AUC Score :
Short-term Tactic1 :
Dominant Strategy :
Time series to forecast n:
ML Model Testing : Ensemble Learning (ML)
Hypothesis Testing : Independent T-Test
Surveillance : Major exchange and OTC
1Short-term revised.
2Time series is updated based on short-term trends.
Key Points
SLB is anticipated to experience moderate growth, driven by increasing oil and gas exploration and production activities globally. This should be supported by a rising demand for SLB's services and technologies. However, SLB faces risks from volatile energy prices, which can significantly impact client spending and project profitability. Geopolitical instability also poses a threat, potentially disrupting operations and supply chains in key regions. Competition from other major oilfield services companies could squeeze margins and market share. Additionally, the transition to renewable energy presents a long-term challenge, requiring SLB to adapt its business model and invest in alternative technologies. These factors could collectively impact the company's financial performance.About Schlumberger N.V.
SLB is a global technology company that powers the energy industry. Founded in 1926, the company provides technology and solutions to the energy sector, with a focus on reservoir characterization, drilling, production, and processing. SLB operates in over 120 countries, serving a diverse clientele, including national and international oil and gas companies.
The company's operations are organized into four divisions: Digital & Integration, Reservoir Performance, Well Construction, and Production Systems. SLB invests heavily in research and development to create innovative technologies and services that improve efficiency, enhance performance, and reduce environmental impact for its customers. Its offerings are critical to exploration, development, and optimization of oil and gas resources worldwide.
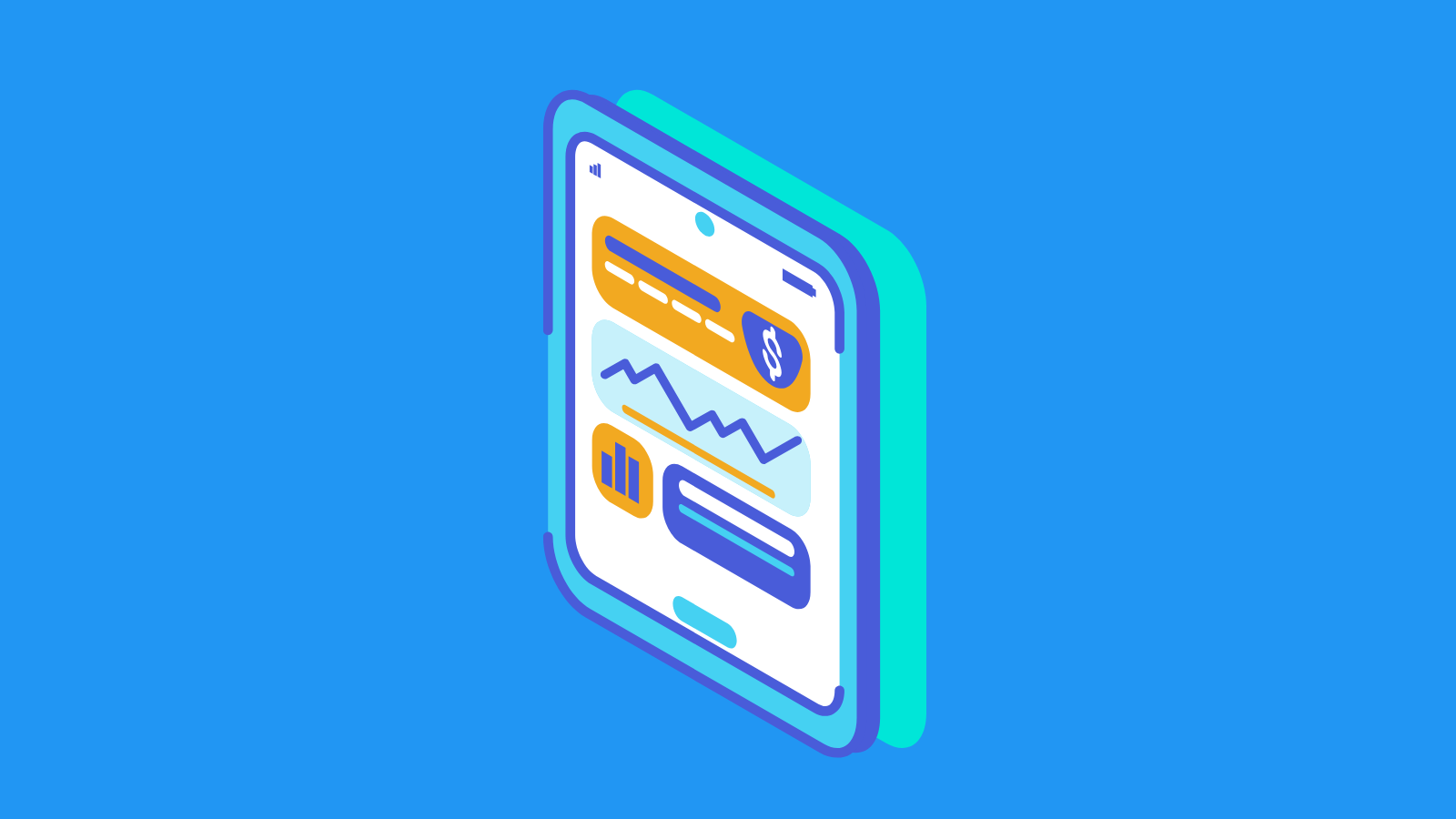
SLB Stock Forecast Model
Our team has developed a comprehensive machine learning model designed to forecast Schlumberger N.V. (SLB) stock performance. The model leverages a multifaceted approach, integrating diverse datasets to capture the intricate factors influencing SLB's market behavior. Key inputs include macroeconomic indicators such as oil prices, global GDP growth, and inflation rates, given their direct correlation with energy sector activity. We also incorporate company-specific data, including quarterly earnings reports (revenue, profit margins, debt levels), operational metrics (e.g., rig counts, project backlogs), and news sentiment analysis gathered from financial news sources and social media platforms. Furthermore, we consider industry-specific trends, encompassing competitor performance, technological advancements, and regulatory changes. The model is designed to be dynamic and adaptable, continuously learning and refining its predictions as new data becomes available.
