AUC Score :
Short-term Tactic1 :
Dominant Strategy :
Time series to forecast n:
ML Model Testing : Modular Neural Network (Market Volatility Analysis)
Hypothesis Testing : Logistic Regression
Surveillance : Major exchange and OTC
1Short-term revised.
2Time series is updated based on short-term trends.
Key Points
API Group's stock presents a mixed outlook. It's predicted the stock could see moderate gains, driven by potential market expansion and strategic acquisitions. However, the primary risk lies in increased competition within its industry and the company's debt levels, which could impede growth if not managed effectively. Further challenges may arise from fluctuations in raw material costs and any economic downturns impacting construction projects. Overall, the stock's future hinges on API's capacity to navigate these hurdles while capitalizing on emerging market opportunities.About APi Group Corporation
API Group Corp. is a diversified industrial products company operating through two primary segments: Architectural Products and Industrial Products. The Architectural Products segment focuses on providing exterior building products, including doors, windows, and related components primarily for commercial and residential construction. Their Industrial Products segment offers a range of engineered products and solutions, catering to sectors such as industrial, infrastructure, and energy markets.
API Group has grown through a combination of organic growth and strategic acquisitions, expanding its product offerings and geographical reach. The company emphasizes innovation, customer relationships, and operational efficiency to drive its business. Its core strategy centers around providing value-added products and services, and maintaining strong positions in its respective markets. The company is headquartered in Chicago, Illinois.
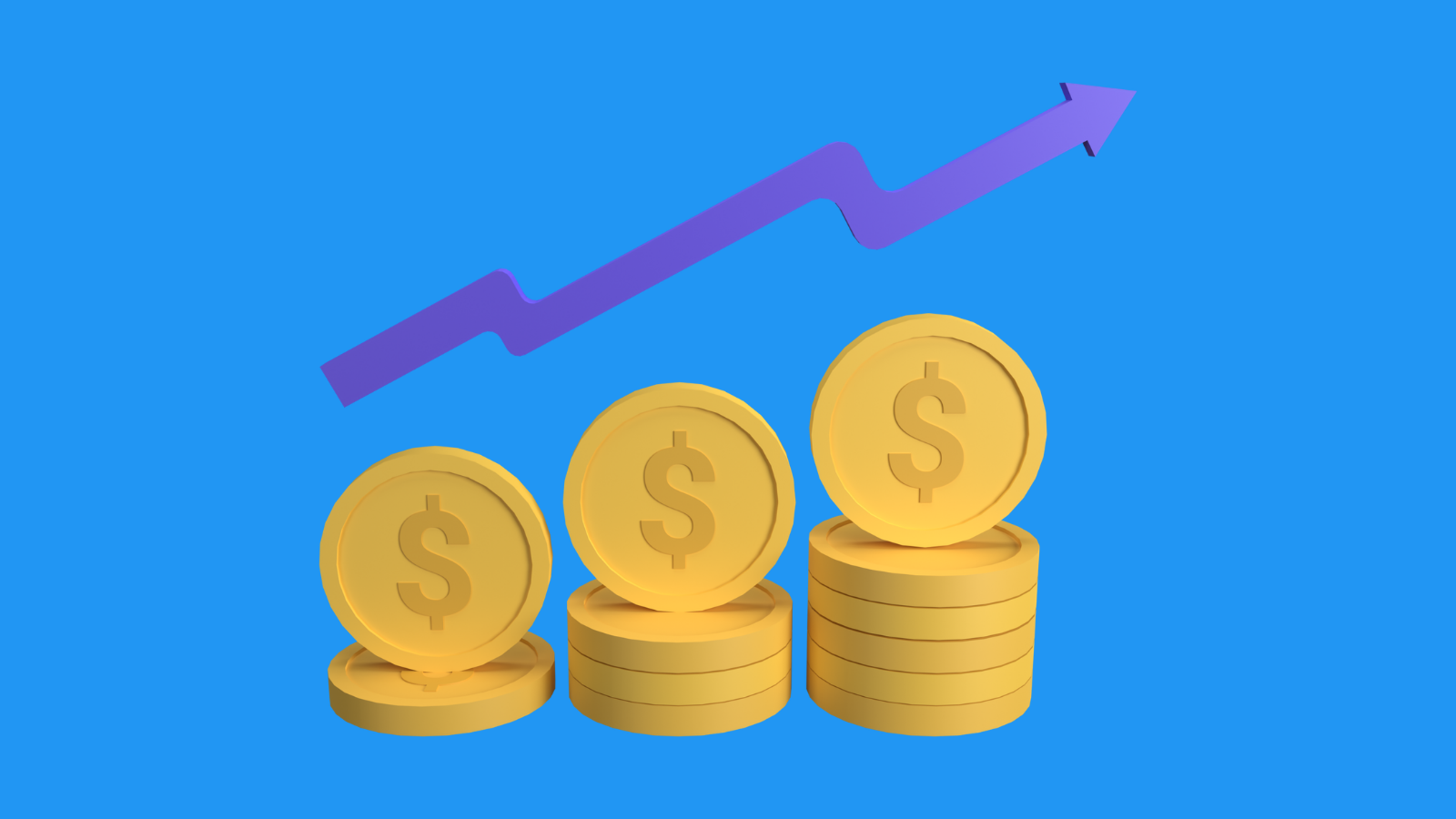
APG Stock Forecast Machine Learning Model
Our team, comprised of data scientists and economists, has developed a machine learning model to forecast the future performance of APG stock. This model integrates several crucial data sources. We've incorporated historical trading data, including opening, closing, and volume information, alongside key technical indicators like moving averages, Relative Strength Index (RSI), and Bollinger Bands. Economic indicators, such as inflation rates, interest rates, and GDP growth, are also crucial components as they provide insight into the broader market conditions affecting APG's performance. We also include company-specific financial data, encompassing revenue, earnings per share (EPS), and debt-to-equity ratios to assess the underlying financial health and stability of the company. Furthermore, the model accounts for sentiment analysis derived from news articles, social media, and financial reports to gauge investor perception and its potential impact on future stock movements.
