AUC Score :
Short-term Tactic1 :
Dominant Strategy :
Time series to forecast n:
ML Model Testing : Modular Neural Network (Emotional Trigger/Responses Analysis)
Hypothesis Testing : Paired T-Test
Surveillance : Major exchange and OTC
1Short-term revised.
2Time series is updated based on short-term trends.
Key Points
Absci's stock faces a landscape of potential, with predictions centering on its AI-driven drug discovery platform's ability to accelerate development timelines and reduce costs, potentially leading to significant partnerships and revenue streams. The company's focus on designing and producing novel protein therapeutics could translate to breakthroughs in various therapeutic areas. However, risks include the inherent uncertainties of drug development, the competitive biotech landscape, and the dependence on successful clinical trials. Regulatory hurdles, potential setbacks in AI model performance, and challenges in scaling manufacturing capabilities also pose considerable downside risks. Investor sentiment and market volatility within the biotech sector will substantially influence the stock's performance.About Absci Corporation
Absci Corporation is a biotechnology company focused on drug discovery and development. Using its proprietary synthetic biology platform, the company aims to discover and develop novel protein-based therapeutics. Their platform combines artificial intelligence, high-throughput screening, and synthetic biology to identify and optimize drug candidates more efficiently. Absci focuses on creating innovative medicines, targeting various diseases and unmet medical needs within the pharmaceutical industry. The company's approach emphasizes the design, engineering, and optimization of proteins for therapeutic applications.
The company's business strategy involves both internal drug development programs and partnerships with other pharmaceutical companies. They collaborate to utilize its platform for drug discovery, as well as research and development endeavors. This strategy allows Absci to expand its reach and diversify its portfolio. By leveraging its technology and collaborative approach, Absci aims to transform drug discovery and accelerate the delivery of novel therapeutics to patients. This effort is crucial in addressing complex diseases through innovative protein-based medicines.
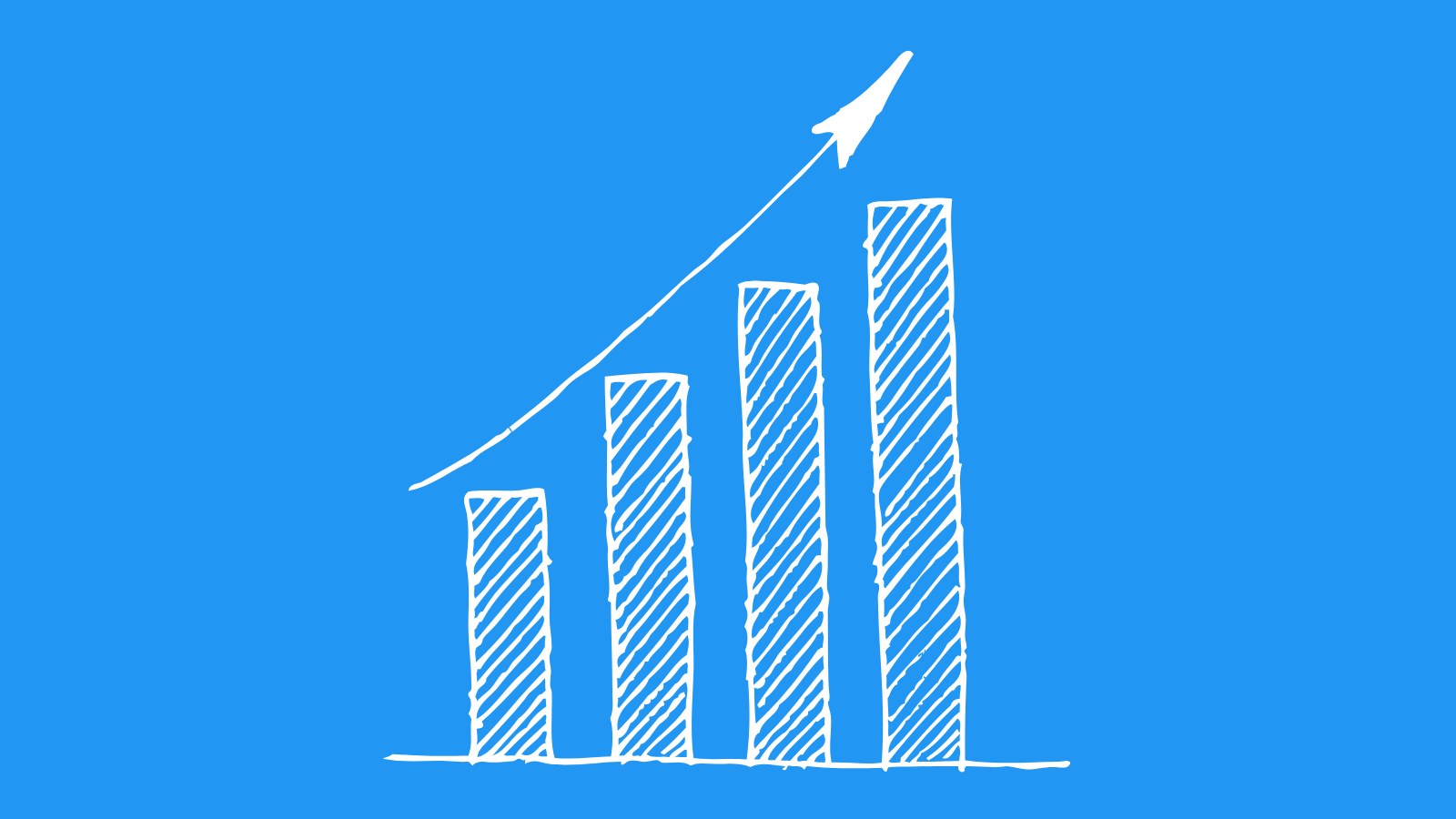
ABSI Stock Forecast Model
As data scientists and economists, we propose a comprehensive machine learning model to forecast Absci Corporation (ABSI) stock performance. Our approach integrates diverse data sources, including historical stock data (volume, open, high, low), financial statements (revenue, earnings per share, debt-to-equity ratio), industry-specific indicators (biotech sector performance, R&D spending trends), and macroeconomic variables (interest rates, inflation). These datasets will be preprocessed through cleaning, handling missing values, and feature engineering. We will employ techniques like moving averages and relative strength index from the technical analysis domain and sentiment analysis of news articles and social media data related to Absci and the broader biotech industry. The model will be trained and validated on historical data, iteratively refining the parameters using appropriate statistical methods.
