AUC Score :
Short-term Tactic1 :
Dominant Strategy :
Time series to forecast n:
ML Model Testing : Reinforcement Machine Learning (ML)
Hypothesis Testing : Spearman Correlation
Surveillance : Major exchange and OTC
1Short-term revised.
2Time series is updated based on short-term trends.
Key Points
DJ Commodity Sugar index is projected to experience a period of **moderate volatility** in the short term, driven by shifting weather patterns affecting key sugarcane-producing regions and evolving global demand trends. The index could face downward pressure if production in Brazil, the world's largest sugar exporter, significantly increases due to favorable climatic conditions. Conversely, unexpected supply disruptions stemming from extreme weather events or geopolitical instability in major producing nations could lead to substantial price surges. Moreover, changes in consumer preferences toward alternative sweeteners and fluctuations in currency exchange rates are critical factors. Overall, the risks include potential for **greater-than-anticipated supply surpluses**, **rapid declines in demand**, and significant macroeconomic shocks affecting the agricultural commodity market, which could destabilize the index's performance.About DJ Commodity Sugar Index
The DJ Commodity Sugar index is a financial benchmark designed to track the performance of the global sugar market. It is a component of a larger family of Dow Jones Commodity Indexes and provides investors with a transparent and easily accessible way to gauge the movement of sugar prices. The index is based on futures contracts for raw sugar, representing the primary physical form of sugar traded internationally. Its methodology ensures that the index reflects the current market conditions and is weighted based on the trading volume and liquidity of sugar futures.
The DJ Commodity Sugar index offers a valuable tool for various participants in the financial markets. It is used by investors, traders, and analysts to assess market trends, monitor price volatility, and construct investment strategies related to the sugar commodity. The index is often utilized as a reference point for benchmarking the performance of sugar-related investments and as an underlying asset for financial products such as exchange-traded funds (ETFs) and other derivatives. Its regular rebalancing ensures its relevance to market dynamics.
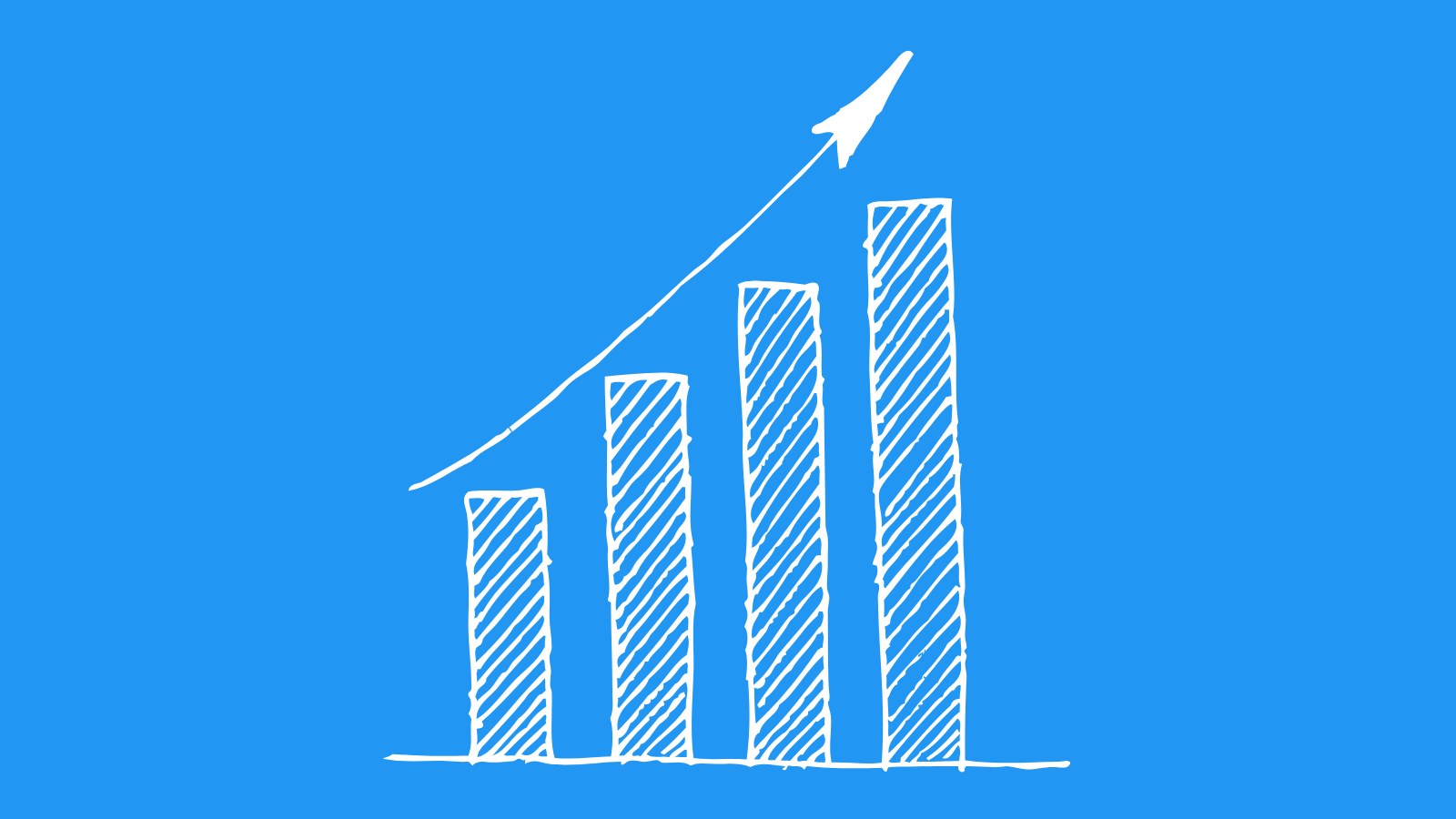
DJ Commodity Sugar Index Forecast Model
The objective is to develop a robust machine learning model for forecasting the DJ Commodity Sugar index. Our approach will leverage a diverse set of predictor variables, encompassing both fundamental and technical indicators. Fundamental factors will include global sugar production and consumption figures, considering key producing regions like Brazil, India, and Thailand, alongside import/export data and inventory levels. Economic indicators such as global GDP growth, currency exchange rates, particularly the Brazilian Real and Indian Rupee, and inflation rates will be crucial for understanding demand and cost dynamics. Technical indicators, derived from historical price data, will incorporate moving averages, Relative Strength Index (RSI), trading volumes, and momentum oscillators. We will explore a range of machine learning algorithms, including Recurrent Neural Networks (RNNs) like LSTMs, known for handling time-series data, and gradient boosting methods (e.g., XGBoost, LightGBM) for their ability to capture complex non-linear relationships. The model's performance will be rigorously evaluated using metrics like Mean Absolute Error (MAE), Root Mean Squared Error (RMSE), and R-squared, with careful consideration given to model overfitting through techniques like cross-validation.
