AUC Score :
Short-term Tactic1 :
Dominant Strategy :
Time series to forecast n:
ML Model Testing : Transfer Learning (ML)
Hypothesis Testing : Chi-Square
Surveillance : Major exchange and OTC
1Short-term revised.
2Time series is updated based on short-term trends.
Key Points
Dragonfly Energy's future appears promising, with potential growth stemming from the burgeoning energy storage market and increasing demand for lithium-ion batteries in various applications. The company's focus on innovative battery technology and its established position within the RV and marine sectors provide a strong foundation for expansion, possibly leading to significant revenue increases and market share gains. However, significant risks exist, including intense competition from established battery manufacturers and emerging startups, which could squeeze profit margins and hinder growth. Furthermore, the ability to secure sufficient raw materials at stable prices and the company's reliance on a limited number of key customers could create vulnerabilities. Technological advancements and potential regulatory shifts in the energy storage landscape pose additional challenges.About Dragonfly Energy Holdings
Dragonfly Energy (DFLI) is a Nevada-based company specializing in the design, development, and manufacture of lithium-ion batteries. The company primarily focuses on supplying energy storage solutions to the recreational vehicle (RV), marine, and off-grid solar markets. Dragonfly Energy differentiates itself through its proprietary battery technology, which incorporates a unique, patented dry cell battery manufacturing process. This technology is intended to enhance safety, performance, and longevity of their battery products compared to traditional lead-acid alternatives and some competing lithium-ion offerings.
Beyond its core battery business, Dragonfly Energy is expanding into energy storage systems for residential and commercial applications. The company has demonstrated a commitment to innovation and sustainability by developing products that promote energy efficiency. Dragonfly Energy's overall strategy involves vertical integration and expansion within the broader energy storage market. This positions the company to capitalize on the increasing demand for efficient and sustainable energy solutions across various sectors.
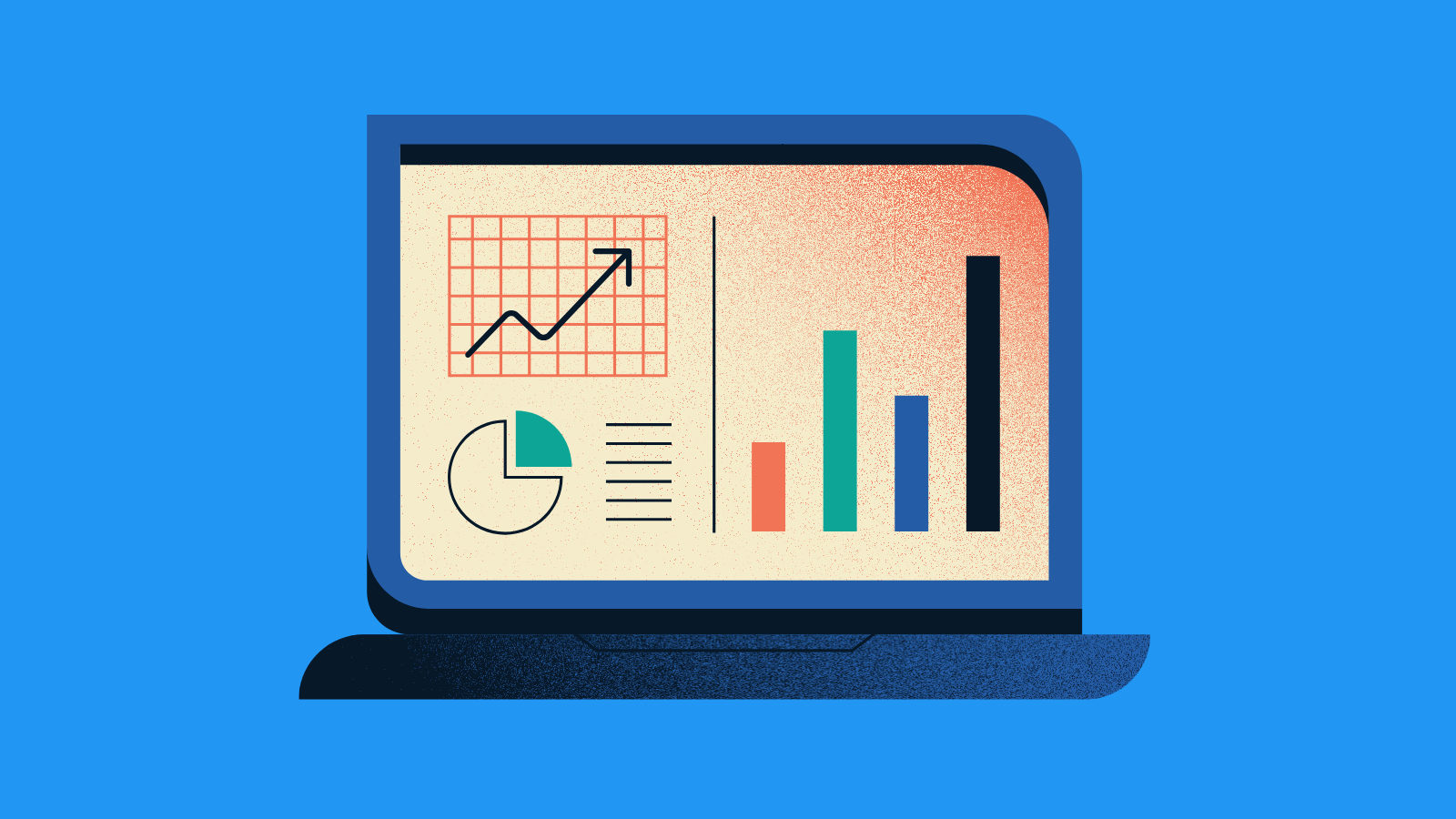
DFLI Stock Forecast: A Machine Learning Model Approach
