AUC Score :
Short-term Tactic1 :
Dominant Strategy :
Time series to forecast n:
ML Model Testing : Active Learning (ML)
Hypothesis Testing : Sign Test
Surveillance : Major exchange and OTC
1Short-term revised.
2Time series is updated based on short-term trends.
Key Points
BRT Apartments is anticipated to experience moderate growth, driven by its diversified portfolio of multifamily properties across various US markets. Occupancy rates are expected to remain stable, benefiting from sustained demand for rental housing. However, increased interest rates pose a significant risk, potentially impacting its ability to refinance existing debt and slowing down future acquisitions. Economic slowdown in key markets where the company operates also presents a risk, which might negatively affect occupancy and rental income. Competition from other real estate investment trusts and developers could also restrict BRT's ability to expand or raise rents. Furthermore, changes in government regulations regarding the real estate market and rent control measures are crucial factors to monitor, as these could introduce uncertainty and impact the profitability of the company.About BRT Apartments Corp.
BRT Apartments Corp. (BRT), a Maryland-based real estate investment trust (REIT), primarily focuses on acquiring, owning, and operating multifamily apartment properties. The company strategically invests in diversified markets across the United States, aiming to generate income for shareholders through rental revenue and potential property appreciation. BRT typically manages its portfolio of apartment communities, handling all aspects of property management, from leasing and maintenance to capital improvements.
BRT's operational approach emphasizes a value-add strategy, often acquiring properties with the intention of renovating and enhancing them to increase rental income and property values. The company's business model centers around identifying and capitalizing on opportunities within the multifamily housing sector. BRT is committed to providing quality housing and generating consistent returns for its investors by adhering to prudent financial management practices within its investment strategy.
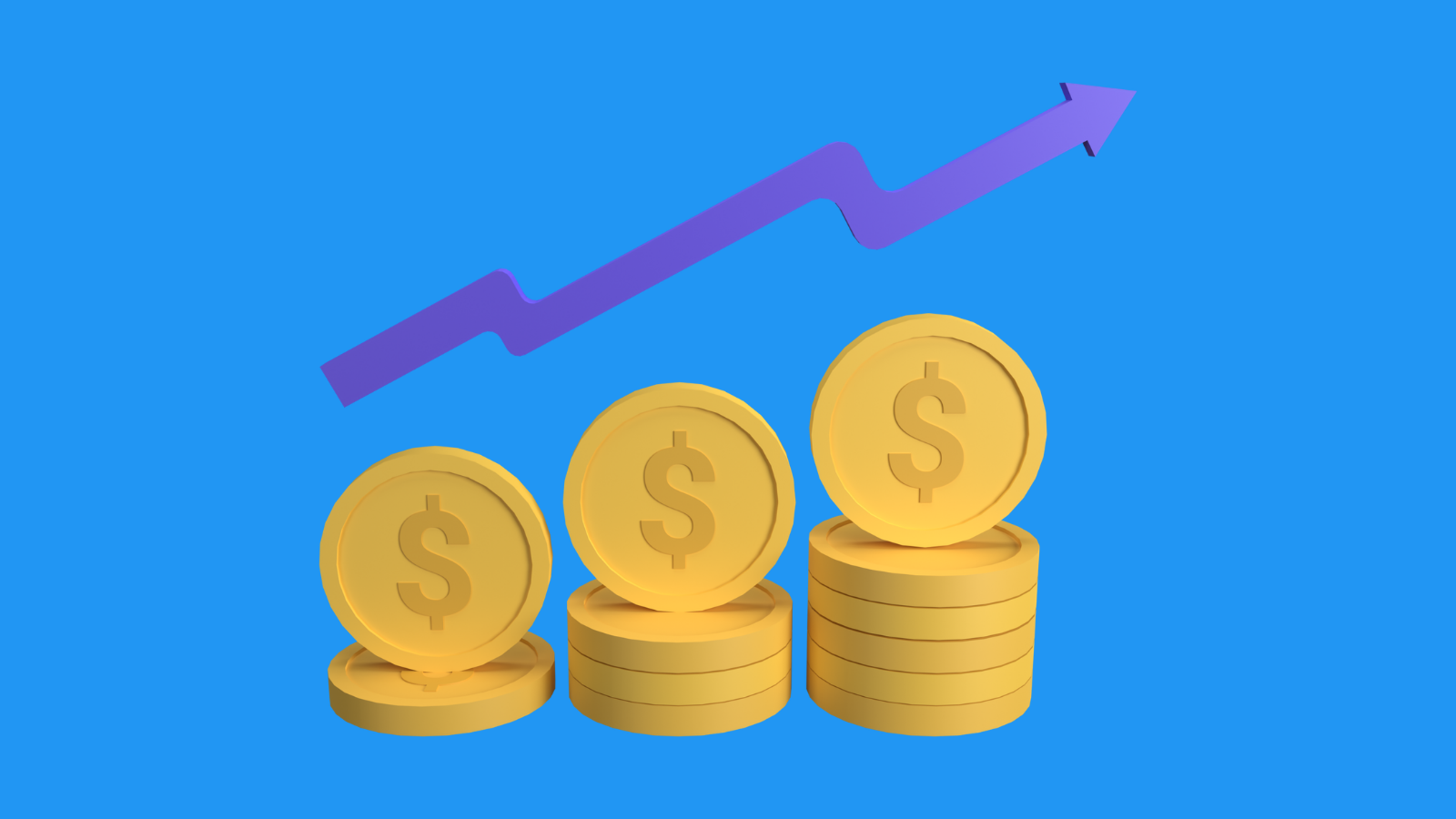
BRT Apartments Corp. (BRT) Stock Forecast Model
Our team, comprised of data scientists and economists, has developed a machine learning model to forecast the performance of BRT Apartments Corp. (BRT) common stock. The model integrates a diverse range of financial and macroeconomic indicators to predict future trends. Key financial variables include BRT's revenue, earnings per share (EPS), debt-to-equity ratio, and dividend yield. We also incorporate real estate market indicators specific to BRT's geographic footprint, such as occupancy rates, rental growth, and property values. Crucially, the model considers macroeconomic factors like interest rates, inflation, consumer confidence, and GDP growth, as these can significantly impact the real estate sector and investor sentiment. The data is cleaned, transformed, and analyzed to identify correlations and patterns that influence BRT's stock behavior.
