AUC Score :
Short-term Tactic1 :
Dominant Strategy :
Time series to forecast n:
ML Model Testing : Modular Neural Network (Market Volatility Analysis)
Hypothesis Testing : Lasso Regression
Surveillance : Major exchange and OTC
1Short-term revised.
2Time series is updated based on short-term trends.
Key Points
The S&P GSCI Crude Oil index is predicted to experience moderate volatility, primarily driven by fluctuations in global demand and geopolitical tensions. A modest upward trend is expected, though supply disruptions or unforeseen economic downturns could trigger sharp price corrections. Increased output from key OPEC+ nations, coupled with stronger than anticipated production from non-OPEC countries, presents a significant risk of oversupply, potentially leading to price declines. Conversely, heightened conflict in major oil-producing regions or unexpectedly robust global economic growth, especially in emerging markets, could propel prices higher, but this scenario carries the risk of inflationary pressures and decreased consumer spending. Further compounding the outlook is the accelerating transition towards renewable energy sources, which poses a long-term risk to sustained price gains for crude oil.About S&P GSCI Crude Oil Index
The S&P GSCI Crude Oil index is a commodity index that tracks the performance of a single commodity: crude oil. It is designed to provide investors with a reliable benchmark for the crude oil market. The index represents a fully collateralized investment in crude oil futures contracts. It is based on the front-month futures contract on West Texas Intermediate (WTI) crude oil traded on the New York Mercantile Exchange (NYMEX).
The S&P GSCI Crude Oil index is a widely used measure of crude oil price movements, often used by financial professionals and institutional investors. Because it is based on futures contracts, the index is sensitive to factors affecting the crude oil market like supply, demand, geopolitical events, and economic conditions. This index, along with related financial products, allows investors to gain exposure to the crude oil market and manage risk within their investment portfolios.
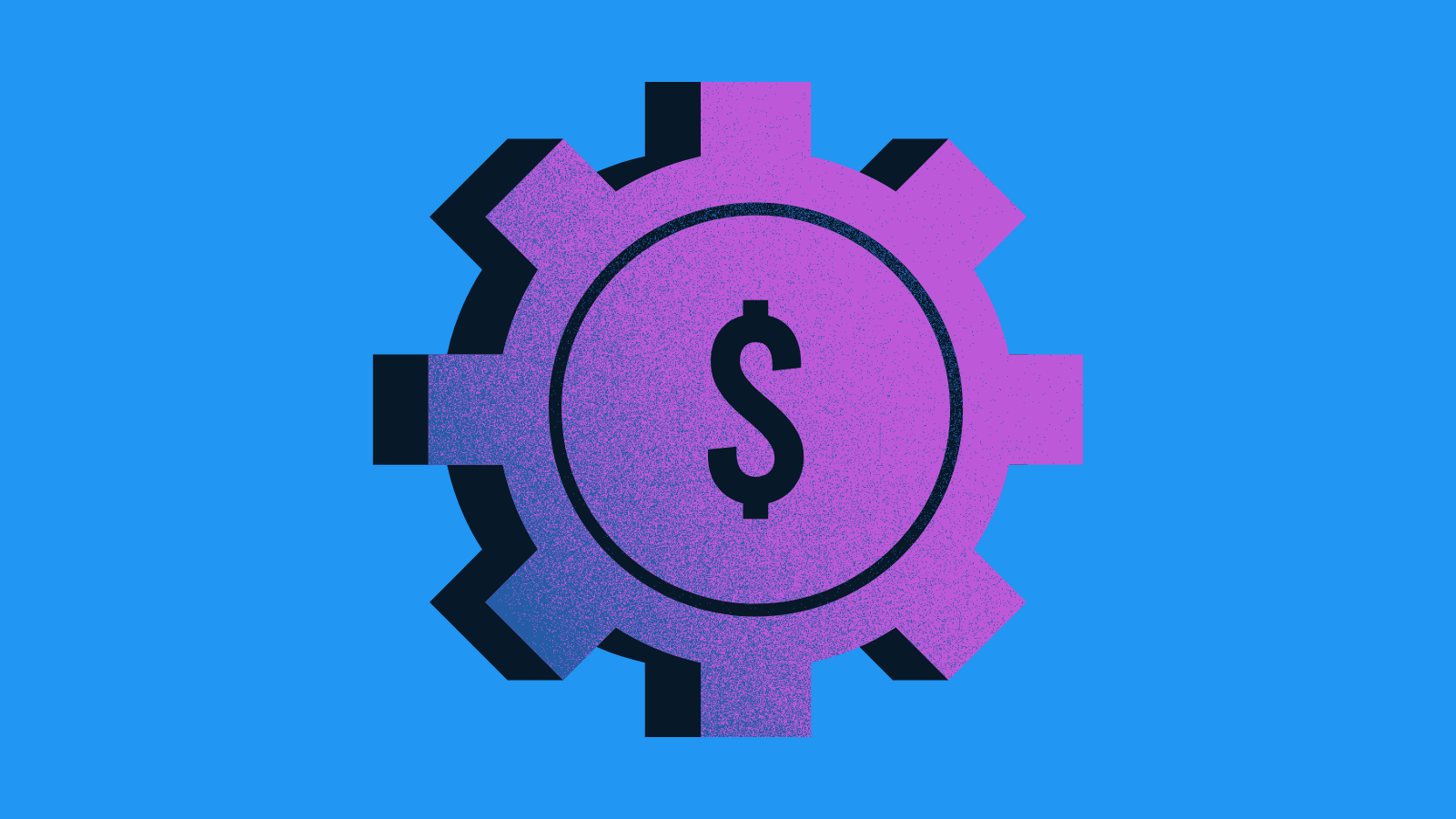
S&P GSCI Crude Oil Index Forecast Machine Learning Model
Our team of data scientists and economists has developed a machine learning model to forecast the S&P GSCI Crude Oil index. This model leverages a comprehensive dataset including historical price data, macroeconomic indicators such as global GDP growth, inflation rates, and interest rates, and geopolitical factors like production quotas from OPEC and non-OPEC countries, supply disruptions (e.g., pipeline outages, natural disasters), and demand signals (e.g., industrial production, consumer spending). We have also incorporated technical indicators like moving averages, Relative Strength Index (RSI), and Bollinger Bands to capture market sentiment and short-term trends. The model is trained on a substantial historical period, allowing us to understand the complex interplay between these various factors and their impact on the index. The dataset is preprocessed to handle missing values, outliers, and data scaling to ensure the model's reliability and accuracy.
