AUC Score :
Short-term Tactic1 :
Dominant Strategy :
Time series to forecast n:
ML Model Testing : Transfer Learning (ML)
Hypothesis Testing : Stepwise Regression
Surveillance : Major exchange and OTC
1Short-term revised.
2Time series is updated based on short-term trends.
Key Points
Teva's outlook appears cautiously optimistic, contingent upon successful execution of its restructuring plan and pipeline advancements. The company may experience moderate growth stemming from new drug launches and expansion in biosimilars, potentially offsetting generic drug price erosion. The major risk lies in unforeseen litigation outcomes related to opioid settlements and the ongoing competitive pressures within the generics market, which could significantly impact financial performance. Regulatory hurdles and delays in drug approvals, as well as any setbacks in clinical trials, also pose considerable threats to the company's progress. Additional risks include fluctuations in currency exchange rates, which can influence its global operations, and its ability to adapt to shifts in the pharmaceutical industry.About Teva Pharmaceutical Industries
Teva is a multinational pharmaceutical company with a significant global presence. It is primarily focused on the development, manufacturing, and commercialization of both generic and specialty pharmaceutical products. Teva's generic segment offers a broad portfolio of medicines, addressing a wide range of therapeutic areas, providing affordable healthcare solutions. The company's specialty medicines division concentrates on innovative therapies, including treatments for neurological disorders, respiratory conditions, and other specialized areas. These specialty products often command higher prices and contribute to the company's overall revenue.
Beyond its product portfolio, Teva operates through an extensive global network, including manufacturing facilities and distribution channels. The company strategically invests in research and development to expand its product pipeline and maintain a competitive edge. Key to Teva's operational success is its ability to navigate complex regulatory landscapes and manage the lifecycle of its products effectively. It faces typical challenges inherent to the pharmaceutical industry, such as patent expirations, competition, and pricing pressures.
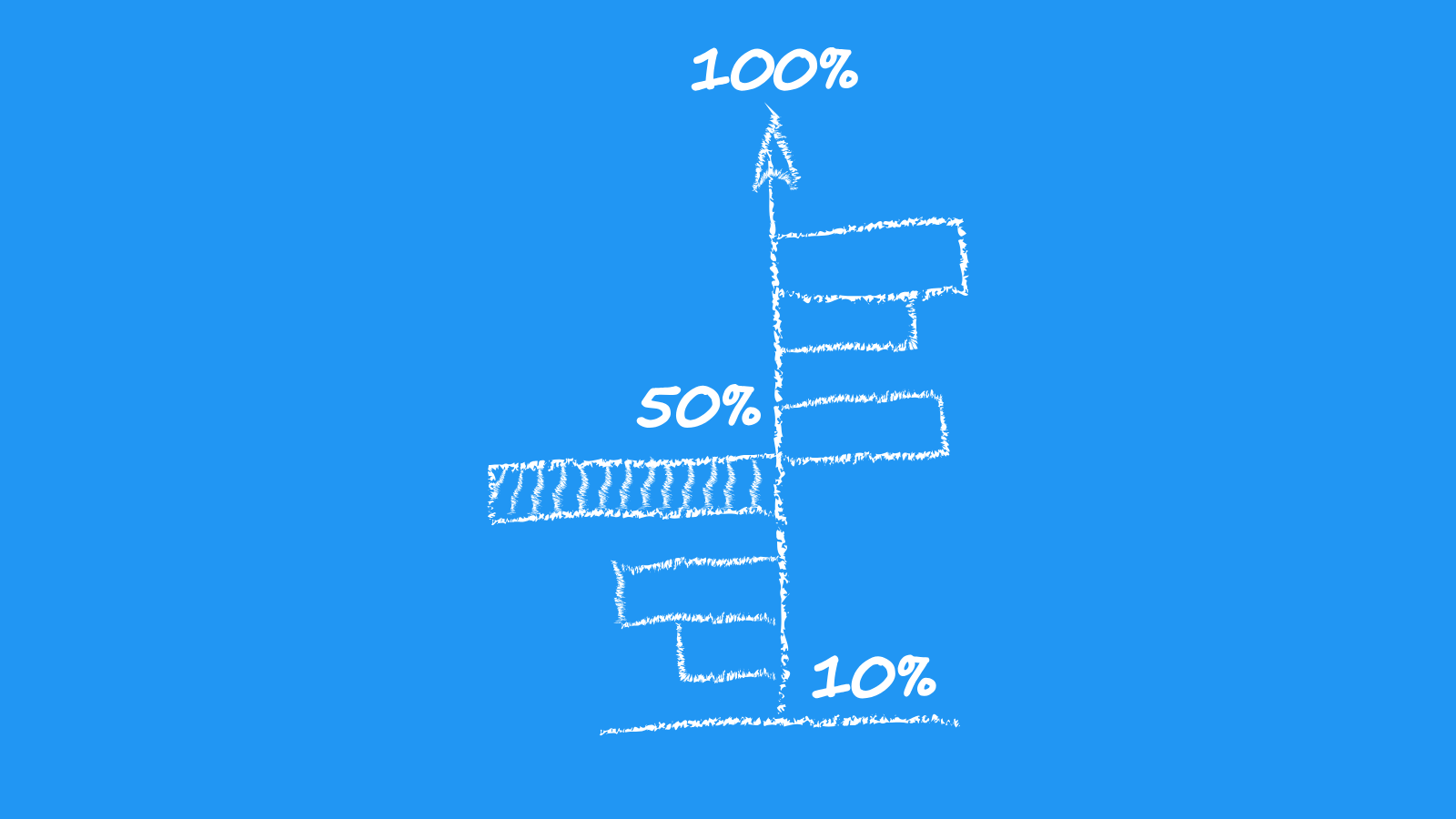
TEVA Stock Prediction Model
Our team proposes a machine learning model to forecast the future performance of Teva Pharmaceutical Industries Limited American Depositary Shares (TEVA). The model's architecture incorporates a combination of time-series analysis and machine learning techniques. We will leverage a comprehensive dataset including historical stock data (daily adjusted closing prices, trading volumes), financial statements (quarterly and annual reports outlining revenue, earnings, debt, and cash