AUC Score :
Short-term Tactic1 :
Dominant Strategy :
Time series to forecast n:
ML Model Testing : Modular Neural Network (DNN Layer)
Hypothesis Testing : Stepwise Regression
Surveillance : Major exchange and OTC
1Short-term revised.
2Time series is updated based on short-term trends.
Key Points
The WIG20 index is anticipated to experience moderate volatility. A potential for a mild upward trend exists, driven by positive sentiment in global markets and potential inflows of foreign capital. However, the index faces considerable risks. Inflationary pressures, both domestically and globally, could negatively impact corporate earnings and consumer spending, thus leading to a downturn. Furthermore, geopolitical uncertainties and the impact of evolving economic policies pose significant challenges. Investor sentiment, which is always susceptible to rapid shifts, adds another layer of risk. Should any of these risks materialize, they are likely to moderate any gains and may even lead to a correction.About WIG20 Index
The WIG20 is the benchmark stock market index of the Warsaw Stock Exchange (GPW) in Poland. It represents the performance of the 20 largest and most liquid companies listed on the GPW, providing a crucial gauge of the overall health and direction of the Polish equity market. The index is market capitalization-weighted, meaning that companies with larger market values have a greater influence on its value. This reflects the relative significance of these companies within the broader Polish economy. The WIG20 serves as a fundamental tool for investors, analysts, and economists to assess market sentiment and make informed investment decisions.
Regularly reviewed and reconstituted, the composition of the WIG20 ensures it remains representative of the most significant Polish companies. The index is used as a basis for various financial products, including exchange-traded funds (ETFs) and futures contracts. This allows investors to gain exposure to the Polish market or hedge their investment risk. Fluctuations in the WIG20 reflect a variety of factors, including economic performance, corporate earnings, investor sentiment, and global market trends. It is an essential indicator for anyone interested in tracking the Polish stock market's performance.
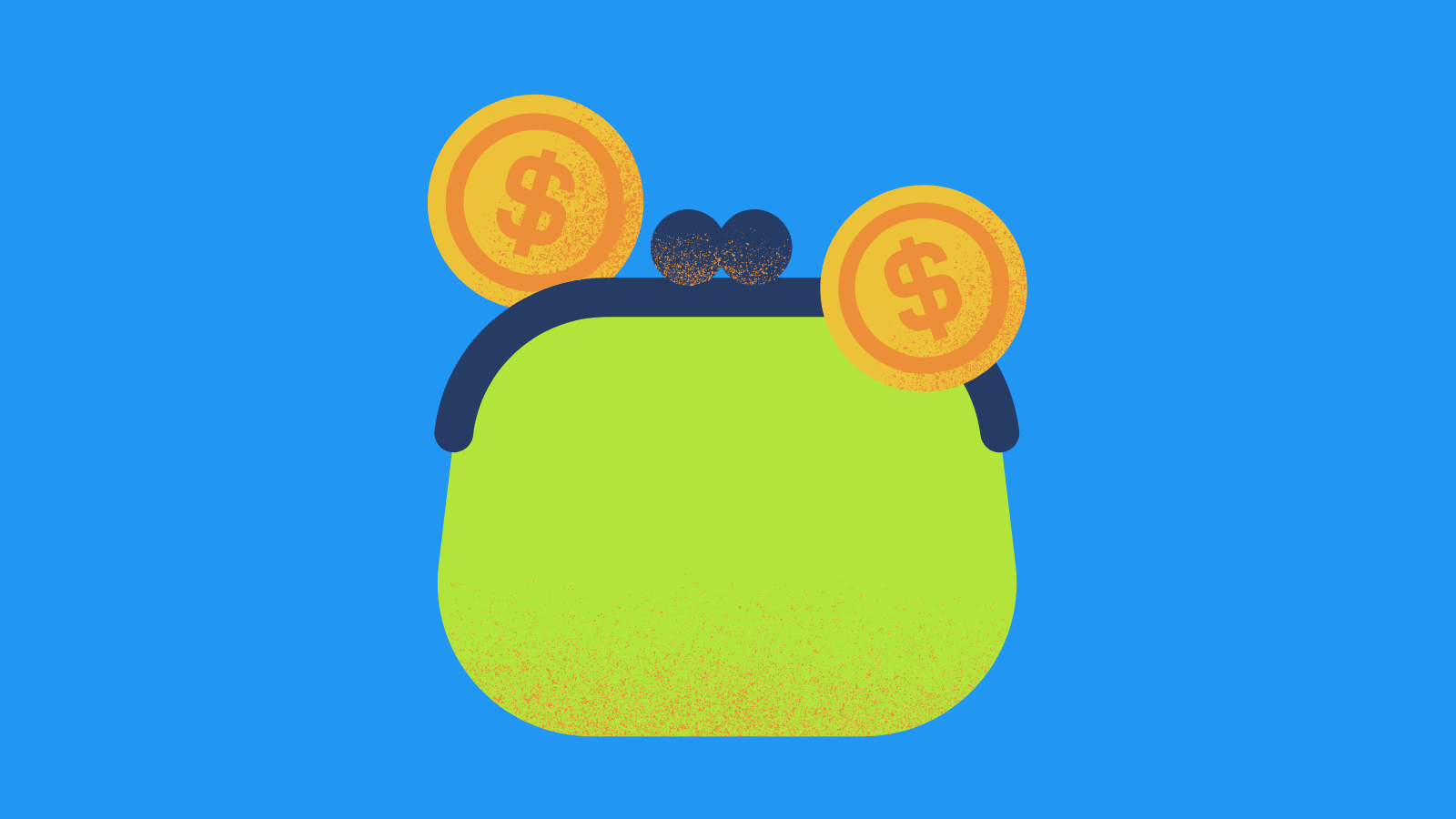
WIG20 Index Forecasting Model
Our team of data scientists and economists has developed a sophisticated machine learning model to forecast the WIG20 index. The model leverages a comprehensive set of input variables, encompassing both technical and fundamental indicators. Technical indicators include moving averages, Relative Strength Index (RSI), and Moving Average Convergence Divergence (MACD) to capture market sentiment and trends. Fundamental factors considered are macroeconomic data such as interest rates, inflation, GDP growth of Poland and the European Union, and investor sentiment derived from surveys and news sentiment analysis. Furthermore, the model incorporates volatility measures, currency exchange rates (particularly the PLN/EUR and PLN/USD), and commodity prices that can influence the Polish economy and subsequently impact the WIG20. The data undergoes rigorous preprocessing including data cleaning, handling missing values, and standardization to ensure data quality and optimal model performance.