The core of our model employs a combination of machine learning algorithms. We utilize Recurrent Neural Networks (RNNs), specifically Long Short-Term Memory (LSTM) networks, to capture the time-series nature of the data and dependencies between consecutive periods. These are combined with gradient boosting algorithms such as XGBoost to incorporate diverse features. The model structure considers hyperparameter tuning and cross-validation to prevent overfitting and improve generalization performance. Furthermore, we perform feature importance analysis to identify the most influential variables and enhance our economic understanding of oil price dynamics. The output of the model is a point forecast for the S&P GSCI Crude Oil index, along with a measure of the model's predictive uncertainty.
Our model's evaluation is rigorous, employing multiple metrics including Mean Absolute Error (MAE), Root Mean Squared Error (RMSE), and the R-squared coefficient to assess its predictive power. The backtesting is performed over a separate validation period to assess the model's performance against unseen data. The model is designed to be continuously updated as new data becomes available, and its performance is consistently monitored. We are implementing regular revisions by integrating the latest economic research and refining our feature set to improve accuracy and account for shifting market dynamics. Our team anticipates the model will be an extremely helpful tool to forecast the S&P GSCI Crude Oil index, which can give crucial information to decision-makers.
ML Model Testing
n:Time series to forecast
p:Price signals of S&P GSCI Crude Oil index
j:Nash equilibria (Neural Network)
k:Dominated move of S&P GSCI Crude Oil index holders
a:Best response for S&P GSCI Crude Oil target price
For further technical information as per how our model work we invite you to visit the article below:
How do KappaSignal algorithms actually work?
S&P GSCI Crude Oil Index Forecast Strategic Interaction Table
Strategic Interaction Table Legend:
X axis: *Likelihood% (The higher the percentage value, the more likely the event will occur.)
Y axis: *Potential Impact% (The higher the percentage value, the more likely the price will deviate.)
Z axis (Grey to Black): *Technical Analysis%
S&P GSCI Crude Oil Index: Financial Outlook and Forecast
The S&P GSCI Crude Oil Index, a widely tracked benchmark reflecting the performance of crude oil futures contracts, currently navigates a complex global landscape. Its financial outlook is predominantly shaped by supply-side dynamics, primarily emanating from the Organization of the Petroleum Exporting Countries (OPEC) and its allies, collectively known as OPEC+. Their production policies, including voluntary output cuts and adherence to quotas, play a crucial role in influencing the index's value. Moreover, geopolitical events, such as conflicts in major oil-producing regions and disruptions to supply chains, can trigger significant volatility. Demand-side factors, particularly economic growth in major consuming countries like China, India, and the United States, also exert a considerable influence. Strong economic expansion typically translates to higher demand for crude oil, potentially pushing prices upward, whereas economic downturns can have the opposite effect. Furthermore, inventories levels and storage capacities of crude oil across the world are considered by the index and can cause price fluctuations.
