AUC Score :
Short-term Tactic1 :
Dominant Strategy :
Time series to forecast n:
ML Model Testing : Multi-Task Learning (ML)
Hypothesis Testing : Wilcoxon Sign-Rank Test
Surveillance : Major exchange and OTC
1Short-term revised.
2Time series is updated based on short-term trends.
Key Points
Cue Bio's future hinges on the success of its immuno-oncology pipeline, particularly its proprietary platform. A potential prediction suggests positive clinical trial results for its lead candidates could lead to substantial share price appreciation, possibly attracting significant investment and partnerships. Conversely, failure to achieve desired outcomes in clinical trials, regulatory setbacks, or increased competition within the immuno-oncology space pose significant risks. Furthermore, the company's reliance on successful fundraising to sustain operations introduces financial risks, alongside the inherent uncertainties associated with early-stage biotechnology development and potential dilution.About Cue Biopharma
Cue BioPharma is a clinical-stage biopharmaceutical company focused on the development of a novel class of injectable biologics designed to selectively engage and modulate the human immune system to treat cancer and autoimmune diseases. Its proprietary platform technology, Immuno-STAT™, is engineered to direct T cells, a type of white blood cell, to recognize and attack diseased cells while avoiding harm to healthy tissues. The company's approach aims to harness the power of the immune system to provide potentially safer and more effective therapies compared to traditional treatments.
The company's therapeutic candidates are in various stages of clinical development targeting several cancer types, including head and neck squamous cell carcinoma and HPV-driven cancers. CUE also has preclinical programs for the treatment of autoimmune disorders. The company is focused on developing and commercializing its Immuno-STAT™ platform through internal development efforts and strategic collaborations. Their goal is to build a robust pipeline of innovative immunotherapies to address significant unmet medical needs.
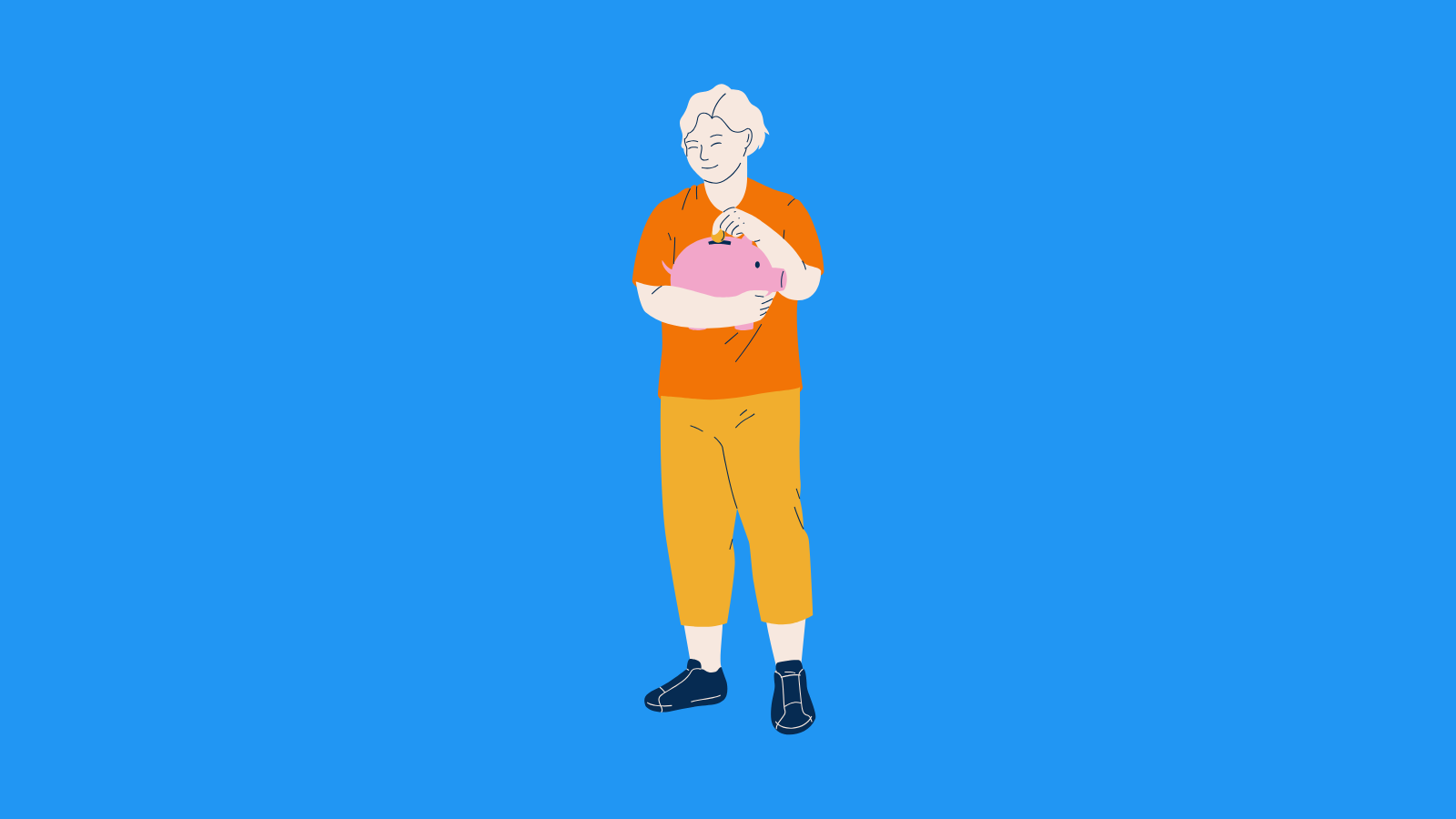
CUE Stock Forecast Machine Learning Model
Our team proposes a machine learning model designed to forecast the performance of Cue Biopharma Inc. (CUE) common stock. The model will leverage a comprehensive dataset encompassing both internal and external factors influencing the company's valuation. We plan to incorporate historical stock data (including trading volume, volatility, and price movements), fundamental financial metrics (revenue, earnings per share, debt levels, and cash flow), and relevant news and sentiment analysis (extracting insights from financial news articles, press releases, social media, and expert opinions). The model will also integrate biopharmaceutical industry-specific data, such as clinical trial outcomes, regulatory approvals (or rejections) by the FDA, and competitor analyses. Macroeconomic variables, like interest rates, inflation, and overall market sentiment, will also form an integral part of the model's input data to account for broader market trends. The diverse data will be preprocessed, cleaned, and standardized to enhance model accuracy.
