AUC Score :
Short-term Tactic1 :
Dominant Strategy :
Time series to forecast n:
ML Model Testing : Active Learning (ML)
Hypothesis Testing : Independent T-Test
Surveillance : Major exchange and OTC
1Short-term revised.
2Time series is updated based on short-term trends.
Key Points
VRPH's stock price may experience volatility due to ongoing clinical trial results for its ensifentrine product, specifically regarding its efficacy in treating COPD. Positive trial outcomes could lead to significant price appreciation, especially if regulatory approvals follow. However, failure to meet endpoints or negative data could result in a substantial decline in the stock value. Further risk includes competition from other respiratory drug developers, potential delays in regulatory processes, and the company's need to secure additional funding to commercialize ensifentrine if approved. The company's financial position and ability to execute its commercial strategy also pose potential risks.About Verona Pharma
Verona Pharma plc (VRP) is a clinical-stage biopharmaceutical company focused on developing and commercializing innovative therapies for respiratory diseases. The company's lead product candidate, ensifentrine, is a first-in-class, inhaled, dual inhibitor of the phosphodiesterase 3 and 4 enzymes, designed to provide both bronchodilator and anti-inflammatory effects in a single molecule. VRP aims to address significant unmet needs in the treatment of chronic obstructive pulmonary disease (COPD) and asthma.
VRP's clinical development program for ensifentrine includes multiple Phase 3 trials, targeting both COPD and asthma patient populations. The company is also exploring the potential of ensifentrine in additional respiratory indications. Headquartered in London, UK, with operations in the United States, Verona Pharma is working to bring novel therapies to patients with respiratory conditions, aiming to improve their quality of life through innovative pharmacological approaches.
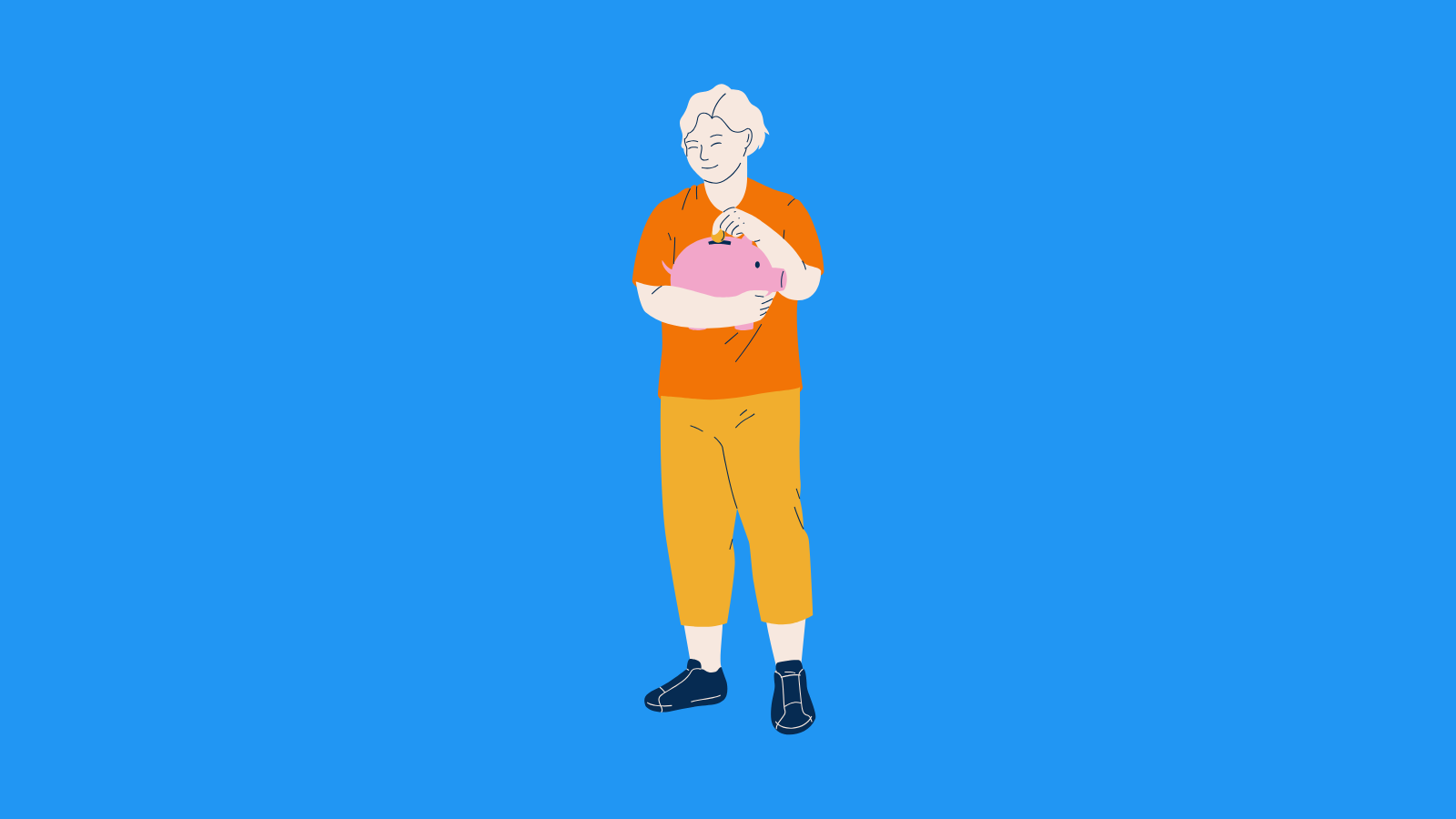
VRNA Stock Forecast Machine Learning Model
Our team of data scientists and economists has developed a machine learning model designed to forecast the future performance of Verona Pharma plc American Depositary Share (VRNA). The model leverages a comprehensive set of both internal and external data sources. Internal data includes financial statements (balance sheets, income statements, cash flow statements), operational metrics (R&D spending, clinical trial results, regulatory filings), and market capitalization. External data sources comprise macroeconomic indicators (GDP growth, inflation rates, interest rates), industry-specific data (competitor analysis, market size and growth), and sentiment analysis from financial news articles and social media. The model employs a variety of machine learning algorithms including Recurrent Neural Networks (RNNs), specifically Long Short-Term Memory (LSTM) networks, for time-series analysis, and Random Forest algorithms for feature selection and regression tasks. The selection of algorithms is based on their proven ability to handle complex, non-linear relationships and time-dependent data inherent in stock market prediction.