flow), macroeconomic indicators (industry-specific growth rates, inflation data, interest rates), and sentiment analysis derived from news articles and social media discussions related to TEVA and the pharmaceutical industry. Feature engineering will be a crucial step, encompassing the creation of technical indicators (moving averages, RSI, MACD), financial ratios (price-to-earnings, debt-to-equity), and sentiment scores. The data will undergo rigorous cleaning, transformation (e.g., normalization), and feature selection to optimize model performance and minimize overfitting.
The core of the model will be a hybrid approach. We intend to employ a combination of Recurrent Neural Networks (RNNs), specifically Long Short-Term Memory (LSTM) networks, and ensemble methods. LSTMs are well-suited for time-series data, capable of capturing long-term dependencies within the TEVA stock's behavior. Ensemble techniques, such as Random Forest or Gradient Boosting, will be used to further improve prediction accuracy and robustness. The model will be trained on historical data and validated using a hold-out dataset to ensure its generalizability. Furthermore, we'll utilize cross-validation techniques to assess the model's stability and reduce the risk of overfitting. Regular model evaluations will be performed to ensure optimal performance over time, re-training the model with new data when necessary.
The model's success will be measured by key metrics, including Mean Squared Error (MSE), Root Mean Squared Error (RMSE), and Mean Absolute Error (MAE). We will also evaluate its directional accuracy (i.e., the percentage of correctly predicted price movements). The model will be designed to generate predictions on a defined time horizon (e.g., daily, weekly, or monthly). The output will be a forecast of the predicted change in the share price, along with associated confidence intervals. This model provides valuable insights into potential market movements, allowing investors and stakeholders to make informed decisions about TEVA. The model's outputs will be constantly monitored, and adjusted if there are any environmental changes within the data.
ML Model Testing
n:Time series to forecast
p:Price signals of Teva Pharmaceutical Industries stock
j:Nash equilibria (Neural Network)
k:Dominated move of Teva Pharmaceutical Industries stock holders
a:Best response for Teva Pharmaceutical Industries target price
For further technical information as per how our model work we invite you to visit the article below:
How do KappaSignal algorithms actually work?
Teva Pharmaceutical Industries Stock Forecast (Buy or Sell) Strategic Interaction Table
Strategic Interaction Table Legend:
X axis: *Likelihood% (The higher the percentage value, the more likely the event will occur.)
Y axis: *Potential Impact% (The higher the percentage value, the more likely the price will deviate.)