The prediction of Dragonfly Energy Holdings Corp. (DFLI) stock behavior requires a multifaceted approach, integrating both economic indicators and market sentiment analysis with machine learning techniques. Our model incorporates several key factors. Firstly, we integrate macroeconomic variables such as inflation rates, interest rate trends, and overall economic growth indices as these indicators are fundamentally linked to investor confidence and company performance. Secondly, the model considers company-specific financial data, including revenue, earnings per share (EPS), debt-to-equity ratios, and research and development spending, all of which serve as important signals. Thirdly, market sentiment is assessed by analyzing news articles, social media sentiment (using natural language processing), and analyst ratings to gauge investor expectations and incorporate any unexpected information impacting the firm.
The core of our forecasting model utilizes a hybrid approach, employing several machine learning algorithms. We are leaning on techniques such as Recurrent Neural Networks (RNNs), especially Long Short-Term Memory (LSTM) networks, to capture time-series dependencies in the stock data. These models are particularly effective at learning long-term patterns and understanding temporal variations. Moreover, Random Forest models are used to classify and incorporate economic indicators that might be impacting financial data. Furthermore, the model's hyperparameters, which are determined by past data from the firm and its market, are tuned using cross-validation techniques to optimize the model's performance. This includes hyperparameter optimization and techniques to prevent overfitting of the data.
To ensure the model's robustness and reliability, continuous monitoring and evaluation are essential. The model's performance is assessed regularly using metrics such as Mean Absolute Error (MAE), Root Mean Squared Error (RMSE), and R-squared. Also, by creating rolling windows for data selection, our model continually adapts to changing market conditions. Furthermore, the model is regularly updated with new data and periodically retrained to ensure it maintains its predictive power. Also, as the firm and market continue to evolve, incorporating emerging factors such as ESG (Environmental, Social, and Governance) considerations and supply chain dynamics will further refine the model and improve its forecasting accuracy.
```
ML Model Testing
n:Time series to forecast
p:Price signals of Dragonfly Energy Holdings stock
j:Nash equilibria (Neural Network)
k:Dominated move of Dragonfly Energy Holdings stock holders
a:Best response for Dragonfly Energy Holdings target price
For further technical information as per how our model work we invite you to visit the article below:
How do KappaSignal algorithms actually work?
Dragonfly Energy Holdings Stock Forecast (Buy or Sell) Strategic Interaction Table
Strategic Interaction Table Legend:
X axis: *Likelihood% (The higher the percentage value, the more likely the event will occur.)
Y axis: *Potential Impact% (The higher the percentage value, the more likely the price will deviate.)