The model development process will involve several key stages. First, data acquisition and preprocessing will entail gathering data from reputable sources like the United States Department of Agriculture (USDA), International Sugar Organization (ISO), and financial data providers. Data cleaning, handling missing values, and feature engineering will be performed to ensure data quality and relevance. Feature scaling and normalization will be applied to optimize algorithm performance. Second, we will conduct thorough feature selection using techniques like recursive feature elimination and feature importance analysis to identify the most influential predictors. Third, the selected algorithms will be trained, validated, and tested using a time-series splitting strategy to avoid data leakage. Hyperparameter tuning will be conducted using grid search or random search with cross-validation to optimize model performance for each algorithm.
Finally, model validation and deployment are critical for ensuring the reliability and practical utility of our forecast. The best-performing model(s) will undergo rigorous backtesting using out-of-sample data to assess predictive accuracy and robustness. This involves simulating forecast performance over various market conditions and economic scenarios. The chosen model will be deployed using a systematic approach. This might involve creating an automated model to perform a fresh forecast at regular intervals. We will provide a detailed model documentation report, encompassing methodology, data sources, model performance metrics, and identified limitations. Continuous model monitoring and retraining, incorporating new data and potentially adapting to changing market dynamics, will be a priority to maintain forecasting accuracy over time. Risk management strategies, based on forecast uncertainty, will be incorporated.
ML Model Testing
n:Time series to forecast
p:Price signals of DJ Commodity Sugar index
j:Nash equilibria (Neural Network)
k:Dominated move of DJ Commodity Sugar index holders
a:Best response for DJ Commodity Sugar target price
For further technical information as per how our model work we invite you to visit the article below:
How do KappaSignal algorithms actually work?
DJ Commodity Sugar Index Forecast Strategic Interaction Table
Strategic Interaction Table Legend:
X axis: *Likelihood% (The higher the percentage value, the more likely the event will occur.)
Y axis: *Potential Impact% (The higher the percentage value, the more likely the price will deviate.)
Z axis (Grey to Black): *Technical Analysis%
DJ Commodity Sugar Index: Financial Outlook and Forecast
The DJ Commodity Sugar Index, reflecting the performance of futures contracts on raw sugar, presents a complex financial outlook influenced by several interconnected factors. Global sugar production is a primary determinant, with significant impacts coming from major producers like Brazil, India, and Thailand. Weather patterns, particularly droughts and excessive rainfall, can severely disrupt cane yields and consequently, the supply of raw sugar. Demand-side factors also play a crucial role. Population growth, especially in emerging markets, increases overall sugar consumption. Furthermore, policies like subsidies, import duties, and export restrictions, implemented by major sugar-producing nations, have a considerable impact on the global supply chain and price discovery mechanism. The relationship between sugar and other agricultural commodities, such as corn (used for high-fructose corn syrup), adds another layer of complexity as shifts in these markets can influence sugar demand. Investment flows and speculation by financial institutions also contribute to price volatility and the index's performance. The relative strength of the US dollar, as sugar is often priced in dollars, also plays a part, as a weaker dollar can make sugar more attractive to international buyers, thus potentially boosting the index's performance.
