AUC Score :
Short-term Tactic1 :
Dominant Strategy :
Time series to forecast n:
ML Model Testing : Ensemble Learning (ML)
Hypothesis Testing : Stepwise Regression
Surveillance : Major exchange and OTC
1Short-term revised.
2Time series is updated based on short-term trends.
Key Points
Paylocity's future performance is projected to be positive, driven by continued expansion within the human capital management software market, fueled by increased adoption among small and medium-sized businesses, and a potential for increased revenue through cross-selling of its suite of products. The company is expected to experience revenue growth and profitability gains over the coming years, driven by its recurring revenue model and strong client retention. However, Paylocity faces risks including increased competition from established and emerging HCM providers, potential slowdowns in economic growth impacting customer spending on its services, the need for continued investment in research and development to maintain its technological edge, and the impact of evolving regulatory environments impacting the HR and payroll landscapes. These factors could present challenges to the company's growth trajectory and profitability.About Paylocity Holding Corporation
Paylocity (PCTY) is a leading provider of cloud-based human capital management (HCM) software solutions. Founded in 1997, the company caters primarily to the needs of medium-sized businesses across the United States. Its comprehensive platform offers integrated functionalities, including payroll processing, human resources, benefits administration, talent management, and time and labor management. Paylocity's focus on user experience and innovation has allowed it to build a strong reputation and a growing customer base.
Paylocity emphasizes a comprehensive and adaptable HCM platform, which facilitates employee engagement and streamlined administrative processes. The company's growth strategy centers on acquiring and retaining clients, as well as expanding its suite of HCM solutions. Furthermore, Paylocity continues to invest in research and development to improve its product offerings and address emerging trends within the human capital management space. Its commitment to providing value to its customers positions it favorably in the competitive HCM market.
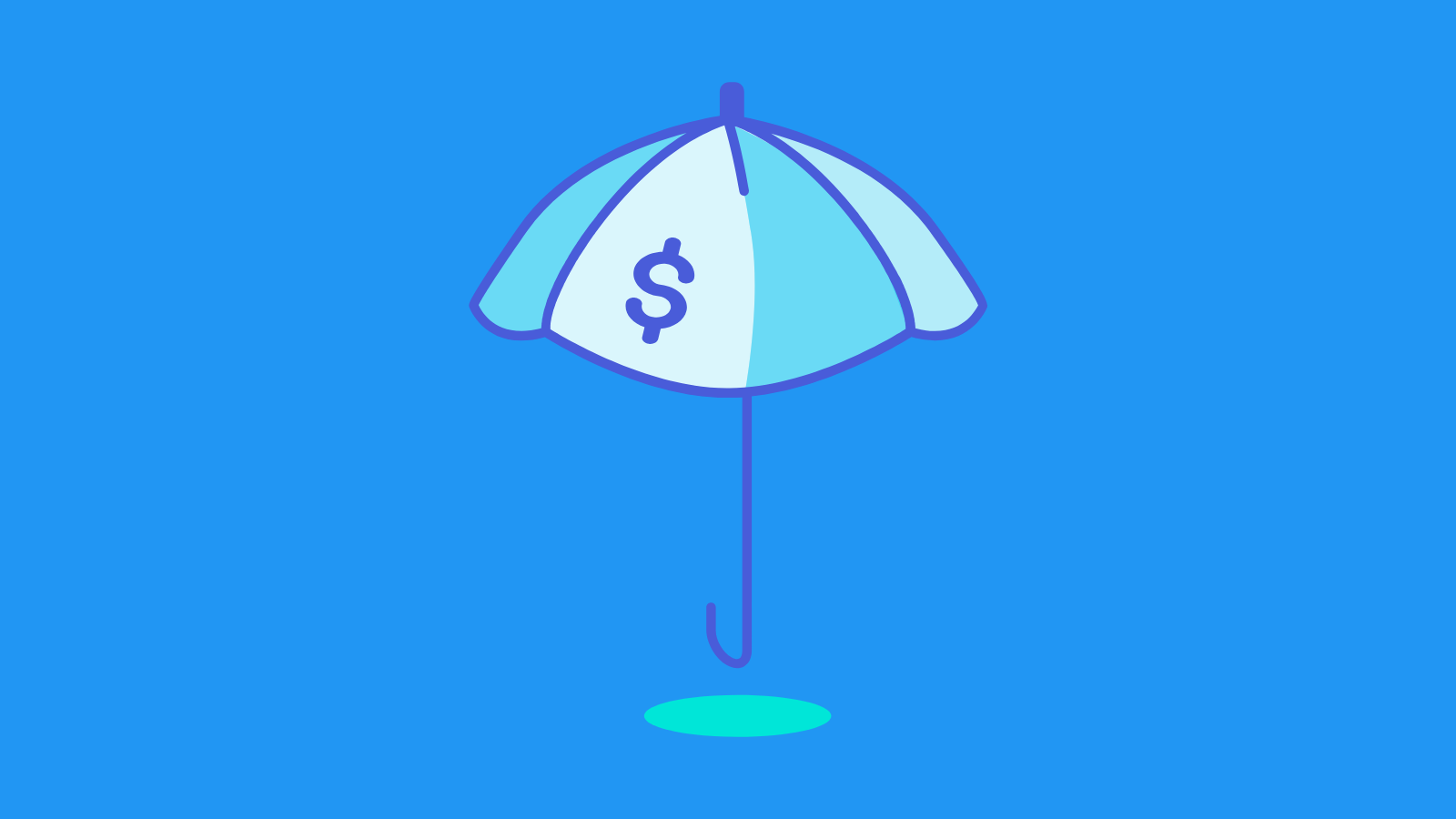
PCTY Stock Price Forecasting Model
Our team, comprised of data scientists and economists, has developed a machine learning model to forecast Paylocity Holding Corporation (PCTY) stock performance. The model leverages a comprehensive dataset encompassing diverse factors known to influence stock behavior. These include, but are not limited to, historical PCTY stock price data, macroeconomic indicators such as inflation rates, interest rates, and GDP growth; industry-specific metrics like competitor performance and market share fluctuations; financial data extracted from Paylocity's earnings reports, including revenue, earnings per share (EPS), and debt levels; and finally, sentiment analysis derived from news articles, social media, and analyst reports related to PCTY and the human capital management (HCM) software industry. The model's construction focuses on data preprocessing, feature engineering, model selection, training, and validation. Data preprocessing includes cleaning, handling missing values, and scaling the features. Feature engineering involves creating new features based on existing ones, such as moving averages and ratios, to enhance the model's predictive power.