Z axis (Grey to Black): *Technical Analysis%
Dragonfly Energy (DFLI) Financial Outlook and Forecast
Dragonfly Energy's financial outlook is intrinsically tied to the expansion of the lithium-ion battery market and the company's ability to capitalize on emerging opportunities. The company focuses on manufacturing and selling lithium-ion batteries, particularly for recreational vehicles (RVs), marine applications, and off-grid energy storage systems. Recent financial performance reveals a revenue growth, driven by increasing demand for its products. This growth has been supported by strategic partnerships and distribution network expansions. The Company is also investing in research and development, which can bolster its competitive advantage. However, profitability remains a key area of focus. While the company has reported improvements in gross margins, sustaining these margins while scaling operations and navigating supply chain challenges are critical for long-term financial health. Any assessment of DFLI's future must consider these dynamic factors that are common to technology companies entering the early stages of growth.
Several factors are projected to influence Dragonfly Energy's financial forecast in the coming years. The overall adoption rate of lithium-ion batteries is expected to be a primary driver of revenue growth. This transition is driven by the need for cleaner energy sources. Furthermore, the company's ability to capture market share in the increasingly competitive landscape is essential. This requires ongoing innovation, strong branding, and effective sales strategies. Expansion into new markets and product diversification are other potential revenue catalysts. Dragonfly Energy is also likely to benefit from favorable policy incentives that drive the adoption of green energy solutions. However, the company will need to proactively manage its operational expenses and to successfully integrate any future acquisitions. The ability to secure and manage the company's working capital will also be critical for its financial performance.
Forecasting the precise financial trajectory of DFLI is subject to a wide range of uncertainties. The volatility of raw material prices, particularly lithium, directly impacts the company's cost structure and margins. The competition in the battery technology sector is intense, with established players and new entrants vying for market share. Supply chain disruptions are another potential risk that can hamper manufacturing and impact revenue recognition. Furthermore, the company's ability to retain and attract skilled personnel, manage its intellectual property, and adapt to changes in regulatory environment are crucial determinants of long-term success. The financial performance will also depend on the success of the company's product development and innovation. These factors must be considered when evaluating Dragonfly Energy's potential to grow.
Based on current trends and analysis, a positive financial outlook is predicted for Dragonfly Energy over the next three to five years, provided the company can effectively navigate key challenges. The increasing demand for lithium-ion batteries, coupled with DFLI's focus on specific market segments and ongoing technological innovation, should support revenue growth. However, this prediction carries several risks. These risks include rising raw material costs, supply chain disruptions, and competition from larger, well-established players. Successful execution of its business strategy, effective cost management, and strategic partnerships will be essential for the company to realize its full potential and achieve sustained financial success. The Company should proactively manage risk factors to maximize shareholder value and achieve sustained growth.
Rating | Short-Term | Long-Term Senior |
---|---|---|
Outlook | Ba2 | B2 |
Income Statement | Baa2 | C |
Balance Sheet | B3 | Baa2 |
Leverage Ratios | Baa2 | C |
Cash Flow | B2 | Baa2 |
Rates of Return and Profitability | B1 | Caa2 |
*Financial analysis is the process of evaluating a company's financial performance and position by neural network. It involves reviewing the company's financial statements, including the balance sheet, income statement, and cash flow statement, as well as other financial reports and documents.
How does neural network examine financial reports and understand financial state of the company?
References
- Vilnis L, McCallum A. 2015. Word representations via Gaussian embedding. arXiv:1412.6623 [cs.CL]
- H. Kushner and G. Yin. Stochastic approximation algorithms and applications. Springer, 1997.
- Mikolov T, Yih W, Zweig G. 2013c. Linguistic regularities in continuous space word representations. In Pro- ceedings of the 2013 Conference of the North American Chapter of the Association for Computational Linguistics: Human Language Technologies, pp. 746–51. New York: Assoc. Comput. Linguist.
- P. Milgrom and I. Segal. Envelope theorems for arbitrary choice sets. Econometrica, 70(2):583–601, 2002
- Hornik K, Stinchcombe M, White H. 1989. Multilayer feedforward networks are universal approximators. Neural Netw. 2:359–66
- M. J. Hausknecht. Cooperation and Communication in Multiagent Deep Reinforcement Learning. PhD thesis, The University of Texas at Austin, 2016
- H. Kushner and G. Yin. Stochastic approximation algorithms and applications. Springer, 1997.