The core of our model employs a blend of machine learning algorithms. We primarily utilize ensemble methods, such as gradient boosting and random forests, to capitalize on the strength of multiple predictive models. These algorithms are adept at handling the non-linear relationships and complex interactions inherent in financial markets. Furthermore, we incorporate time series analysis techniques, including ARIMA and its variants, to identify and model the temporal dependencies within SLB's historical stock data. The selection of the optimal algorithm and the weighting of different data sources are determined through rigorous validation and cross-validation procedures, ensuring robustness and generalizability. A key feature of the model is the use of feature engineering to construct new features from existing ones to better capture the non-linear behaviour. This can include the creation of ratios (e.g., debt-to-equity, revenue growth), volatility measures, and sentiment scores.
Model output includes probabilistic forecasts of SLB's performance over different time horizons. This encompasses both point estimates and confidence intervals, allowing for risk assessment and informed investment decision-making. The model output will also include insights into the key drivers of the forecast, enabling transparency and facilitating a deeper understanding of the predicted market movements. To manage model uncertainty, we utilize backtesting and stress-testing procedures. Regular monitoring of model performance, including accuracy metrics and bias checks, is performed to ensure that the model adapts to changes in market conditions and data distributions. We will perform the analysis to understand the model bias to maintain the model's reliability over time. Finally, the team is committed to ongoing model improvement, incorporating new data sources, refining algorithms, and enhancing the interpretability of the predictions.
ML Model Testing
n:Time series to forecast
p:Price signals of Schlumberger N.V. stock
j:Nash equilibria (Neural Network)
k:Dominated move of Schlumberger N.V. stock holders
a:Best response for Schlumberger N.V. target price
For further technical information as per how our model work we invite you to visit the article below:
How do KappaSignal algorithms actually work?
Schlumberger N.V. Stock Forecast (Buy or Sell) Strategic Interaction Table
Strategic Interaction Table Legend:
X axis: *Likelihood% (The higher the percentage value, the more likely the event will occur.)
Y axis: *Potential Impact% (The higher the percentage value, the more likely the price will deviate.)