Our model's core architecture will utilize a hybrid approach, combining the strengths of different machine learning algorithms. We plan to incorporate a Recurrent Neural Network (RNN), particularly Long Short-Term Memory (LSTM) layers, to capture temporal dependencies and sequential patterns within the financial time series data. Simultaneously, we will employ Gradient Boosting Machines (GBM) like XGBoost or LightGBM to model complex non-linear relationships between features, incorporating the structured financial and macroeconomic data. Furthermore, a Random Forest model will add diversity to the ensemble, and feature importance analysis will provide valuable insights into the key drivers of stock price movements. Model evaluation will be conducted using several metrics, including Mean Absolute Error (MAE), Root Mean Squared Error (RMSE), and Sharpe Ratio.
The model will generate forecasts for ABSI stock performance based on different time horizons. The model's output will include the predicted direction (increase, decrease, or no change) of the stock's movement and will provide a confidence interval associated with the predictions. Regular backtesting and re-training of the model using the latest data will ensure its continued accuracy and relevance, mitigating potential biases and adapting to changing market dynamics. Risk management will be paramount and incorporate techniques to handle volatility forecasting. Ultimately, the model's output will inform investment strategies, identifying potential opportunities and providing risk assessments.
```ML Model Testing
n:Time series to forecast
p:Price signals of Absci Corporation stock
j:Nash equilibria (Neural Network)
k:Dominated move of Absci Corporation stock holders
a:Best response for Absci Corporation target price
For further technical information as per how our model work we invite you to visit the article below:
How do KappaSignal algorithms actually work?
Absci Corporation Stock Forecast (Buy or Sell) Strategic Interaction Table
Strategic Interaction Table Legend:
X axis: *Likelihood% (The higher the percentage value, the more likely the event will occur.)
Y axis: *Potential Impact% (The higher the percentage value, the more likely the price will deviate.)
Z axis (Grey to Black): *Technical Analysis%
Absci Corporation Common Stock: Financial Outlook and Forecast
The financial outlook for Absci (ABSI) appears promising, fueled by its innovative Solu-Pro platform and its partnerships within the pharmaceutical industry. The company is strategically positioned to capitalize on the growing demand for novel protein therapeutics. ABSI's core technology, Solu-Pro, offers a unique ability to design and manufacture complex proteins with enhanced properties, including improved stability, manufacturability, and efficacy. This platform significantly reduces the time and cost associated with drug development. Furthermore, the company's collaborative partnerships with major pharmaceutical players validate the potential of its technology and provide revenue streams that are crucial for financial stability and growth. ABSI's focus on biomanufacturing and its ability to offer integrated solutions from drug discovery to clinical development further strengthens its position in the competitive biotechnology landscape. Additionally, the expansion of their pipeline, which includes proprietary and partnered assets, provides diversification, potentially reducing the risk associated with relying on a single product or partner.
