AUC Score :
Short-term Tactic1 :
Dominant Strategy :
Time series to forecast n:
ML Model Testing : Modular Neural Network (Financial Sentiment Analysis)
Hypothesis Testing : ElasticNet Regression
Surveillance : Major exchange and OTC
1Short-term revised.
2Time series is updated based on short-term trends.
Key Points
The TR/CC CRB Nickel index is anticipated to experience volatility in the coming period. Factors such as global economic conditions, supply chain disruptions, and fluctuating demand for nickel in various industrial applications are expected to exert pressure on pricing. Potential price increases may be driven by tightening supply, while a decline could result from a global economic slowdown or a surplus in supply. The uncertainty surrounding these factors introduces substantial risk to any investment strategy, with potential for significant gains or losses depending on the trajectory of the index.About TR/CC CRB Nickel Index
The TR/CC CRB Nickel index is a benchmark measure of the price of nickel, a crucial metal in various industrial applications. It's designed to track the spot and futures market price movements of nickel, providing a consistent and standardized way to assess the metal's value. The index considers multiple sources and trading locations to reflect the overall market sentiment and pricing trends. This allows for a comprehensive overview of the nickel market, essential for investors, traders, and market analysts.
Key aspects of the TR/CC CRB Nickel index include its calculation methodology, which typically uses a weighted average of prices from different exchanges and contracts. This weighted average accounts for the volume traded on each market, ensuring that the index accurately represents the prevailing market price. The index is a significant indicator of the supply and demand dynamics in the nickel market. Any significant movements in the index can signal shifts in investor confidence or underlying economic trends impacting the nickel industry.
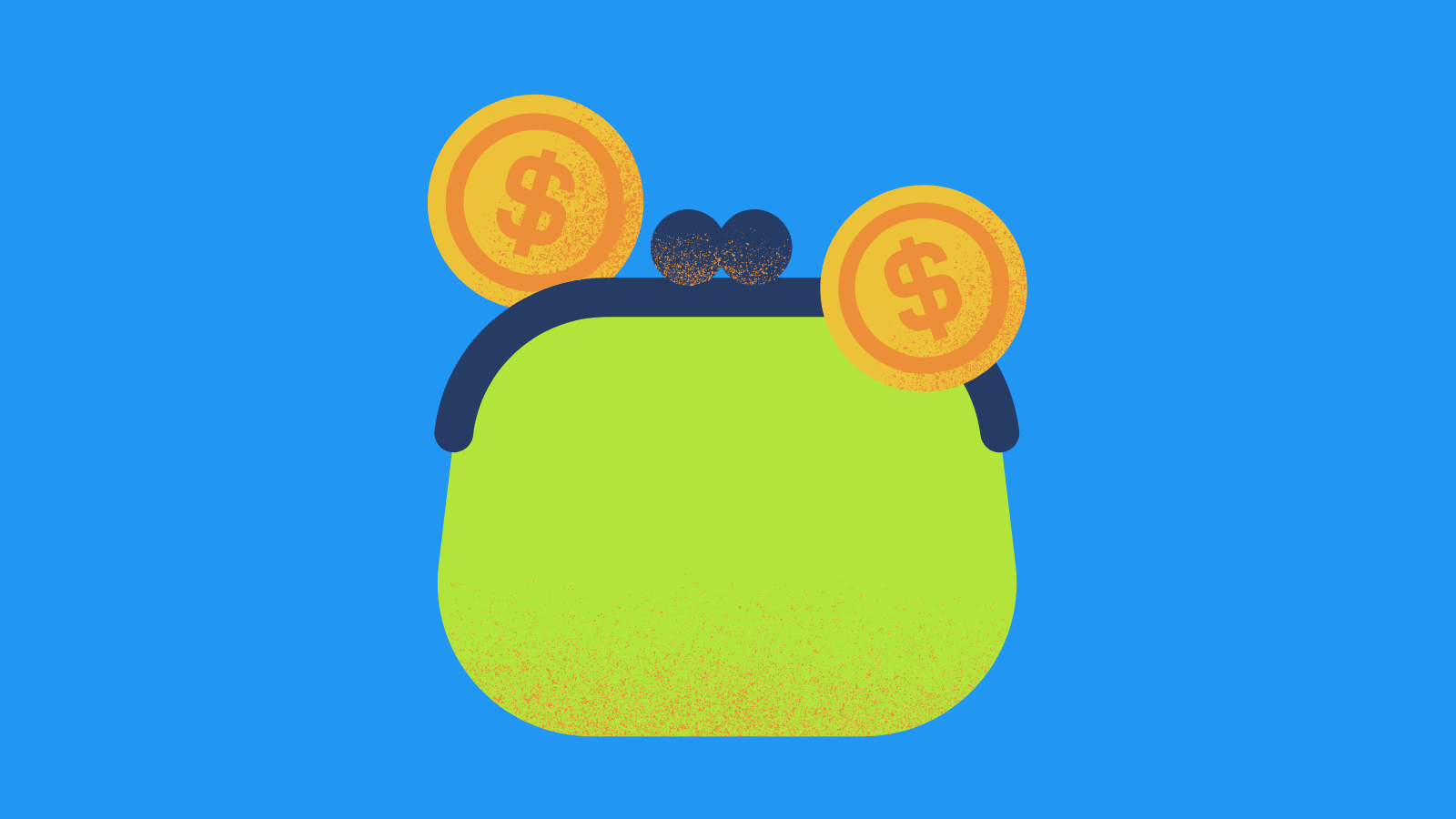
TR/CC CRB Nickel Index Price Forecast Model
To develop a robust forecasting model for the TR/CC CRB Nickel index, a multi-faceted approach was employed integrating various data sources and machine learning techniques. A comprehensive dataset encompassing historical price fluctuations, macroeconomic indicators (e.g., global GDP growth, interest rates, inflation), geopolitical events (e.g., trade wars, political instability), and commodity market trends was meticulously collected and preprocessed. Crucial features were selected based on their statistically significant correlation with the TR/CC CRB Nickel index's historical performance through correlation analysis and feature importance techniques. Data cleaning, normalization, and handling of missing values were rigorously undertaken to ensure data quality and prevent bias in the model. Time series analysis was employed to identify seasonality and other temporal patterns inherent in the index's evolution, enabling a more accurate depiction of its future trajectory.