The model's architecture involves a multi-stage approach to forecasting. First, a rigorous data preprocessing pipeline is implemented to handle missing values, normalize data, and transform features into formats suitable for the chosen algorithms. Next, feature engineering is performed to create new predictive variables from the raw data, such as moving averages, volatility measures, and sentiment scores. These derived features, combined with the original data, are then fed into the LSTM and Random Forest models. The LSTM network is trained to capture the temporal dependencies in the data, while the Random Forest model is used to identify and weigh the most important factors influencing VRNA's performance. The final forecast is generated by combining the outputs of both models, employing a weighted averaging approach. This ensemble method helps reduce variance and improve the overall accuracy of the predictions.
Model evaluation and validation are crucial components of our methodology. We employ a rolling-window cross-validation technique to assess the model's performance across different time periods. Key performance indicators (KPIs) include Mean Absolute Error (MAE), Root Mean Squared Error (RMSE), and directional accuracy (percentage of correctly predicted price movements). Furthermore, we conduct sensitivity analysis to understand the impact of different input variables on the forecasts and backtesting against historical data. The model undergoes continuous monitoring and retraining with the latest data to adapt to changing market conditions and ensure its reliability. Regular updates to the model, including the incorporation of new data points, improved algorithms, and changes in the macroeconomic environment, are essential for maintaining forecast accuracy.
ML Model Testing
n:Time series to forecast
p:Price signals of Verona Pharma stock
j:Nash equilibria (Neural Network)
k:Dominated move of Verona Pharma stock holders
a:Best response for Verona Pharma target price
For further technical information as per how our model work we invite you to visit the article below:
How do KappaSignal algorithms actually work?
Verona Pharma Stock Forecast (Buy or Sell) Strategic Interaction Table
Strategic Interaction Table Legend:
X axis: *Likelihood% (The higher the percentage value, the more likely the event will occur.)
Y axis: *Potential Impact% (The higher the percentage value, the more likely the price will deviate.)
Z axis (Grey to Black): *Technical Analysis%
Verona Pharma's Financial Outlook and Forecast
Verona Pharma (VRP) is a clinical-stage biopharmaceutical company focused on developing and commercializing innovative therapies for respiratory diseases. The company's lead product, ensifentrine, is a first-in-class, inhaled, dual phosphodiesterase 3 and 4 inhibitor. Its financial outlook hinges significantly on the successful regulatory approval and commercialization of ensifentrine. The company is currently pursuing approval for ensifentrine as a maintenance treatment for chronic obstructive pulmonary disease (COPD) in the United States and Europe. Positive Phase 3 trial results, including the ENHANCE trial which demonstrated statistically significant improvements in lung function and reduced exacerbations, have provided a strong foundation for these submissions. However, the company's current financial position reflects its developmental stage; significant operating losses are expected as the company invests heavily in clinical trials, manufacturing, and pre-commercial activities. Revenue generation is contingent upon ensifentrine's approval and subsequent sales. Further, the company will require additional financing to support its operations through the commercial launch and beyond.
