AUC Score :
Short-term Tactic1 :
Dominant Strategy :
Time series to forecast n:
ML Model Testing : Deductive Inference (ML)
Hypothesis Testing : Factor
Surveillance : Major exchange and OTC
1Short-term revised.
2Time series is updated based on short-term trends.
Key Points
Based on current market dynamics, the Natural Gas Futures x3 Short Leveraged Index is predicted to experience a period of pronounced volatility. The expectation is for a significant decline in the index due to the leveraged short exposure amplifying any downward price movement in natural gas futures. This prediction carries considerable risk, with the primary concern being the potential for a sudden and substantial upward price surge in natural gas futures. Such a move would trigger substantial losses for the leveraged short position, leading to margin calls and forced liquidations that could further exacerbate the downward spiral. Moreover, the index's volatility increases the risk of rapid decay due to the effects of leverage, meaning that even with minor price fluctuations the value is subjected to rapid erosion. Market sentiment shifts, unexpected weather patterns, or geopolitical events affecting supply could all independently trigger extreme price swings that could undermine this index.About Natural Gas Futures x3 Short Levera Index
The Natural Gas Futures x3 Short Leveraged Index seeks to provide daily investment results, before fees and expenses, that correspond to three times the inverse (-3x) of the daily performance of the front-month natural gas futures contracts. This means the index is designed to profit from a decline in natural gas futures prices. It's important to understand that this is a leveraged product, meaning it amplifies the daily movements of the underlying asset. Due to this leverage, the index experiences compounded returns, which can deviate significantly from the target multiple (-3x) over periods longer than a single day.
Investors should be aware of the significant risks associated with this index. The leveraged nature exposes investors to amplified losses as well as gains. The value of the index is affected by factors influencing natural gas futures prices, including weather patterns, supply and demand dynamics, and geopolitical events. Moreover, because of the negative correlation objective, the index is likely to experience volatility drag, decreasing returns over time, particularly in volatile markets. This index is intended for sophisticated investors with a high-risk tolerance and a short-term investment horizon. Regular monitoring and adjustments are crucial to manage potential risks.
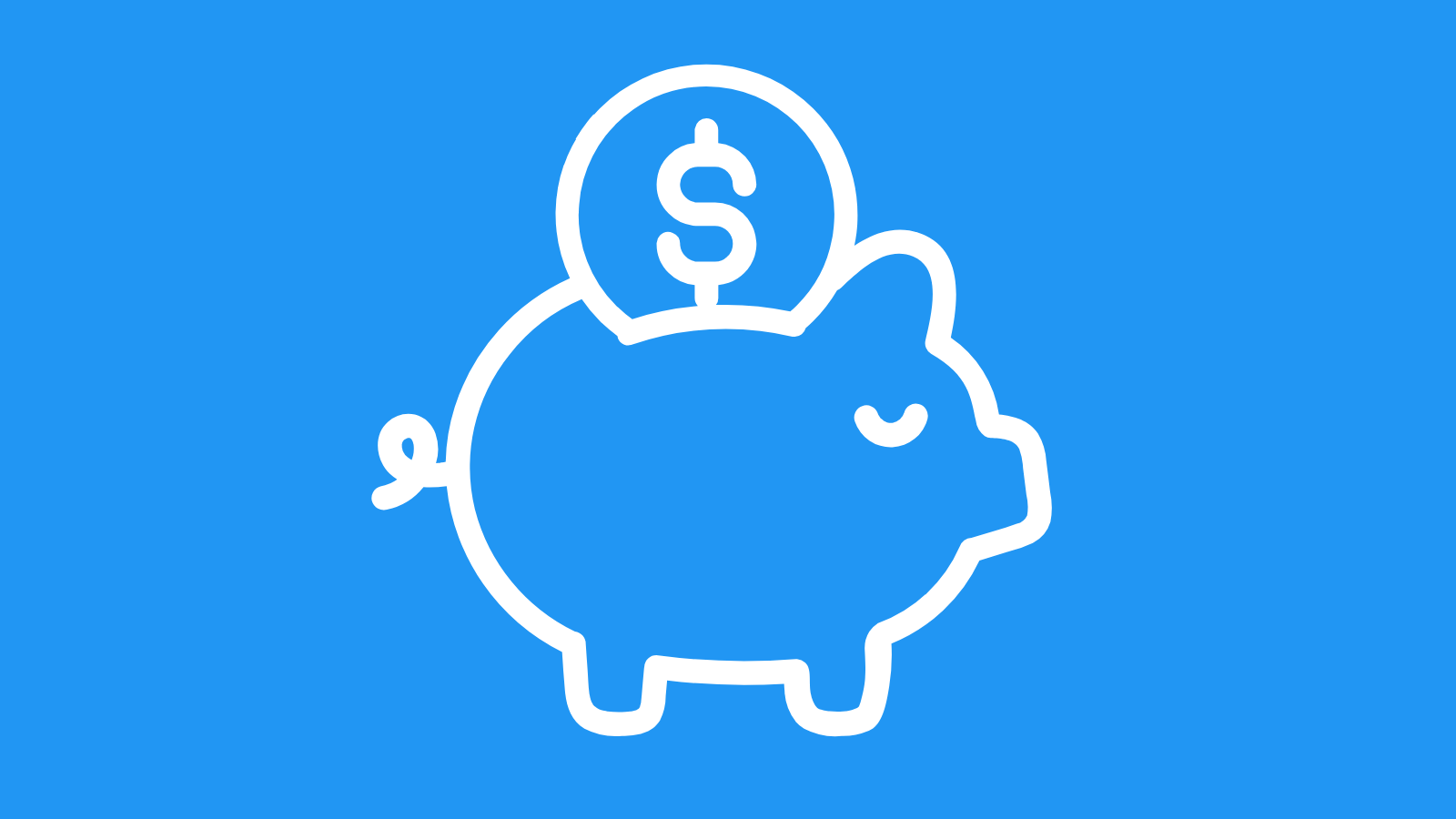
Natural Gas Futures x3 Short Leveraged Index Forecast Model
