AUC Score :
Short-term Tactic1 :
Dominant Strategy :
Time series to forecast n:
ML Model Testing : Modular Neural Network (Speculative Sentiment Analysis)
Hypothesis Testing : Stepwise Regression
Surveillance : Major exchange and OTC
1Short-term revised.
2Time series is updated based on short-term trends.
Key Points
The TR/CC CRB Copper index is expected to experience moderate volatility. Price increases are anticipated due to growing demand from electric vehicle and infrastructure projects coupled with potential supply constraints, however, these gains could be partially offset by economic slowdowns and potential easing of supply chain disruptions. The primary risk lies in a global economic downturn that could significantly curtail demand, leading to a sharp price correction. Additionally, unexpected increases in supply or technological advancements reducing copper's importance could negatively impact the index. Conversely, intensified geopolitical tensions, unforeseen supply chain disruptions, or accelerated green energy initiatives could create significant upward price pressure.About TR/CC CRB Copper Index
The Thomson Reuters/CoreCommodity CRB (TR/CC CRB) Index is a benchmark reflecting the overall price movements of a diverse basket of commodities. It serves as a broad indicator of commodity market performance, encompassing raw materials essential for the global economy. The index includes a variety of commodities, such as energy products, industrial metals, precious metals, agricultural products, and livestock, providing a comprehensive view of the commodity sector. Changes in the TR/CC CRB Index are often used by investors and analysts to gauge inflation expectations, assess global economic health, and make informed investment decisions in commodity-related markets.
The TR/CC CRB Index utilizes a weighted methodology, where the specific commodities and their respective weights within the index are periodically reviewed and adjusted. This process aims to maintain a representative reflection of the global commodity markets. The index's composition and weighting strategy can evolve over time to account for shifts in commodity production, demand, and market dynamics. Consequently, the TR/CC CRB Index offers valuable insights into the collective performance of a significant segment of the commodities market, influencing global financial strategies and economic analyses.
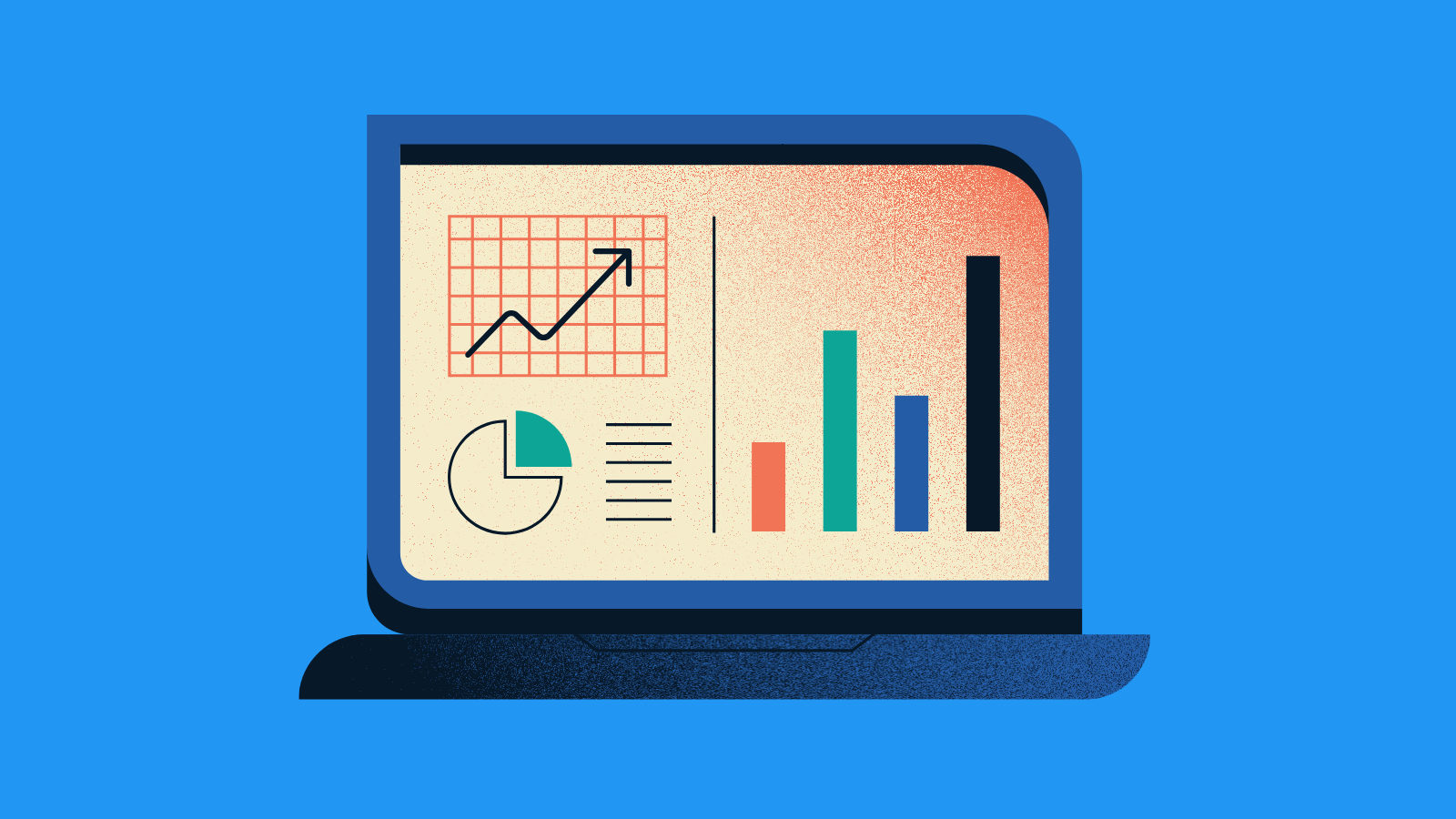
TR/CC CRB Copper Index Forecasting Model
Our team proposes a comprehensive machine learning model for forecasting the TR/CC CRB Copper index, leveraging both time-series data and macroeconomic indicators. The core of our approach will be a hybrid architecture, combining the strengths of Recurrent Neural Networks (RNNs), specifically Long Short-Term Memory (LSTM) networks, with traditional econometric techniques. LSTM networks are particularly well-suited to capture the temporal dependencies and complex patterns inherent in financial time series. We intend to incorporate lagged values of the index itself, along with a range of relevant features. These features include global economic growth indicators (e.g., GDP growth rates from major economies like China, the US, and the EU), industrial production indices, and purchasing managers' indices (PMIs) as well as metal-specific indicators, such as copper inventories, production levels and mining capacity utilization rates. Furthermore, we plan to incorporate currency exchange rates, especially the USD/CNY rate, given China's significant role in the global copper market. The model will be trained on a substantial historical dataset, covering at least the past 10 years, to ensure robust performance and generalization capabilities. The model's output will be a point forecast for a defined period, and we will conduct a series of robustness checks that will consider various time horizons ranging from one week to one quarter.