The core of our forecasting approach relies on a hybrid model employing various machine learning algorithms. Initially, time series models such as ARIMA and its variants are utilized to capture the temporal dependencies within the WIG20 index. Subsequently, we integrate ensemble methods like Random Forest and Gradient Boosting Machines to capture non-linear relationships and interactions between the diverse input variables. A recurrent neural network (RNN) model is used to capture long-range dependencies in the time series data. These models are optimized using hyperparameter tuning techniques, such as cross-validation, to enhance predictive accuracy. The final forecasts are derived from a weighted ensemble of the outputs from each model, allowing the model to capture advantages of each technique and adjust to changing market dynamics. The model performance is evaluated using metrics such as Mean Absolute Error (MAE), Root Mean Squared Error (RMSE), and R-squared to ensure reliability.
The model's output comprises a daily forecast of the WIG20 index, with the capability to generate forecasts for multiple time horizons. Risk management is integrated into the modeling process, considering factors such as forecast uncertainty. The model is continuously monitored and updated as new data becomes available to maintain its accuracy and relevance. Regular backtesting and retraining are conducted to adapt the model to changing market conditions. This iterative process is designed to produce a robust and informative tool that can support investment decisions and help the Polish economy and related market. The model is designed for use in applications such as portfolio optimization, risk management, and market analysis, providing timely insights to investors and other market participants.
ML Model Testing
n:Time series to forecast
p:Price signals of WIG20 index
j:Nash equilibria (Neural Network)
k:Dominated move of WIG20 index holders
a:Best response for WIG20 target price
For further technical information as per how our model work we invite you to visit the article below:
How do KappaSignal algorithms actually work?
WIG20 Index Forecast Strategic Interaction Table
Strategic Interaction Table Legend:
X axis: *Likelihood% (The higher the percentage value, the more likely the event will occur.)
Y axis: *Potential Impact% (The higher the percentage value, the more likely the price will deviate.)
Z axis (Grey to Black): *Technical Analysis%
WIG20 Index: Financial Outlook and Forecast
The WIG20 index, representing the top 20 companies listed on the Warsaw Stock Exchange (WSE), exhibits a financial outlook that is closely tied to the economic health of Poland and the broader European Union. Poland's economic growth trajectory, influenced by factors such as domestic consumption, investment, and international trade, significantly shapes the performance of WIG20 constituents. The index's outlook is further influenced by global economic trends, geopolitical events, and monetary policy decisions by the National Bank of Poland (NBP) and the European Central Bank (ECB). Sectors like banking, energy, and consumer goods, which are heavily represented in the WIG20, often experience fluctuations based on interest rate adjustments, commodity price volatility, and shifts in consumer confidence. Furthermore, the index is sensitive to investor sentiment, which can be affected by company-specific news, earnings reports, and overall market trends. The WIG20's performance often serves as a barometer for the sentiment of international investors towards the Polish economy.