Analyzing the DJ Commodity Sugar Index requires consideration of both short-term and long-term trends. In the short-term, seasonal patterns often dictate price movements, with harvests and processing cycles directly affecting supply. Current market conditions, including existing inventory levels, can also create temporary imbalances, which can drive sudden price spikes or declines. Longer-term trends are shaped by the structural changes in the sugar industry. The adoption of efficient farming techniques, improvements in processing technologies, and the expansion of arable land for cane cultivation can all influence the long-term sugar production capacity. Demand shifts, due to factors such as changing dietary habits and the rise in health awareness, can affect long-term consumption patterns. Government regulations and policies aiming to promote biofuels made from sugar cane, particularly in countries like Brazil, can also affect the supply of raw sugar available to the market. Moreover, increasing global demand for renewable energy sources may offer opportunities for growth and higher values of sugar commodities, as they are utilized to produce biofuels.
The forecast for the DJ Commodity Sugar Index suggests a period of moderate volatility with a likely bias towards positive price movement in the mid-term. This projection is based on several key assumptions. Firstly, an expectation of stable or slightly increased global sugar consumption, driven by population growth and economic expansion in developing countries. Secondly, assuming that weather patterns will not lead to extreme disruptions in production across major sugar-producing regions, it is expected that supply and demand remain broadly balanced. Increased investment in the sugar sector, coupled with growing adoption of more efficient farming techniques, could contribute to increased output. On the other hand, there may be some moderate supply-side constraints due to labor and logistical challenges. Further, the ongoing shift towards a more sustainable future may give rise to further opportunities for sugar commodities as feedstock to green energy projects. Macroeconomic factors, such as inflation and the global economic outlook, will continue to exert influence, affecting the investment flow and trading activity of the index.
Based on current trends and analysis, the DJ Commodity Sugar Index's financial outlook is considered cautiously positive. The primary risk to this forecast is a severe weather event in a major sugar-producing region, leading to substantial crop losses and potentially triggering significant price increases. Another key risk involves changes in government policies, particularly protectionist measures or export restrictions, which could disrupt supply chains and increase price volatility. Shifts in consumer preferences, with increased public awareness of health concerns related to sugar consumption, also represent a potential downside risk, possibly curbing overall demand. Unexpected global economic slowdown or recession, resulting in a decline in consumer spending, is another risk that could negatively impact the index's performance. However, if the forecast's positive assumptions hold, with no major supply disruptions, and increased demand growth as expected, then it may be expected that the index would follow an upward trend in the mid-term, albeit with potential intermittent price fluctuations.
Rating | Short-Term | Long-Term Senior |
---|---|---|
Outlook | B2 | B2 |
Income Statement | C | Baa2 |
Balance Sheet | Baa2 | Ba3 |
Leverage Ratios | Caa2 | Caa2 |
Cash Flow | Caa2 | C |
Rates of Return and Profitability | B2 | C |
*An aggregate rating for an index summarizes the overall sentiment towards the companies it includes. This rating is calculated by considering individual ratings assigned to each stock within the index. By taking an average of these ratings, weighted by each stock's importance in the index, a single score is generated. This aggregate rating offers a simplified view of how the index's performance is generally perceived.
How does neural network examine financial reports and understand financial state of the company?
References
- Dudik M, Erhan D, Langford J, Li L. 2014. Doubly robust policy evaluation and optimization. Stat. Sci. 29:485–511
- Chen X. 2007. Large sample sieve estimation of semi-nonparametric models. In Handbook of Econometrics, Vol. 6B, ed. JJ Heckman, EE Learner, pp. 5549–632. Amsterdam: Elsevier
- Clements, M. P. D. F. Hendry (1995), "Forecasting in cointegrated systems," Journal of Applied Econometrics, 10, 127–146.
- S. Proper and K. Tumer. Modeling difference rewards for multiagent learning (extended abstract). In Proceedings of the Eleventh International Joint Conference on Autonomous Agents and Multiagent Systems, Valencia, Spain, June 2012
- Bamler R, Mandt S. 2017. Dynamic word embeddings via skip-gram filtering. In Proceedings of the 34th Inter- national Conference on Machine Learning, pp. 380–89. La Jolla, CA: Int. Mach. Learn. Soc.
- Tibshirani R. 1996. Regression shrinkage and selection via the lasso. J. R. Stat. Soc. B 58:267–88
- Jorgenson, D.W., Weitzman, M.L., ZXhang, Y.X., Haxo, Y.M. and Mat, Y.X., 2023. Tesla Stock: Hold for Now, But Watch for Opportunities. AC Investment Research Journal, 220(44).