To address the potential non-stationarity of the time-series data, we will apply appropriate pre-processing techniques. These include techniques such as differencing and detrending to remove any systematic trends or seasonal components and ensure that the data meets the stationarity requirements of our models. Feature scaling (e.g., standardization or min-max scaling) will be applied to bring all features to a comparable scale and improve model convergence. The model training process will involve careful hyperparameter tuning using techniques like grid search or Bayesian optimization to optimize the model's performance. To avoid overfitting, we will employ regularization techniques such as dropout and L1 or L2 regularization. The model's performance will be evaluated using standard metrics such as Mean Absolute Error (MAE), Root Mean Squared Error (RMSE), and Mean Absolute Percentage Error (MAPE). We will also compare the performance of the LSTM-based model to simpler benchmark models such as ARIMA (Autoregressive Integrated Moving Average) and Exponential Smoothing to assess its added value and the ability to capture non-linear relationships. The model will be rigorously validated using out-of-sample data to assess its predictive accuracy and robustness.
Finally, our team will focus on interpretability and risk management. While LSTM networks are often considered "black boxes," we will employ techniques like SHAP (Shapley Additive Explanations) values to provide insights into the feature importance and how the model makes its predictions. This will help to build confidence in the model and allow us to identify the key drivers of copper price movements. Furthermore, we will integrate a risk management framework into the model. This will involve stress-testing the model's predictions under various economic scenarios (e.g., global recessions, supply chain disruptions, and changes in Chinese demand) to assess its sensitivity and identify potential downside risks. We plan to incorporate scenario analysis and sensitivity testing to evaluate the model's response to extreme events and incorporate appropriate risk mitigation strategies. The model will be continuously monitored, and its performance will be regularly reviewed and updated to account for changes in market conditions and the availability of new data.
ML Model Testing
n:Time series to forecast
p:Price signals of TR/CC CRB Copper index
j:Nash equilibria (Neural Network)
k:Dominated move of TR/CC CRB Copper index holders
a:Best response for TR/CC CRB Copper target price
For further technical information as per how our model work we invite you to visit the article below:
How do KappaSignal algorithms actually work?
TR/CC CRB Copper Index Forecast Strategic Interaction Table
Strategic Interaction Table Legend:
X axis: *Likelihood% (The higher the percentage value, the more likely the event will occur.)
Y axis: *Potential Impact% (The higher the percentage value, the more likely the price will deviate.)