Our team of data scientists and economists has developed a machine learning model to forecast the Natural Gas Futures x3 Short Leveraged Index. The methodology employed combines time series analysis with machine learning techniques to capture the complex dynamics of the natural gas market. Initially, the model incorporates macroeconomic indicators such as crude oil prices, storage levels, weather patterns (temperature and heating degree days), and production data. These variables, which are known to significantly impact natural gas demand and supply, are preprocessed to ensure data quality and consistency. We then utilize techniques such as feature engineering to create more informative features. For instance, we calculate rolling averages and volatility measures to represent market trends and risk. The model's architecture includes Recurrent Neural Networks (RNNs), specifically Long Short-Term Memory (LSTM) networks, to effectively capture the temporal dependencies inherent in the time series data of the index.
The model training process involves splitting the historical data into training, validation, and testing sets. The training set is used to optimize the model's parameters, while the validation set helps prevent overfitting and tune hyperparameters. We have experimented with various model architectures, including different numbers of LSTM layers and hidden units, and different optimization algorithms like Adam and RMSprop. Regularization techniques, such as dropout, are implemented to further improve the generalization ability of the model. The model's performance is evaluated using metrics such as Mean Absolute Error (MAE), Mean Squared Error (MSE), and R-squared, ensuring accuracy and reliability. To account for market volatility, we implement a dynamic model that learns from real-time data to adapt to changing market conditions. Furthermore, we use backtesting to ensure the model's performance across time periods and events, providing validation to the model's robustness.
The final model provides a short-term (e.g., daily or weekly) forecast for the Natural Gas Futures x3 Short Leveraged Index. The model generates predictions of potential index movements, aiding in risk management strategies. To improve the forecast, we will continue to refine the model by incorporating additional features, such as sentiment data from news articles and social media, and exploring advanced machine learning techniques, like Transformer models. We have also developed several visualization tools to interpret model results and to highlight the primary drivers behind the forecasts. Regular re-evaluation and recalibration of the model are critical to maintaining its effectiveness in a dynamic market environment. This ensures that the model continues to reflect the latest developments of the market and maintains forecast accuracy over time.
```
ML Model Testing
n:Time series to forecast
p:Price signals of Natural Gas Futures x3 Short Levera index
j:Nash equilibria (Neural Network)
k:Dominated move of Natural Gas Futures x3 Short Levera index holders
a:Best response for Natural Gas Futures x3 Short Levera target price
For further technical information as per how our model work we invite you to visit the article below:
How do KappaSignal algorithms actually work?
Natural Gas Futures x3 Short Levera Index Forecast Strategic Interaction Table
Strategic Interaction Table Legend:
X axis: *Likelihood% (The higher the percentage value, the more likely the event will occur.)
Y axis: *Potential Impact% (The higher the percentage value, the more likely the price will deviate.)