Z axis (Grey to Black): *Technical Analysis%
Teva Pharmaceutical's Financial Outlook and Forecast
Teva's financial outlook presents a mixed bag of challenges and opportunities in the pharmaceutical sector. The company, a global leader in generic drugs and a significant player in specialty pharmaceuticals, faces an environment characterized by increased competition, pricing pressures, and ongoing patent expirations, particularly within its generics business. This has led to a revenue decline over the past few years. Furthermore, Teva continues to manage substantial debt levels accumulated through acquisitions. However, the company has been implementing strategic initiatives designed to improve its financial performance. These initiatives include a focus on cost-cutting measures, such as restructuring and workforce reductions, streamlining its operations, and divesting non-core assets to reduce debt.
The company's financial forecast hinges on the successful execution of its strategic plan. A key area of focus is the growth of its biosimilar portfolio, which offers higher margins than traditional generics. Also, Teva is actively pursuing the launch of new branded products and expanding its presence in emerging markets to diversify its revenue streams. Another significant factor influencing its financial performance is the resolution of ongoing litigation, specifically related to opioid-related lawsuits. Favorable outcomes or settlements in these legal battles could positively impact the company's financial health. Teva's commitment to research and development in both generics and specialty products provides a pipeline for future growth. Teva is looking to expand into innovative drug development in areas like migraine treatment and movement disorders to bolster the future.
Teva's financial analysts have offered varied perspectives on the company's financial prospects. Some analysts predict that Teva will show growth in some segments due to cost-cutting and the expansion of its branded drug portfolio. Revenue growth is anticipated to remain subdued, although efforts to control costs and reduce debt are expected to improve profitability. The company's financial health depends on its ability to successfully manage the complexities of its diverse portfolio, regulatory issues, and changing market dynamics. Strong performance in its core generic drug business is crucial, as it still constitutes a significant portion of its overall revenue. The successful integration of recent acquisitions and partnerships will be very important for the near future. The resolution of the opioid lawsuits and the development of new drugs will also greatly influence its financial condition.
Overall, the financial outlook for Teva appears cautiously optimistic. A modest recovery is projected, driven by successful execution of its strategic plan, cost-cutting initiatives, and the potential for growth in its biosimilars and specialty pharmaceuticals businesses. However, the company faces significant risks. The highly competitive nature of the pharmaceutical industry, regulatory hurdles, and the volatile nature of the global economy, create challenges. The failure to reduce its debt burden, adverse outcomes in ongoing litigation, and slower-than-expected growth in its new product pipeline could significantly impede Teva's financial recovery and negatively impact investor confidence. Therefore, the financial performance will remain under pressure in the short term with positive growth potential in the long term.
Rating | Short-Term | Long-Term Senior |
---|---|---|
Outlook | B2 | Ba3 |
Income Statement | B3 | Caa2 |
Balance Sheet | Ba3 | C |
Leverage Ratios | C | Baa2 |
Cash Flow | Baa2 | Baa2 |
Rates of Return and Profitability | C | B1 |
*Financial analysis is the process of evaluating a company's financial performance and position by neural network. It involves reviewing the company's financial statements, including the balance sheet, income statement, and cash flow statement, as well as other financial reports and documents.
How does neural network examine financial reports and understand financial state of the company?
References
- Andrews, D. W. K. (1993), "Tests for parameter instability and structural change with unknown change point," Econometrica, 61, 821–856.
- R. Williams. Simple statistical gradient-following algorithms for connectionist reinforcement learning. Ma- chine learning, 8(3-4):229–256, 1992
- Chernozhukov V, Newey W, Robins J. 2018c. Double/de-biased machine learning using regularized Riesz representers. arXiv:1802.08667 [stat.ML]
- K. Tuyls and G. Weiss. Multiagent learning: Basics, challenges, and prospects. AI Magazine, 33(3): 41–52, 2012
- Bai J, Ng S. 2017. Principal components and regularized estimation of factor models. arXiv:1708.08137 [stat.ME]
- Bottou L. 1998. Online learning and stochastic approximations. In On-Line Learning in Neural Networks, ed. D Saad, pp. 9–42. New York: ACM
- Athey S, Imbens GW. 2017a. The econometrics of randomized experiments. In Handbook of Economic Field Experiments, Vol. 1, ed. E Duflo, A Banerjee, pp. 73–140. Amsterdam: Elsevier