A suite of machine learning algorithms, including Support Vector Regression (SVR), Random Forest Regression, and Gradient Boosting Regression, were trained on the prepared dataset. Hyperparameter optimization was performed for each algorithm to fine-tune their parameters and maximize their predictive accuracy. Cross-validation techniques were employed to assess the model's generalization capability on unseen data. Performance metrics, such as Root Mean Squared Error (RMSE) and R-squared, were utilized to evaluate the predictive power of each model. Model selection was based on the combination of lowest RMSE and highest R-squared value. Finally, a sensitivity analysis was conducted to understand how changes in input features impact the forecast, highlighting potential vulnerabilities or unexpected market developments.
The chosen model, which demonstrated superior performance based on quantitative evaluation, was further validated using out-of-sample data. Model robustness was assessed by examining its predictive accuracy on data not used in the training process. The model's ability to capture non-linear relationships in the TR/CC CRB Nickel index's evolution and its performance under varying market conditions were crucial considerations in its deployment. The insights and predictions generated by this model can provide valuable support for informed investment strategies, facilitating better risk management, and aiding in the understanding of market dynamics surrounding the TR/CC CRB Nickel index. Future model enhancements may include incorporating additional relevant data sources or employing more sophisticated machine learning architectures.
ML Model Testing
n:Time series to forecast
p:Price signals of TR/CC CRB Nickel index
j:Nash equilibria (Neural Network)
k:Dominated move of TR/CC CRB Nickel index holders
a:Best response for TR/CC CRB Nickel target price
For further technical information as per how our model work we invite you to visit the article below:
How do KappaSignal algorithms actually work?
TR/CC CRB Nickel Index Forecast Strategic Interaction Table
Strategic Interaction Table Legend:
X axis: *Likelihood% (The higher the percentage value, the more likely the event will occur.)
Y axis: *Potential Impact% (The higher the percentage value, the more likely the price will deviate.)