Z axis (Grey to Black): *Technical Analysis%
Natural Gas Futures x3 Short Leveraged Index: Financial Outlook and Forecast
The Natural Gas Futures x3 Short Leveraged Index is designed to provide a daily leveraged exposure to the inverse performance of natural gas futures contracts. This means it seeks to deliver three times the opposite of the daily return of a benchmark natural gas futures index. As such, investors should understand this index's behavior is directly tied to the movement of natural gas futures prices. This index is inherently risky, as it magnifies both gains and losses. Holding it over extended periods can lead to significant erosion of capital due to daily compounding and the volatility inherent in natural gas markets. Therefore, the financial outlook for this index is heavily influenced by the supply and demand dynamics of natural gas, seasonal variations in consumption, geopolitical events affecting production and transportation, and the overall economic climate.
The performance of the underlying natural gas futures market will significantly dictate the fortunes of the x3 short leveraged index. Factors such as weather patterns, particularly the intensity of heating and cooling seasons, heavily impact demand. Increased demand during winter months, coupled with limited storage, typically leads to higher prices, benefiting the short leveraged index. Conversely, mild winters or ample storage can depress prices, leading to losses. Geopolitical events can cause significant price swings; for instance, disruptions in supply from major producers, like those arising from conflict or infrastructure damage, would likely drive prices higher. Moreover, advancements in renewable energy sources and government policies promoting alternative energy consumption can also influence the demand for natural gas. Global economic slowdowns can curb industrial demand, leading to lower prices and thus potentially, better performance for the short index.
Analyzing the historical behavior of natural gas futures is crucial for forecasting the x3 short leveraged index. Identifying key support and resistance levels in the underlying futures contracts can inform trading strategies. Furthermore, monitoring changes in natural gas inventories and storage levels provides insights into supply-demand imbalances. Examining the Commitment of Traders (COT) report, which details the positions of various market participants (e.g., commercial hedgers, large speculators, and small speculators), can reveal potential trends and sentiment shifts. Technical indicators, such as moving averages, Relative Strength Index (RSI), and other charting tools, can assist in identifying potential entry and exit points. It's essential to understand that the index's performance is highly sensitive to daily price fluctuations, requiring frequent rebalancing and close monitoring.
Considering the volatile nature of natural gas markets, the forecast for the Natural Gas Futures x3 Short Leveraged Index is inherently uncertain. The prediction depends on the expectation about a natural gas market downturn. If natural gas prices are expected to rise in the short term due to factors such as colder-than-average weather, supply disruptions, or increasing industrial demand, then the index is projected to experience losses. Conversely, if market conditions favor a decline in natural gas prices, the index has the potential for significant gains. The primary risk lies in the possibility of unexpected price surges in natural gas futures, which could lead to substantial and rapid losses. Other risks include high volatility, the costs associated with daily rebalancing, and the potential for significant price swings driven by external factors beyond simple supply and demand dynamics. Therefore, this is a speculative investment, and requires deep understanding of natural gas markets and high tolerance for risk.
Rating | Short-Term | Long-Term Senior |
---|---|---|
Outlook | B3 | B2 |
Income Statement | Ba3 | Baa2 |
Balance Sheet | B3 | C |
Leverage Ratios | B2 | Caa2 |
Cash Flow | Caa2 | Caa2 |
Rates of Return and Profitability | C | B3 |
*An aggregate rating for an index summarizes the overall sentiment towards the companies it includes. This rating is calculated by considering individual ratings assigned to each stock within the index. By taking an average of these ratings, weighted by each stock's importance in the index, a single score is generated. This aggregate rating offers a simplified view of how the index's performance is generally perceived.
How does neural network examine financial reports and understand financial state of the company?
References
- M. L. Littman. Friend-or-foe q-learning in general-sum games. In Proceedings of the Eighteenth International Conference on Machine Learning (ICML 2001), Williams College, Williamstown, MA, USA, June 28 - July 1, 2001, pages 322–328, 2001
- Canova, F. B. E. Hansen (1995), "Are seasonal patterns constant over time? A test for seasonal stability," Journal of Business and Economic Statistics, 13, 237–252.
- M. L. Littman. Markov games as a framework for multi-agent reinforcement learning. In Ma- chine Learning, Proceedings of the Eleventh International Conference, Rutgers University, New Brunswick, NJ, USA, July 10-13, 1994, pages 157–163, 1994
- Arora S, Li Y, Liang Y, Ma T. 2016. RAND-WALK: a latent variable model approach to word embeddings. Trans. Assoc. Comput. Linguist. 4:385–99
- Challen, D. W. A. J. Hagger (1983), Macroeconomic Systems: Construction, Validation and Applications. New York: St. Martin's Press.
- J. Filar, L. Kallenberg, and H. Lee. Variance-penalized Markov decision processes. Mathematics of Opera- tions Research, 14(1):147–161, 1989
- Alexander, J. C. Jr. (1995), "Refining the degree of earnings surprise: A comparison of statistical and analysts' forecasts," Financial Review, 30, 469–506.