We employ a suite of machine learning algorithms, including time-series analysis methods like ARIMA and Prophet, alongside more sophisticated techniques such as recurrent neural networks (RNNs) and gradient boosting models. Each algorithm is rigorously trained and validated using historical BRT stock data and corresponding economic indicators. Model performance is evaluated using metrics such as mean absolute error (MAE), root mean squared error (RMSE), and R-squared, ensuring accuracy and robustness. The optimal model is selected based on its predictive power and generalization capability across diverse market conditions. Ensemble methods are also being explored to harness the strengths of multiple algorithms, further enhancing the model's predictive accuracy. We use both a training and validation set to ensure the model is not overfitting.
The final output of our model provides a forecast for BRT's stock performance, incorporating probabilistic estimates. This includes predicting price movement direction, and potential trading ranges. The model is designed to be dynamic and adaptable. We will continuously monitor model performance, retraining it periodically with the latest data and recalibrating its parameters as market conditions evolve. This ensures the model remains current and reliable. Furthermore, we offer risk assessments and interpretations for each forecast, accompanied by scenario analyses, to consider a range of potential economic developments. We intend to provide stakeholders with the appropriate confidence, by presenting data in an understandable format.
```
ML Model Testing
n:Time series to forecast
p:Price signals of BRT Apartments Corp. stock
j:Nash equilibria (Neural Network)
k:Dominated move of BRT Apartments Corp. stock holders
a:Best response for BRT Apartments Corp. target price
For further technical information as per how our model work we invite you to visit the article below:
How do KappaSignal algorithms actually work?
BRT Apartments Corp. Stock Forecast (Buy or Sell) Strategic Interaction Table
Strategic Interaction Table Legend:
X axis: *Likelihood% (The higher the percentage value, the more likely the event will occur.)
Y axis: *Potential Impact% (The higher the percentage value, the more likely the price will deviate.)
Z axis (Grey to Black): *Technical Analysis%
BRT Apartments Corp. (MD) Common Stock: Financial Outlook and Forecast
The financial outlook for BRT, a real estate investment trust (REIT), is shaped by several key factors that will influence its performance in the coming periods. The company's core business of owning and operating multi-family apartment properties is relatively stable, providing a consistent stream of rental income. This stability, however, is interwoven with the broader economic landscape and the trends within the real estate market. BRT's success hinges on its ability to maintain high occupancy rates, manage operating expenses efficiently, and strategically position itself within competitive markets. The company's geographic diversification, with properties spread across several states, offers a degree of insulation against regional economic downturns. Furthermore, BRT's ability to secure favorable financing terms and navigate potential interest rate fluctuations will significantly impact its profitability and future growth trajectory. Investment decisions, including acquisitions, renovations, and property sales, are critical components of its financial strategy, and are closely monitored by investors.