The core of our forecasting model employs a hybrid approach, combining multiple machine learning algorithms. We utilize both time-series models, such as ARIMA and its variants, to capture temporal dependencies in the stock price, and ensemble methods, like Random Forests and Gradient Boosting, to incorporate the complex relationships between the diverse input features. This hybrid structure allows us to effectively model the stock price behavior and incorporate both time-dependent and feature-dependent information. Furthermore, we've implemented a recursive feature elimination strategy to identify the most impactful features, preventing model overfitting and optimizing predictive accuracy. Model training involves splitting the dataset into training, validation, and test sets to assess model performance using appropriate metrics like Mean Absolute Error (MAE) and Root Mean Squared Error (RMSE). The model is then fine-tuned using cross-validation techniques to enhance its generalization capabilities.
The model's output provides a forecast of PCTY stock performance, and associated confidence intervals, over a specified future period. This forecast will undergo continuous monitoring and evaluation through backtesting and comparison with actual market movements to ensure accuracy and reliability. The forecasts are intended to be used to aid in making well-informed investment decisions. Regular updates and improvements will be applied to the model as fresh data becomes available and as the understanding of market dynamics deepens. This model is a tool for decision support and should be used in conjunction with other investment analysis techniques and consideration of individual risk tolerance. The forecast is not financial advice and should not be considered as such. Risks of investing should be taken into consideration.
ML Model Testing
n:Time series to forecast
p:Price signals of Paylocity Holding Corporation stock
j:Nash equilibria (Neural Network)
k:Dominated move of Paylocity Holding Corporation stock holders
a:Best response for Paylocity Holding Corporation target price
For further technical information as per how our model work we invite you to visit the article below:
How do KappaSignal algorithms actually work?
Paylocity Holding Corporation Stock Forecast (Buy or Sell) Strategic Interaction Table
Strategic Interaction Table Legend:
X axis: *Likelihood% (The higher the percentage value, the more likely the event will occur.)
Y axis: *Potential Impact% (The higher the percentage value, the more likely the price will deviate.)
Z axis (Grey to Black): *Technical Analysis%
Paylocity Holding Corporation: Financial Outlook and Forecast
The financial outlook for Paylocity (PCTY) remains positive, underpinned by its robust performance in the human capital management (HCM) software market. The company has demonstrated consistent revenue growth, driven by strong sales of its cloud-based platform and an increasing adoption rate among its target clientele, which includes small to medium-sized businesses (SMBs). PCTY's business model, centered around recurring revenue streams from software subscriptions, provides a degree of stability and predictability, enabling the company to forecast future financial performance with reasonable accuracy. Furthermore, Paylocity has a proven track record of successfully integrating acquisitions, expanding its product offerings, and gaining market share, thus strengthening its competitive position. The company's focus on innovation, particularly in areas like artificial intelligence (AI) and employee engagement tools, is likely to further fuel revenue growth by meeting the evolving needs of its customers. Overall, Paylocity's financial health appears solid, reflecting its ability to execute its strategic plan and capture opportunities in the expanding HCM market.