ABSI's financial performance is expected to improve, driven by the advancement of its partnered programs and potential milestone payments. Revenue growth is anticipated to be significant as projects move through clinical trials and towards commercialization. The partnerships, including those with prominent biopharmaceutical companies, provide steady revenue through upfront payments, research funding, and future milestone payments, reducing the reliance on raising capital in the short term. The company's ongoing investment in its platform and infrastructure will drive operational efficiency, which is expected to improve profitability over time. Management's commitment to research and development, specifically in extending the capabilities of Solu-Pro, suggests a commitment to future innovation and sustained growth in the long run. ABSI's strategic focus on both expanding its platform capabilities and building a robust pipeline indicates a plan for sustainable financial success.
The company's business model offers several strategic advantages. ABSI's focus on partnering with established pharmaceutical companies for drug development significantly reduces the inherent risk associated with drug development. By leveraging the resources and expertise of its partners, ABSI is able to de-risk the process, accelerate clinical trials, and potentially expedite the time to market for its drug candidates. This collaborative approach also provides a diversified revenue stream, as milestone payments and royalties from successful product launches will boost the company's revenue. Furthermore, ABSI's ability to design, manufacture and bring to market a variety of protein therapeutics positions it as a comprehensive service provider, which will likely to attract even more partners. ABSI's potential to generate long-term value comes from its integrated solutions, which increases its relevance in the biotechnology industry.
Based on its robust technology platform, partnerships, and pipeline, ABSI's financial outlook is positive, with the potential for substantial growth. The successful advancement of partnered programs and the potential for future partnerships are key drivers. However, investors should acknowledge significant risks: primarily, the inherent uncertainty of drug development, regulatory hurdles, and the possibility of intense competition from other companies developing similar technologies. Delays in clinical trials or regulatory approvals, coupled with the failure of drug candidates, can significantly impact financial projections. Overall, while the long-term potential is evident, investors should carefully monitor clinical trial progress and the evolution of strategic partnerships. The success of ABSI is tied to the complex nature of drug development. Despite these risks, ABSI has a strong business model, that positions them for growth in the coming years.
Rating | Short-Term | Long-Term Senior |
---|---|---|
Outlook | Ba3 | Ba2 |
Income Statement | C | Baa2 |
Balance Sheet | B2 | Baa2 |
Leverage Ratios | Baa2 | B2 |
Cash Flow | Baa2 | Baa2 |
Rates of Return and Profitability | Baa2 | C |
*Financial analysis is the process of evaluating a company's financial performance and position by neural network. It involves reviewing the company's financial statements, including the balance sheet, income statement, and cash flow statement, as well as other financial reports and documents.
How does neural network examine financial reports and understand financial state of the company?
References
- Thompson WR. 1933. On the likelihood that one unknown probability exceeds another in view of the evidence of two samples. Biometrika 25:285–94
- Byron, R. P. O. Ashenfelter (1995), "Predicting the quality of an unborn grange," Economic Record, 71, 40–53.
- M. L. Littman. Friend-or-foe q-learning in general-sum games. In Proceedings of the Eighteenth International Conference on Machine Learning (ICML 2001), Williams College, Williamstown, MA, USA, June 28 - July 1, 2001, pages 322–328, 2001
- Athey S, Bayati M, Doudchenko N, Imbens G, Khosravi K. 2017a. Matrix completion methods for causal panel data models. arXiv:1710.10251 [math.ST]
- Akgiray, V. (1989), "Conditional heteroscedasticity in time series of stock returns: Evidence and forecasts," Journal of Business, 62, 55–80.
- Dudik M, Langford J, Li L. 2011. Doubly robust policy evaluation and learning. In Proceedings of the 28th International Conference on Machine Learning, pp. 1097–104. La Jolla, CA: Int. Mach. Learn. Soc.
- Chernozhukov V, Chetverikov D, Demirer M, Duflo E, Hansen C, et al. 2016a. Double machine learning for treatment and causal parameters. Tech. Rep., Cent. Microdata Methods Pract., Inst. Fiscal Stud., London