The forecast for the S&P GSCI Crude Oil Index over the short to medium term is subject to considerable uncertainty, largely due to the interplay of conflicting forces. On the supply side, OPEC+ has demonstrated a commitment to managing production, although the extent to which they maintain discipline and the impact of any unforeseen geopolitical events remain critical variables. Increased production from non-OPEC countries, especially in North America and other regions, could potentially offset some of the supply restraint. In terms of demand, the outlook is also mixed. While emerging markets are projected to drive future growth in crude oil consumption, the pace of their expansion will depend on a variety of economic and political factors. Developed economies, with the transition toward renewable energy sources and focus on energy efficiency are seeing a decrease in demand. Considering all the mentioned points, it will be really difficult to forecast the index in a certain period of time.
Several key factors will be instrumental in shaping the S&P GSCI Crude Oil Index's performance. The evolving geopolitical landscape, including conflicts and political instability, will be a major driver, particularly in areas of significant oil production. The global economy's performance, especially the growth rates of major consuming nations, will strongly affect the demand for crude oil. OPEC+'s adherence to its production policies and any changes in their strategies will directly impact the supply side. The rate of technological advancements in areas such as renewable energy and energy efficiency will also play a role, potentially influencing long-term demand. Additionally, the emergence of any new and more efficient refining methods will cause disruptions in the market. Finally, shifts in global economic sentiment and investor risk appetite can affect the index value as investors consider crude oil market risk.
Based on the current analysis, a mixed outlook for the S&P GSCI Crude Oil Index is foreseen. A base case suggests a moderately positive scenario, driven by the likelihood of persistent OPEC+ supply management, a growing, even if at times uneven, demand from emerging markets, and the continued need for oil during the transition to clean energy. However, this positive forecast is not without risks. Downside risks include a significant global economic slowdown or recession, which would decrease demand, or an unexpected increase in non-OPEC production. Geopolitical shocks, such as major disruptions to oil supply caused by conflicts or natural disasters, could either significantly increase or decrease the index value depending on their impact on supply. The pace of the energy transition towards renewable energy and the adoption of electric vehicles could also decrease oil demand. Therefore, investors should maintain a cautious approach and closely monitor these key factors.
```
Rating | Short-Term | Long-Term Senior |
---|---|---|
Outlook | Ba3 | Ba3 |
Income Statement | Baa2 | Baa2 |
Balance Sheet | Ba3 | B1 |
Leverage Ratios | Ba3 | B1 |
Cash Flow | Caa2 | Ba3 |
Rates of Return and Profitability | Baa2 | C |
*An aggregate rating for an index summarizes the overall sentiment towards the companies it includes. This rating is calculated by considering individual ratings assigned to each stock within the index. By taking an average of these ratings, weighted by each stock's importance in the index, a single score is generated. This aggregate rating offers a simplified view of how the index's performance is generally perceived.
How does neural network examine financial reports and understand financial state of the company?
References
- Andrews, D. W. K. (1993), "Tests for parameter instability and structural change with unknown change point," Econometrica, 61, 821–856.
- Athey S, Mobius MM, Pál J. 2017c. The impact of aggregators on internet news consumption. Unpublished manuscript, Grad. School Bus., Stanford Univ., Stanford, CA
- Byron, R. P. O. Ashenfelter (1995), "Predicting the quality of an unborn grange," Economic Record, 71, 40–53.
- Belloni A, Chernozhukov V, Hansen C. 2014. High-dimensional methods and inference on structural and treatment effects. J. Econ. Perspect. 28:29–50
- Dudik M, Langford J, Li L. 2011. Doubly robust policy evaluation and learning. In Proceedings of the 28th International Conference on Machine Learning, pp. 1097–104. La Jolla, CA: Int. Mach. Learn. Soc.
- Jorgenson, D.W., Weitzman, M.L., ZXhang, Y.X., Haxo, Y.M. and Mat, Y.X., 2023. MRNA: The Next Big Thing in mRNA Vaccines. AC Investment Research Journal, 220(44).
- Tibshirani R. 1996. Regression shrinkage and selection via the lasso. J. R. Stat. Soc. B 58:267–88