Several key factors are poised to influence the WIG20's performance in the upcoming period. These include the pace of inflation and subsequent interest rate policies, both domestically and within the Eurozone. Rising interest rates can create headwinds for economic growth, impacting borrowing costs for businesses and potentially dampening consumer spending, thereby affecting the profitability of companies listed on the index. Conversely, easing interest rates can stimulate economic activity and boost investor confidence, leading to a positive impact. The strength of the Polish Zloty (PLN) against major currencies, particularly the Euro and US Dollar, also plays a crucial role. A weakening Zloty can benefit exporters but may increase import costs, while a strengthening Zloty has the opposite effect. Government policies, including fiscal measures, regulatory changes, and infrastructure investments, further influence the business environment and, consequently, the prospects of WIG20 companies. Finally, the ongoing geopolitical climate, particularly the situation in Ukraine, has a substantial impact on the Polish economy and therefore on the WIG20's outlook.
A detailed examination of specific sectors within the WIG20 is essential for a comprehensive forecast. The banking sector, a cornerstone of the index, is influenced by net interest margins, credit growth, and the quality of the loan portfolio. Energy companies are vulnerable to fluctuations in commodity prices, regulatory developments related to renewable energy, and the evolving energy transition landscape. Consumer goods firms rely on consumer purchasing power, which is influenced by employment rates, inflation, and disposable income. Companies involved in real estate and construction are affected by interest rate movements, government infrastructure spending, and overall market demand. Telecommunications, which has a significant weighting in the index, is influenced by competition, technological advancements, and the regulatory environment. The performance of these sectors will be central to the index's overall trajectory, requiring careful monitoring of company-specific strategies and adaptation to the changing economic landscape.
The outlook for the WIG20 index is cautiously optimistic, predicated on a stable economic environment within Poland and Europe. However, this prediction is subject to several risks. An escalation of the conflict in Ukraine or unforeseen geopolitical tensions could disrupt supply chains, increase energy prices, and undermine investor confidence. A steeper-than-expected increase in interest rates by the NBP or ECB could stifle economic growth, leading to lower corporate earnings. Furthermore, a significant slowdown in global economic growth, particularly in the Eurozone, could negatively affect export-oriented Polish companies. Increased inflation eroding consumer spending, and regulatory uncertainties affecting specific sectors such as banking or energy, pose additional challenges. While the potential for economic expansion exists, the prudent investor must acknowledge these risks and actively manage their portfolio to mitigate potential losses.
Rating | Short-Term | Long-Term Senior |
---|---|---|
Outlook | Baa2 | Ba3 |
Income Statement | Baa2 | B3 |
Balance Sheet | Ba1 | Baa2 |
Leverage Ratios | B1 | Baa2 |
Cash Flow | B1 | B2 |
Rates of Return and Profitability | Baa2 | Ba3 |
*An aggregate rating for an index summarizes the overall sentiment towards the companies it includes. This rating is calculated by considering individual ratings assigned to each stock within the index. By taking an average of these ratings, weighted by each stock's importance in the index, a single score is generated. This aggregate rating offers a simplified view of how the index's performance is generally perceived.
How does neural network examine financial reports and understand financial state of the company?
References
- uyer, S. Whiteson, B. Bakker, and N. A. Vlassis. Multiagent reinforcement learning for urban traffic control using coordination graphs. In Machine Learning and Knowledge Discovery in Databases, European Conference, ECML/PKDD 2008, Antwerp, Belgium, September 15-19, 2008, Proceedings, Part I, pages 656–671, 2008.
- Dudik M, Erhan D, Langford J, Li L. 2014. Doubly robust policy evaluation and optimization. Stat. Sci. 29:485–511
- Arjovsky M, Bottou L. 2017. Towards principled methods for training generative adversarial networks. arXiv:1701.04862 [stat.ML]
- J. Peters, S. Vijayakumar, and S. Schaal. Natural actor-critic. In Proceedings of the Sixteenth European Conference on Machine Learning, pages 280–291, 2005.
- Matzkin RL. 1994. Restrictions of economic theory in nonparametric methods. In Handbook of Econometrics, Vol. 4, ed. R Engle, D McFadden, pp. 2523–58. Amsterdam: Elsevier
- L. Panait and S. Luke. Cooperative multi-agent learning: The state of the art. Autonomous Agents and Multi-Agent Systems, 11(3):387–434, 2005.
- Kallus N. 2017. Balanced policy evaluation and learning. arXiv:1705.07384 [stat.ML]