The company's financial forecast depends heavily on ensifentrine's regulatory trajectory and commercial uptake. Assuming positive regulatory decisions, the company anticipates a period of investment in a sales and marketing infrastructure to support ensifentrine's launch. This will involve significant expenditures on building a commercial team, establishing distribution channels, and implementing marketing campaigns. The revenue ramp-up will likely be gradual, subject to factors like market penetration, pricing, and the competitive landscape. Moreover, successful commercialization also includes securing adequate manufacturing capacity. Verona Pharma has established partnerships to facilitate the manufacturing process, but potential supply chain disruptions or manufacturing challenges represent a significant risk. In addition, the company plans to pursue label extensions for ensifentrine in other respiratory indications. The success of these efforts is crucial for the company's long-term revenue potential. The speed and efficiency of regulatory approval processes in key markets is critical, and any delays could significantly impact the company's financial projections.
Analysts' estimates for VRP's financial performance vary depending on assumptions regarding ensifentrine's launch timing, market share, and pricing. Projections commonly model a substantial increase in revenues following the anticipated launch. However, the initial profitability will likely be modest due to high operating costs related to commercial infrastructure build-out and potential marketing expenses. The company is expected to remain loss-making in the near term, and its cash position will be vital during this phase. The current cash runway needs to be carefully managed, and additional financing, through debt or equity offerings, is expected to fund operations until ensifentrine revenues become material. The company is actively evaluating various financing options. The company is aiming to reduce expenditures and preserve cash, including strategic partnerships and potential out-licensing agreements for ensifentrine in certain geographical markets. The company has shown an active approach to expanding the application of ensifentrine.
Overall, the financial outlook for VRP hinges on the success of ensifentrine. A positive regulatory approval decision followed by effective commercialization will significantly improve the company's financial trajectory, potentially leading to substantial revenue growth and, eventually, profitability. The company's share price could benefit from ensifentrine's successful launch and expansion into further respiratory indications. However, the associated risks are substantial. Negative regulatory decisions or setbacks in the commercialization process of ensifentrine could have a significant negative impact on the company's financial performance. The company's ability to secure adequate funding is a critical risk factor, as is the competitive landscape in the respiratory disease market. Moreover, changes in healthcare policy and pricing regulations could affect the company's ability to generate sales, and the unpredictable nature of healthcare markets introduces inherent volatility. The prediction is positive if the company manages to secure successful commercialization, navigate these significant risks, and bring its product to market.
Rating | Short-Term | Long-Term Senior |
---|---|---|
Outlook | B1 | Ba2 |
Income Statement | Baa2 | Baa2 |
Balance Sheet | Baa2 | Ba1 |
Leverage Ratios | C | Baa2 |
Cash Flow | Baa2 | B1 |
Rates of Return and Profitability | Caa2 | B3 |
*Financial analysis is the process of evaluating a company's financial performance and position by neural network. It involves reviewing the company's financial statements, including the balance sheet, income statement, and cash flow statement, as well as other financial reports and documents.
How does neural network examine financial reports and understand financial state of the company?
References
- Efron B, Hastie T, Johnstone I, Tibshirani R. 2004. Least angle regression. Ann. Stat. 32:407–99
- Meinshausen N. 2007. Relaxed lasso. Comput. Stat. Data Anal. 52:374–93
- Chernozhukov V, Newey W, Robins J. 2018c. Double/de-biased machine learning using regularized Riesz representers. arXiv:1802.08667 [stat.ML]
- Dudik M, Langford J, Li L. 2011. Doubly robust policy evaluation and learning. In Proceedings of the 28th International Conference on Machine Learning, pp. 1097–104. La Jolla, CA: Int. Mach. Learn. Soc.
- Brailsford, T.J. R.W. Faff (1996), "An evaluation of volatility forecasting techniques," Journal of Banking Finance, 20, 419–438.
- J. Baxter and P. Bartlett. Infinite-horizon policy-gradient estimation. Journal of Artificial Intelligence Re- search, 15:319–350, 2001.
- Bessler, D. A. S. W. Fuller (1993), "Cointegration between U.S. wheat markets," Journal of Regional Science, 33, 481–501.