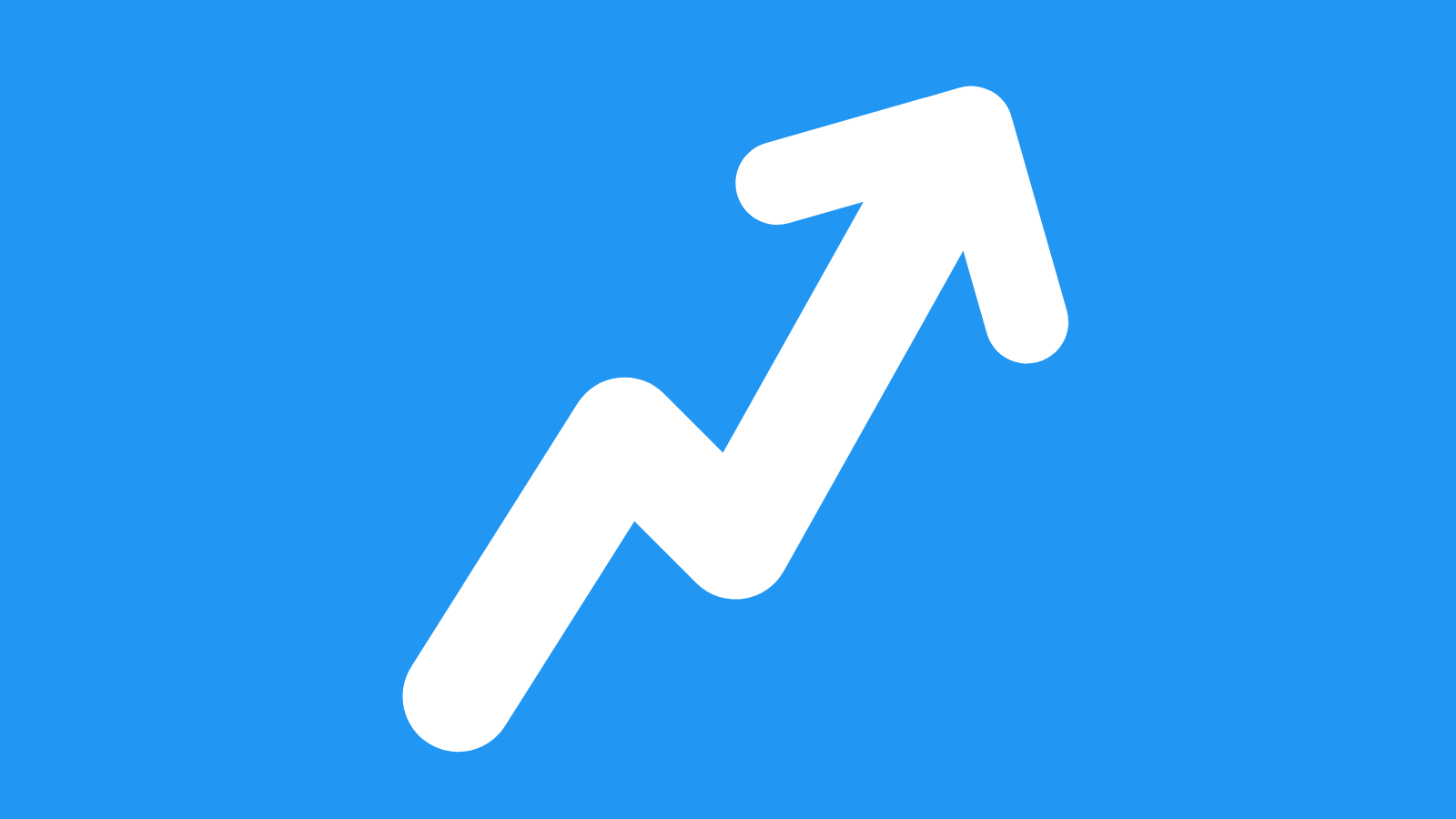
AREC Stock Forecast Machine Learning Model
Our team of data scientists and economists proposes a machine learning model to forecast the performance of American Resources Corporation Class A Common Stock (AREC). The model will leverage a comprehensive dataset encompassing both internal and external factors influencing AREC's value. Key inputs will include historical financial data such as revenue, earnings per share, debt levels, and cash flow. We will also incorporate macroeconomic indicators like GDP growth, inflation rates, interest rates, and commodity prices, given AREC's involvement in resource extraction. Furthermore, we will integrate sector-specific data, focusing on trends within the coal and recycling industries, regulatory changes, and competitor performance. The model will analyze news sentiment from reputable financial sources to gauge investor perception and market dynamics.
The architecture of our forecasting model will employ a combination of machine learning algorithms. We intend to utilize a time-series analysis model like LSTM (Long Short-Term Memory) recurrent neural networks, which are particularly well-suited for capturing temporal dependencies in financial data. This will be complemented by ensemble methods, such as Gradient Boosting Machines or Random Forests, to improve predictive accuracy and robustness. Before training, the data will undergo rigorous preprocessing including cleaning, feature engineering (e.g., moving averages, volatility measures), and normalization. Model performance will be evaluated using established metrics like Mean Squared Error (MSE), Root Mean Squared Error (RMSE), and R-squared, ensuring rigorous assessment and refinement. Cross-validation techniques will be implemented to prevent overfitting and maintain generalization capabilities.