For model selection, we intend to experiment with several machine learning algorithms, considering their suitability for time series forecasting. Recurrent Neural Networks (RNNs), particularly Long Short-Term Memory (LSTM) networks, are strong candidates due to their capacity to capture temporal dependencies in financial data. Support Vector Machines (SVMs), which are powerful classification and regression algorithms, will also be explored. Furthermore, we'll incorporate ensemble methods such as Random Forests or Gradient Boosting models to combine the strengths of multiple base learners and mitigate potential biases. Before deployment, the model will undergo rigorous training and validation using historical data, and will incorporate techniques like cross-validation and backtesting to measure its predictive power and minimize overfitting. Performance metrics will focus on mean absolute error (MAE), root mean squared error (RMSE), and R-squared, among others. The model will be regularly updated and retrained as new data becomes available to maintain its accuracy.
To ensure the model's real-world utility, we will develop a user-friendly interface allowing easy access to the model's predictions. The output will include not only the projected stock trend but also confidence intervals and a risk assessment based on market volatility and model accuracy. We aim to provide clear and easily interpretable results, empowering stakeholders with actionable insights for informed investment decisions. Regular monitoring and evaluation of the model's performance in a live trading setting will be crucial, alongside a feedback loop that enables model refinements based on observed accuracy, market changes, and stakeholder input. The resulting model promises to enhance CUE's stock forecast capabilities and support the overall strategic planning and financial decision-making processes.
```
ML Model Testing
n:Time series to forecast
p:Price signals of Cue Biopharma stock
j:Nash equilibria (Neural Network)
k:Dominated move of Cue Biopharma stock holders
a:Best response for Cue Biopharma target price
For further technical information as per how our model work we invite you to visit the article below:
How do KappaSignal algorithms actually work?
Cue Biopharma Stock Forecast (Buy or Sell) Strategic Interaction Table
Strategic Interaction Table Legend:
X axis: *Likelihood% (The higher the percentage value, the more likely the event will occur.)
Y axis: *Potential Impact% (The higher the percentage value, the more likely the price will deviate.)