Paylocity's forecasts for future financial performance suggest continued growth. Analysts generally project healthy revenue increases over the next several years, supported by a combination of new client acquisitions and an expanding product suite. Moreover, the company is expected to maintain or improve its operating margins as it achieves economies of scale and optimizes its cost structure. Management's guidance typically aligns with these positive expectations, and its proactive investment in research and development (R&D) indicates a commitment to maintain its competitive edge. The company's strong free cash flow generation also provides it with flexibility to invest in strategic initiatives, return capital to shareholders, or pursue additional acquisitions. Paylocity's expansion into new market segments and its focus on providing a comprehensive suite of HCM solutions will likely act as catalysts for growth. The company also has a demonstrated ability to retain customers, which ensures a steady stream of recurring revenue and supports long-term sustainability.
Several factors contribute to Paylocity's favorable long-term growth prospects. The HCM software market is experiencing rapid expansion, fueled by increasing demand from businesses of all sizes that aim to improve their efficiency in managing human resources and streamline their operations. The company's cloud-based platform provides a flexible and scalable solution for its customers and allows them to access their data from anywhere. Paylocity has an advantage over many competitors by providing a strong focus on employee engagement, which has grown as an important aspect of attracting and retaining talent for many companies. Moreover, Paylocity's success in integrating new products and features into its platform, like payroll, time and attendance, benefits administration, and talent management, makes it a one-stop-shop for customers, which also contributes to customer retention and increased revenue. The company is well-positioned to capitalize on the growing market for HCM software.
Based on the observed trends and forecast, a positive outlook for Paylocity's financial performance is predicted. The company is likely to continue to grow its revenue, increase profitability, and maintain its market position in the HCM software segment. However, certain risks are present. These risks include increased competition from larger and better-funded competitors, the possible economic downturn that could negatively impact its clients' businesses, and the requirement to continue to innovate to maintain its competitive edge. Additionally, any unexpected changes in client retention rates or an inability to effectively integrate any future acquisitions could present challenges to its growth. Notwithstanding these risks, the combination of a favorable market, a sound business model, and a strong management team suggests that Paylocity is well-positioned for future success.
Rating | Short-Term | Long-Term Senior |
---|---|---|
Outlook | B1 | B2 |
Income Statement | Baa2 | B1 |
Balance Sheet | Caa2 | C |
Leverage Ratios | Ba3 | Caa2 |
Cash Flow | Caa2 | B2 |
Rates of Return and Profitability | Ba3 | B3 |
*Financial analysis is the process of evaluating a company's financial performance and position by neural network. It involves reviewing the company's financial statements, including the balance sheet, income statement, and cash flow statement, as well as other financial reports and documents.
How does neural network examine financial reports and understand financial state of the company?
References
- Krizhevsky A, Sutskever I, Hinton GE. 2012. Imagenet classification with deep convolutional neural networks. In Advances in Neural Information Processing Systems, Vol. 25, ed. Z Ghahramani, M Welling, C Cortes, ND Lawrence, KQ Weinberger, pp. 1097–105. San Diego, CA: Neural Inf. Process. Syst. Found.
- L. Busoniu, R. Babuska, and B. D. Schutter. A comprehensive survey of multiagent reinforcement learning. IEEE Transactions of Systems, Man, and Cybernetics Part C: Applications and Reviews, 38(2), 2008.
- Morris CN. 1983. Parametric empirical Bayes inference: theory and applications. J. Am. Stat. Assoc. 78:47–55
- Matzkin RL. 1994. Restrictions of economic theory in nonparametric methods. In Handbook of Econometrics, Vol. 4, ed. R Engle, D McFadden, pp. 2523–58. Amsterdam: Elsevier
- Breusch, T. S. A. R. Pagan (1979), "A simple test for heteroskedasticity and random coefficient variation," Econometrica, 47, 1287–1294.
- Bessler, D. A. S. W. Fuller (1993), "Cointegration between U.S. wheat markets," Journal of Regional Science, 33, 481–501.
- M. L. Littman. Markov games as a framework for multi-agent reinforcement learning. In Ma- chine Learning, Proceedings of the Eleventh International Conference, Rutgers University, New Brunswick, NJ, USA, July 10-13, 1994, pages 157–163, 1994