Z axis (Grey to Black): *Technical Analysis%
TR/CC CRB Copper Index: Financial Outlook and Forecast
The TR/CC CRB Copper Index, reflecting the price movements of copper within a broader commodity basket, is subject to a complex interplay of global economic factors, supply-demand dynamics, and geopolitical events. Analysis of the current environment points towards a period of moderate growth, with a cautious outlook. The copper market is influenced by industrial activity, particularly in the manufacturing and construction sectors. Strong performance in these areas, driven by infrastructure projects and technological advancements, often fuels increased demand for copper. Simultaneously, the index is impacted by supply-side constraints, including mine production, potential disruptions, and the availability of refined copper. Furthermore, currency fluctuations, interest rates, and investor sentiment significantly influence price movements, impacting the overall investment climate for copper futures. The index, therefore, requires careful monitoring and forecasting, taking these macroeconomic forces into consideration.
Demand for copper is anticipated to be bolstered by several key trends. The global shift towards renewable energy and electric vehicles is projected to increase copper consumption, as the metal is a critical component in these industries. Emerging markets, especially those undergoing urbanization and infrastructure development, are expected to remain significant drivers of copper demand. However, the demand-side outlook is also exposed to potential headwinds, such as economic slowdowns in major economies like China and the United States. The construction sector, a major copper consumer, may experience cyclical downturns, which could moderate demand growth. Supply-side considerations are similarly complex. Mine production can be impacted by various factors, including geological challenges, environmental regulations, and labor disputes. Refined copper availability may be influenced by refining capacity and logistical constraints. These elements contribute to supply-side volatility and are crucial to consider when evaluating the index's performance.
Technological advancements and sustainability initiatives are also impacting the landscape. The integration of smart grids and advanced electrical infrastructure is expected to increase copper usage. Recycling and circular economy models, aimed at improving copper reclamation, could potentially impact the supply dynamics and influence the price of the index. Moreover, geopolitical tensions and trade policies play a significant role, as they can affect supply chain efficiency and impact international trade flows of copper. Shifts in these policies can disrupt the stability of the copper market, potentially impacting prices and volatility. Governments' focus on transitioning towards a low-carbon economy has also heightened the importance of copper.
Considering the above factors, the outlook for the TR/CC CRB Copper Index is moderately positive in the medium term. Demand from renewable energy, electric vehicles, and infrastructure development is expected to provide a degree of support to copper prices. However, the index faces risks related to economic downturns, supply disruptions, and geopolitical uncertainty. The primary risk is a potential slowdown in global economic growth, which could significantly diminish copper demand, and also increased inflation and interest rates. The positive prediction is contingent on stable economic growth, successful infrastructure projects, and advancements in recycling technologies. These factors, if realized, are anticipated to foster a period of steady demand, potentially leading to moderate growth of the index.
Rating | Short-Term | Long-Term Senior |
---|---|---|
Outlook | B1 | Ba1 |
Income Statement | C | C |
Balance Sheet | Baa2 | Baa2 |
Leverage Ratios | B3 | Baa2 |
Cash Flow | Baa2 | Baa2 |
Rates of Return and Profitability | B1 | Ba1 |
*An aggregate rating for an index summarizes the overall sentiment towards the companies it includes. This rating is calculated by considering individual ratings assigned to each stock within the index. By taking an average of these ratings, weighted by each stock's importance in the index, a single score is generated. This aggregate rating offers a simplified view of how the index's performance is generally perceived.
How does neural network examine financial reports and understand financial state of the company?
References
- A. Shapiro, W. Tekaya, J. da Costa, and M. Soares. Risk neutral and risk averse stochastic dual dynamic programming method. European journal of operational research, 224(2):375–391, 2013
- Jorgenson, D.W., Weitzman, M.L., ZXhang, Y.X., Haxo, Y.M. and Mat, Y.X., 2023. Google's Stock Price Set to Soar in the Next 3 Months. AC Investment Research Journal, 220(44).
- Efron B, Hastie T. 2016. Computer Age Statistical Inference, Vol. 5. Cambridge, UK: Cambridge Univ. Press
- Akgiray, V. (1989), "Conditional heteroscedasticity in time series of stock returns: Evidence and forecasts," Journal of Business, 62, 55–80.
- Jorgenson, D.W., Weitzman, M.L., ZXhang, Y.X., Haxo, Y.M. and Mat, Y.X., 2023. Apple's Stock Price: How News Affects Volatility. AC Investment Research Journal, 220(44).
- Breiman L. 1996. Bagging predictors. Mach. Learn. 24:123–40
- A. K. Agogino and K. Tumer. Analyzing and visualizing multiagent rewards in dynamic and stochastic environments. Journal of Autonomous Agents and Multi-Agent Systems, 17(2):320–338, 2008