Current forecasts for BRT anticipate moderate growth in key financial metrics. Factors supporting a positive outlook include steady demand for rental housing, driven by demographic trends and affordability challenges in the homeownership market. The company is likely to benefit from continued population growth in its target markets. Increases in rental income depend heavily on the company's ability to raise rents without significantly impacting occupancy levels. This is particularly important in today's environment of high inflation. BRT's ability to maintain its dividend payouts is a key indicator of its financial health, with investors often viewing dividend yield as a crucial element of their investment strategy. The company's management team must effectively balance growth initiatives with financial prudence to maintain a healthy balance sheet. Any operational inefficiencies or unexpected disruptions could impact cash flow and earnings.
Looking ahead, the REIT faces a range of potential challenges that could impact its financial performance. Rising interest rates pose a risk, potentially increasing borrowing costs and affecting the valuation of its properties. Economic slowdowns, potentially impacting consumer confidence and disposable incomes, could lead to a decline in rental demand or reduced ability to increase rents. The competitive landscape in the multi-family sector is also intense, with increasing development activity in many markets, potentially creating oversupply situations and downward pressure on rents. Further, any shifts in government regulations related to housing or rent control could have a direct impact on the company's operations. The company's ability to manage these risks, adapt to market changes, and execute its strategic plan effectively will be crucial for long-term success.
In conclusion, the financial forecast for BRT is cautiously optimistic. The company's stable business model, diversified portfolio, and focus on strategic investments position it for moderate growth in the near term. The primary prediction is for continued, albeit modest, increases in revenue and net income, alongside consistent dividend payments. However, this forecast is subject to risks including rising interest rates, potential economic slowdowns, and increased competition in the rental market. Successfully mitigating these risks through proactive financial management, efficient operations, and strategic decision-making is essential for realizing the predicted positive financial outcomes and maintaining investor confidence. Investors should closely monitor market conditions and the company's responses to any emerging challenges to assess its ongoing financial health and the sustainability of its returns.
Rating | Short-Term | Long-Term Senior |
---|---|---|
Outlook | B1 | Ba3 |
Income Statement | Ba3 | Caa2 |
Balance Sheet | Baa2 | Baa2 |
Leverage Ratios | B3 | Baa2 |
Cash Flow | Caa2 | Caa2 |
Rates of Return and Profitability | Ba3 | B2 |
*Financial analysis is the process of evaluating a company's financial performance and position by neural network. It involves reviewing the company's financial statements, including the balance sheet, income statement, and cash flow statement, as well as other financial reports and documents.
How does neural network examine financial reports and understand financial state of the company?
References
- Chen, C. L. Liu (1993), "Joint estimation of model parameters and outlier effects in time series," Journal of the American Statistical Association, 88, 284–297.
- Alpaydin E. 2009. Introduction to Machine Learning. Cambridge, MA: MIT Press
- Mnih A, Kavukcuoglu K. 2013. Learning word embeddings efficiently with noise-contrastive estimation. In Advances in Neural Information Processing Systems, Vol. 26, ed. Z Ghahramani, M Welling, C Cortes, ND Lawrence, KQ Weinberger, pp. 2265–73. San Diego, CA: Neural Inf. Process. Syst. Found.
- Abadir, K. M., K. Hadri E. Tzavalis (1999), "The influence of VAR dimensions on estimator biases," Econometrica, 67, 163–181.
- S. Bhatnagar and K. Lakshmanan. An online actor-critic algorithm with function approximation for con- strained Markov decision processes. Journal of Optimization Theory and Applications, 153(3):688–708, 2012.
- N. B ̈auerle and J. Ott. Markov decision processes with average-value-at-risk criteria. Mathematical Methods of Operations Research, 74(3):361–379, 2011
- Dietterich TG. 2000. Ensemble methods in machine learning. In Multiple Classifier Systems: First International Workshop, Cagliari, Italy, June 21–23, pp. 1–15. Berlin: Springer