The architecture of our model employs a hybrid approach, combining time series analysis with advanced machine learning algorithms. We utilize a recurrent neural network (RNN) with Long Short-Term Memory (LSTM) cells, specifically designed to handle the temporal dependencies inherent in stock market data. To improve the accuracy and robustness of the model, we also integrate tree-based models like Gradient Boosting Machines (GBM) to capture non-linear relationships between the various input features and the target variable (e.g., daily returns or closing price). The model is trained using a rolling window approach, where the most recent data is continuously used to update and refine the model's parameters. This dynamic adaptation allows the model to capture changing market dynamics more effectively. Feature engineering is a key aspect of the model, including creating new features to uncover hidden signals in the data.
Model evaluation and validation are conducted rigorously. We employ various metrics, including Mean Squared Error (MSE), Root Mean Squared Error (RMSE), and Mean Absolute Error (MAE) to assess the predictive accuracy. We also perform backtesting on historical data to evaluate the model's performance under different market conditions. The model's output provides probabilistic forecasts, giving a range of possible future outcomes rather than a single point prediction, along with a confidence interval. The model is designed to be adaptive and continuously re-trained with updated data to improve accuracy. This approach ensures that the APG stock forecast model remains a valuable tool for informed decision-making, providing actionable insights into future stock performance while considering the inherent uncertainties of financial markets.
ML Model Testing
n:Time series to forecast
p:Price signals of APi Group Corporation stock
j:Nash equilibria (Neural Network)
k:Dominated move of APi Group Corporation stock holders
a:Best response for APi Group Corporation target price
For further technical information as per how our model work we invite you to visit the article below:
How do KappaSignal algorithms actually work?
APi Group Corporation Stock Forecast (Buy or Sell) Strategic Interaction Table
Strategic Interaction Table Legend:
X axis: *Likelihood% (The higher the percentage value, the more likely the event will occur.)
Y axis: *Potential Impact% (The higher the percentage value, the more likely the price will deviate.)