Z axis (Grey to Black): *Technical Analysis%
Schlumberger N.V. Financial Outlook and Forecast
SLB, a global leader in oilfield services, is poised for a period of sustained growth, driven by increasing global energy demand and the ongoing need for oil and gas production. The company's diverse portfolio, encompassing reservoir characterization, drilling and evaluation, and production, positions it favorably to capitalize on opportunities across the entire energy value chain. Furthermore, SLB's strategic focus on digitalization and sustainable solutions, including carbon capture and storage technologies, is expected to enhance its long-term competitiveness and adaptability in a rapidly evolving energy landscape. Continued investment in research and development, aimed at improving operational efficiency and reducing environmental impact, will be crucial for maintaining its leadership position. SLB is also benefiting from a recovering international market as energy companies ramp up their spending in response to higher prices, while also navigating geopolitical uncertainties and economic fluctuations.
The financial outlook for SLB is projected to be robust. Revenue is expected to experience consistent growth, supported by increased activity levels in key geographical regions, particularly in North America and the international markets. Profit margins are anticipated to expand as a result of improved pricing dynamics and the successful integration of acquired businesses, along with implemented cost-saving measures. The company's strong balance sheet, characterized by healthy cash flows and manageable debt levels, provides a solid foundation for supporting strategic investments and returning capital to shareholders. Moreover, SLB's commitment to operational excellence and innovation will support its ability to navigate market cycles and maximize profitability. Strong cash flow generation will also enable SLB to continue investing in new technologies and acquisitions to sustain long-term growth.
SLB's forecast is underpinned by several key factors. The continued demand for hydrocarbons, even as the energy transition gains momentum, will underpin the requirement for its services, especially in complex projects and aging fields. SLB's strategic focus on expanding its presence in emerging markets will provide diversification and opportunities. Additionally, the company is expected to drive growth from its focus on the energy transition by leveraging its expertise to support the development of low-carbon technologies, making it a key player in the industry's shift. The company's ability to manage its operational costs efficiently, while keeping pace with technological advancements, will be critical for its overall success. Furthermore, SLB is positioned to benefit from ongoing improvements in project execution and technology deployments.
Overall, SLB's financial future appears positive. The company's strong market position, diverse service offerings, and focus on innovation will enable it to capitalize on favorable market dynamics. The primary risk to this positive outlook is a potential slowdown in global economic growth or an unforeseen drop in oil prices, both of which could reduce demand for its services. Moreover, the company faces risks from increased competition and possible project delays. Another risk includes any significant changes to government energy policies in key operating regions, particularly those that encourage accelerated transition to renewable energy, that could impact long-term demand.
Rating | Short-Term | Long-Term Senior |
---|---|---|
Outlook | B1 | Ba3 |
Income Statement | Caa2 | Baa2 |
Balance Sheet | B2 | C |
Leverage Ratios | Baa2 | B3 |
Cash Flow | B3 | Baa2 |
Rates of Return and Profitability | Ba3 | Ba3 |
*Financial analysis is the process of evaluating a company's financial performance and position by neural network. It involves reviewing the company's financial statements, including the balance sheet, income statement, and cash flow statement, as well as other financial reports and documents.
How does neural network examine financial reports and understand financial state of the company?
References
- Wooldridge JM. 2010. Econometric Analysis of Cross Section and Panel Data. Cambridge, MA: MIT Press
- L. Busoniu, R. Babuska, and B. D. Schutter. A comprehensive survey of multiagent reinforcement learning. IEEE Transactions of Systems, Man, and Cybernetics Part C: Applications and Reviews, 38(2), 2008.
- Mikolov T, Yih W, Zweig G. 2013c. Linguistic regularities in continuous space word representations. In Pro- ceedings of the 2013 Conference of the North American Chapter of the Association for Computational Linguistics: Human Language Technologies, pp. 746–51. New York: Assoc. Comput. Linguist.
- Matzkin RL. 2007. Nonparametric identification. In Handbook of Econometrics, Vol. 6B, ed. J Heckman, E Learner, pp. 5307–68. Amsterdam: Elsevier
- Brailsford, T.J. R.W. Faff (1996), "An evaluation of volatility forecasting techniques," Journal of Banking Finance, 20, 419–438.
- Dudik M, Langford J, Li L. 2011. Doubly robust policy evaluation and learning. In Proceedings of the 28th International Conference on Machine Learning, pp. 1097–104. La Jolla, CA: Int. Mach. Learn. Soc.
- Hornik K, Stinchcombe M, White H. 1989. Multilayer feedforward networks are universal approximators. Neural Netw. 2:359–66