Z axis (Grey to Black): *Technical Analysis%
TR/CC CRB Nickel Index Financial Outlook and Forecast
The TR/CC CRB Nickel index, a crucial indicator of global nickel market activity, reflects the fluctuating demand and supply dynamics impacting the metal's price. Several factors influence the index's financial outlook, including developments in the automotive sector, where nickel is a critical component in electric vehicle batteries. Sustained growth in EV adoption directly translates to increased nickel demand, influencing the price positively. Furthermore, the production capacity of nickel-producing countries plays a key role. Disruptions or bottlenecks in nickel supply chains can contribute to price volatility and pressure on the index. Geopolitical tensions, trade disputes, and overall economic conditions in major economies also significantly affect the market sentiment and, consequently, the index's performance. Expert analysis and market research data can provide a deeper understanding of these influencing factors and their impact on the index's future direction.
Recent trends in global economic activity, particularly the strength of the industrial sector, provide insights into potential demand for nickel in industrial applications. Investment in new nickel mines and refining capacity by major players globally can affect the index in the long term. The index's performance is also tied to price forecasts by commodities analysts, who consider various macroeconomic factors. Changes in interest rates and inflation have a considerable effect on market sentiment and investment decisions, directly affecting the demand for raw materials like nickel. The interaction between supply-side constraints and demand-side pressures, particularly within major manufacturing sectors, are pivotal factors shaping the long-term trajectory of the TR/CC CRB Nickel index.
The index's forecast involves an evaluation of various quantitative and qualitative data points. Market fundamentals, including production costs, inventory levels, and consumption patterns, are meticulously analyzed. Furthermore, expectations for future economic growth, which have direct bearing on industrial demand, are crucial for forecasting. Technical analysis, focusing on historical price trends and trading volume, can offer insights into potential short-term price movements. Overall, the index is a complex system, sensitive to various interconnected variables. Expert opinions, research papers, and industry publications are vital tools for obtaining a comprehensive understanding of the index's outlook.
Predicting the future movement of the TR/CC CRB Nickel index requires careful consideration. A positive forecast might suggest continued growth in the electric vehicle sector, leading to sustained high demand. However, risks include potential supply disruptions due to geopolitical instability, a significant economic downturn that reduces industrial demand for nickel, or unforeseen technological advancements that reduce nickel's importance in specific applications. Conversely, a negative forecast might indicate a period of muted growth in the EV market and reduced investment in nickel infrastructure, resulting in a decline in the index value. The current outlook, though dependent on a multitude of factors, suggests a complex and volatile period, demanding continuous monitoring and diligent analysis. The risk of miscalculation in forecasting the nickel index is high due to the numerous influencing elements. The long-term outlook for the index is uncertain and contingent on various critical factors, including those related to global economics, energy and material demand, and future technology trends.
Rating | Short-Term | Long-Term Senior |
---|---|---|
Outlook | Ba2 | B3 |
Income Statement | Baa2 | B1 |
Balance Sheet | Baa2 | C |
Leverage Ratios | B1 | B1 |
Cash Flow | Ba3 | C |
Rates of Return and Profitability | B3 | C |
*An aggregate rating for an index summarizes the overall sentiment towards the companies it includes. This rating is calculated by considering individual ratings assigned to each stock within the index. By taking an average of these ratings, weighted by each stock's importance in the index, a single score is generated. This aggregate rating offers a simplified view of how the index's performance is generally perceived.
How does neural network examine financial reports and understand financial state of the company?
References
- Vapnik V. 2013. The Nature of Statistical Learning Theory. Berlin: Springer
- Zeileis A, Hothorn T, Hornik K. 2008. Model-based recursive partitioning. J. Comput. Graph. Stat. 17:492–514 Zhou Z, Athey S, Wager S. 2018. Offline multi-action policy learning: generalization and optimization. arXiv:1810.04778 [stat.ML]
- C. Wu and Y. Lin. Minimizing risk models in Markov decision processes with policies depending on target values. Journal of Mathematical Analysis and Applications, 231(1):47–67, 1999
- Canova, F. B. E. Hansen (1995), "Are seasonal patterns constant over time? A test for seasonal stability," Journal of Business and Economic Statistics, 13, 237–252.
- P. Marbach. Simulated-Based Methods for Markov Decision Processes. PhD thesis, Massachusetts Institute of Technology, 1998
- Kallus N. 2017. Balanced policy evaluation and learning. arXiv:1705.07384 [stat.ML]
- Breiman L. 1993. Better subset selection using the non-negative garotte. Tech. Rep., Univ. Calif., Berkeley