The model's outputs will provide a forecast horizon of a specific period. The results will include a predicted direction (upward or downward) or range and confidence intervals. These forecasts will be coupled with clear visualizations and interpretability features, allowing stakeholders to understand the rationale behind the predictions. The model will be continuously monitored and updated. The model's performance and data inputs will be re-evaluated regularly, incorporating new data and recalibrating parameters as needed. This iterative approach ensures the model remains relevant and adaptable to the ever-changing market environment, providing valuable insights for informed investment decisions regarding AREC stock.
ML Model Testing
n:Time series to forecast
p:Price signals of American Resources Corp. stock
j:Nash equilibria (Neural Network)
k:Dominated move of American Resources Corp. stock holders
a:Best response for American Resources Corp. target price
For further technical information as per how our model work we invite you to visit the article below:
How do KappaSignal algorithms actually work?
American Resources Corp. Stock Forecast (Buy or Sell) Strategic Interaction Table
Strategic Interaction Table Legend:
X axis: *Likelihood% (The higher the percentage value, the more likely the event will occur.)
Y axis: *Potential Impact% (The higher the percentage value, the more likely the price will deviate.)
Z axis (Grey to Black): *Technical Analysis%
Financial Outlook and Forecast for ARC Class A Common Stock
ARC, a company focused on resource extraction and infrastructure projects, presents a cautiously optimistic financial outlook. The firm's primary revenue streams are derived from the production and sale of metallurgical and thermal coal, construction aggregates, and its associated infrastructure services. The company's financial performance is heavily correlated with the global demand for steel and energy, making it vulnerable to fluctuations in commodity prices. Despite these risks, ARC has demonstrated strategic expansion by acquiring and developing strategic resources. This suggests a potential for growth in the coming years, as long as commodity prices stay reasonable. Infrastructure projects, such as the construction of rail lines and port facilities, present additional long-term revenue opportunities. ARC is strategically positioning itself to benefit from increased spending on infrastructure, especially in its target regions.
Forecasting ARC's financial future necessitates considering the intricate interplay of economic factors. Global economic growth, specifically in emerging markets that drive steel demand, significantly influences its coal revenue. Furthermore, the regulatory landscape, which includes environmental regulations on coal production and consumption, is a critical factor. A more aggressive regulatory environment could impose greater compliance costs or limit production capabilities, negatively affecting earnings. The company's debt management and capital expenditure decisions will also play a pivotal role. Prudent financial management will be essential to navigating potential economic downturns and investment opportunities. While capital expenditures for strategic acquisitions and infrastructure projects can lead to higher revenues, these investments can significantly impact short-term profitability and company cash flow, increasing the firm's financial risk.
ARC's ability to maintain competitiveness and to adapt to market dynamics will determine its financial performance. Efficient operations, cost management, and diversification will be crucial. Diversification to non-coal assets such as construction aggregate operations might help to improve stability of company's income, and reduces the risk associated with fluctuations in coal markets. The company also appears to be focused on technological advancements to improve efficiency and lower costs. These actions, combined with strategic partnerships and acquisitions, suggest that the company is proactively attempting to mitigate risks and capture new growth opportunities. The success of these initiatives hinges on the company's ability to execute its strategic plans effectively and respond appropriately to changing market conditions, and the overall trajectory of the global economy.
Overall, the financial outlook for ARC is cautiously positive. The company has the potential for revenue growth driven by infrastructure development, which will have positive impact on future earnings. However, the forecast depends on the global economic growth, commodity prices, and the evolution of energy regulations, all of which carry substantial risks. Adverse movements in these variables could significantly hamper profitability and growth. If ARC can efficiently manage its operations, diversify its revenue streams, and successfully execute its growth strategies, then the company should be able to achieve positive returns and create shareholder value, but continued monitoring of the industry is essential.
Rating | Short-Term | Long-Term Senior |
---|---|---|
Outlook | Caa2 | B2 |
Income Statement | C | Caa2 |
Balance Sheet | C | C |
Leverage Ratios | C | Baa2 |
Cash Flow | B2 | C |
Rates of Return and Profitability | B1 | Baa2 |
*Financial analysis is the process of evaluating a company's financial performance and position by neural network. It involves reviewing the company's financial statements, including the balance sheet, income statement, and cash flow statement, as well as other financial reports and documents.
How does neural network examine financial reports and understand financial state of the company?
References
- O. Bardou, N. Frikha, and G. Pag`es. Computing VaR and CVaR using stochastic approximation and adaptive unconstrained importance sampling. Monte Carlo Methods and Applications, 15(3):173–210, 2009.
- Y. Le Tallec. Robust, risk-sensitive, and data-driven control of Markov decision processes. PhD thesis, Massachusetts Institute of Technology, 2007.
- Semenova V, Goldman M, Chernozhukov V, Taddy M. 2018. Orthogonal ML for demand estimation: high dimensional causal inference in dynamic panels. arXiv:1712.09988 [stat.ML]
- Athey S, Wager S. 2017. Efficient policy learning. arXiv:1702.02896 [math.ST]
- A. Tamar, Y. Glassner, and S. Mannor. Policy gradients beyond expectations: Conditional value-at-risk. In AAAI, 2015
- Breusch, T. S. A. R. Pagan (1979), "A simple test for heteroskedasticity and random coefficient variation," Econometrica, 47, 1287–1294.
- K. Boda and J. Filar. Time consistent dynamic risk measures. Mathematical Methods of Operations Research, 63(1):169–186, 2006