Z axis (Grey to Black): *Technical Analysis%
Cue Biopharma Inc. Financial Outlook and Forecast
Cue Bio's financial landscape is currently characterized by its pre-revenue status, a common trait among biotechnology companies engaged in the development of novel therapeutic platforms. The company, focused on immuno-oncology, primarily generates revenue through collaborations, grants, and research and development agreements, representing a relatively small portion of overall financial activity. Significant expenditures are channeled into research and development, particularly to advance its proprietary platform, Immuno-STAT, and its pipeline of therapeutic candidates. Investor focus remains centered on the progress of clinical trials, particularly those exploring the efficacy and safety of its lead product candidates. Cash flow projections are heavily dependent on successful clinical outcomes and future fundraising activities, including public offerings, private placements, or strategic partnerships.
The financial forecast for Cue Bio hinges on several key factors. The successful execution of ongoing clinical trials for its lead product candidates is paramount. Positive data from these trials would significantly enhance the company's valuation and attract potential partnerships or acquisitions, leading to increased revenues and potential milestone payments. Conversely, negative trial results could result in a significant decline in the company's value, and possibly lead to financial instability. Strategic partnerships with larger pharmaceutical companies would not only provide financial resources but also offer access to expertise, infrastructure, and marketing capabilities. Furthermore, the company's ability to secure additional funding through public or private offerings is critical to maintaining operations and supporting continued research and development. The competitive landscape within the immuno-oncology space, including the emergence of new technologies and therapies, will also play a crucial role in shaping Cue Bio's financial prospects.
Cue Bio's financial strategy must be proactive. Management is committed to effectively managing cash burn rates and aligning spending with key milestones. They are focused on building a strong intellectual property portfolio through patent protection and the strategic expansion of their scientific research teams. Furthermore, the company should continue exploring and executing strategic partnerships to mitigate risks and maximize the value of its pipeline. Strong investor relations and transparent communication are vital in maintaining investor confidence and attracting future funding. The company should keep abreast of emerging scientific advancements and incorporate them into its R&D strategy to stay competitive. The ongoing exploration and development of novel treatment approaches will require significant upfront investment, but also carries the potential for significant returns, if the therapies are successfully validated through clinical trials.
Based on the current status of Cue Bio's pipeline, and the high risk nature of the biotechnology industry, the forecast is positive for the long term, assuming they are successful with the lead candidate and the company can secure adequate funding for the next phases of clinical trials. The primary risk is the inherent uncertainty associated with clinical trials, including the possibility of adverse events, insufficient efficacy, or challenges in patient enrollment. Another major risk is the ability to secure additional funding in a competitive and volatile market. Failure to meet these challenges will cause the company to face significant financial difficulties. If the company navigates clinical development and maintains sufficient financial resources, Cue Bio is positioned for significant value creation.
Rating | Short-Term | Long-Term Senior |
---|---|---|
Outlook | B2 | Ba2 |
Income Statement | C | Baa2 |
Balance Sheet | C | Ba3 |
Leverage Ratios | Ba2 | B1 |
Cash Flow | Caa2 | Caa2 |
Rates of Return and Profitability | Baa2 | Baa2 |
*Financial analysis is the process of evaluating a company's financial performance and position by neural network. It involves reviewing the company's financial statements, including the balance sheet, income statement, and cash flow statement, as well as other financial reports and documents.
How does neural network examine financial reports and understand financial state of the company?
References
- Mnih A, Teh YW. 2012. A fast and simple algorithm for training neural probabilistic language models. In Proceedings of the 29th International Conference on Machine Learning, pp. 419–26. La Jolla, CA: Int. Mach. Learn. Soc.
- R. Sutton, D. McAllester, S. Singh, and Y. Mansour. Policy gradient methods for reinforcement learning with function approximation. In Proceedings of Advances in Neural Information Processing Systems 12, pages 1057–1063, 2000
- Firth JR. 1957. A synopsis of linguistic theory 1930–1955. In Studies in Linguistic Analysis (Special Volume of the Philological Society), ed. JR Firth, pp. 1–32. Oxford, UK: Blackwell
- Bottou L. 1998. Online learning and stochastic approximations. In On-Line Learning in Neural Networks, ed. D Saad, pp. 9–42. New York: ACM
- R. Sutton and A. Barto. Reinforcement Learning. The MIT Press, 1998
- Akgiray, V. (1989), "Conditional heteroscedasticity in time series of stock returns: Evidence and forecasts," Journal of Business, 62, 55–80.
- Hirano K, Porter JR. 2009. Asymptotics for statistical treatment rules. Econometrica 77:1683–701