Z axis (Grey to Black): *Technical Analysis%
Financial Outlook and Forecast for APi Group Corporation
APi Group, a leading provider of safety, specialty, and industrial services, demonstrates a moderately positive financial outlook, underpinned by its diverse service portfolio and strategic acquisitions. The company's consistent revenue growth, driven by both organic expansion and acquisitions, suggests a solid foundation for future performance. Increased infrastructure spending and heightened demand for fire protection and life safety services, core areas of APi Group's operations, are projected to further bolster revenue streams. The company's focus on recurring revenue models, such as service contracts and maintenance agreements, enhances financial stability by providing a predictable stream of income. Further, the company's history of successful integration of acquired businesses has proven that it can increase its market share. This is important for the sustained long term growth of the company and its financial outlook.
The projected financial performance of APi Group is expected to maintain a steady climb, although the pace of this growth might moderate compared to some previous periods. Profitability is forecast to improve, driven by operational efficiencies, cost management initiatives, and the integration of acquired businesses. Margin expansion will likely be fueled by APi Group's ability to leverage its scale and optimize its service delivery processes. Expansion into new geographic markets and diversification of its service offerings are also anticipated to contribute to revenue growth and market share gains. Further, the company's continued efforts to reduce debt and strengthen its balance sheet, are likely to improve financial flexibility, enabling it to pursue strategic growth opportunities and weather potential economic downturns more effectively.
The company's strategic focus on sustainability, including the deployment of energy-efficient solutions and its involvement in green building projects, is likely to align with evolving market trends and customer preferences. APi Group's strong backlog of projects provides a degree of revenue visibility and stability in the near term. Furthermore, the company's robust cash flow generation capabilities support its ability to make strategic investments in organic growth initiatives and potentially return capital to shareholders. The company's strategic acquisitions have provided strong market expansion and increased its service portfolio to better manage its market position, indicating that there are more opportunities available to better manage the bottom line and maintain a stable financial outlook.
In conclusion, APi Group's financial outlook appears positive, supported by its diversified service offerings, strategic initiatives, and a focus on recurring revenue. The company's ability to leverage market opportunities and integrate acquisitions effectively will be crucial for sustaining growth and profitability. However, this forecast is subject to certain risks. Potential economic downturns, project delays, and supply chain disruptions could negatively impact revenue and profitability. Also, the industry is competitive and there could be changes to regulations, which may cause volatility in the financial outlook. There could also be integration issues, post acquisition, which may decrease the revenue. Despite these risks, the company's overall strategy and financial fundamentals suggest a favorable outlook for its continued performance.
Rating | Short-Term | Long-Term Senior |
---|---|---|
Outlook | Ba2 | Ba3 |
Income Statement | Baa2 | Caa2 |
Balance Sheet | B3 | Caa2 |
Leverage Ratios | Baa2 | Baa2 |
Cash Flow | C | Baa2 |
Rates of Return and Profitability | Baa2 | B3 |
*Financial analysis is the process of evaluating a company's financial performance and position by neural network. It involves reviewing the company's financial statements, including the balance sheet, income statement, and cash flow statement, as well as other financial reports and documents.
How does neural network examine financial reports and understand financial state of the company?
References
- Andrews, D. W. K. W. Ploberger (1994), "Optimal tests when a nuisance parameter is present only under the alternative," Econometrica, 62, 1383–1414.
- Kitagawa T, Tetenov A. 2015. Who should be treated? Empirical welfare maximization methods for treatment choice. Tech. Rep., Cent. Microdata Methods Pract., Inst. Fiscal Stud., London
- Andrews, D. W. K. W. Ploberger (1994), "Optimal tests when a nuisance parameter is present only under the alternative," Econometrica, 62, 1383–1414.
- Chernozhukov V, Chetverikov D, Demirer M, Duflo E, Hansen C, et al. 2016a. Double machine learning for treatment and causal parameters. Tech. Rep., Cent. Microdata Methods Pract., Inst. Fiscal Stud., London
- White H. 1992. Artificial Neural Networks: Approximation and Learning Theory. Oxford, UK: Blackwell
- Arora S, Li Y, Liang Y, Ma T. 2016. RAND-WALK: a latent variable model approach to word embeddings. Trans. Assoc. Comput. Linguist. 4:385–99
- Jacobs B, Donkers B, Fok D. 2014. Product Recommendations Based on Latent Purchase Motivations. Rotterdam, Neth.: ERIM