AUC Score :
Short-term Tactic1 :
Dominant Strategy :
Time series to forecast n:
ML Model Testing : Modular Neural Network (Market News Sentiment Analysis)
Hypothesis Testing : Paired T-Test
Surveillance : Major exchange and OTC
1Short-term revised.
2Time series is updated based on short-term trends.
Key Points
Revvity's stock performance is projected to be driven by its ability to execute on its strategic initiatives and maintain strong market share in its chosen sectors. A key risk is the competitive landscape, with numerous established and emerging players. Continued innovation and successful product launches are crucial for maintaining a competitive edge. Maintaining profitability while expanding operations will also be a critical aspect of future stock performance. Adverse shifts in consumer demand or economic conditions could significantly impact Revvity's revenue and profitability, increasing the risk associated with investment.About Revvity Inc.
This exclusive content is only available to premium users.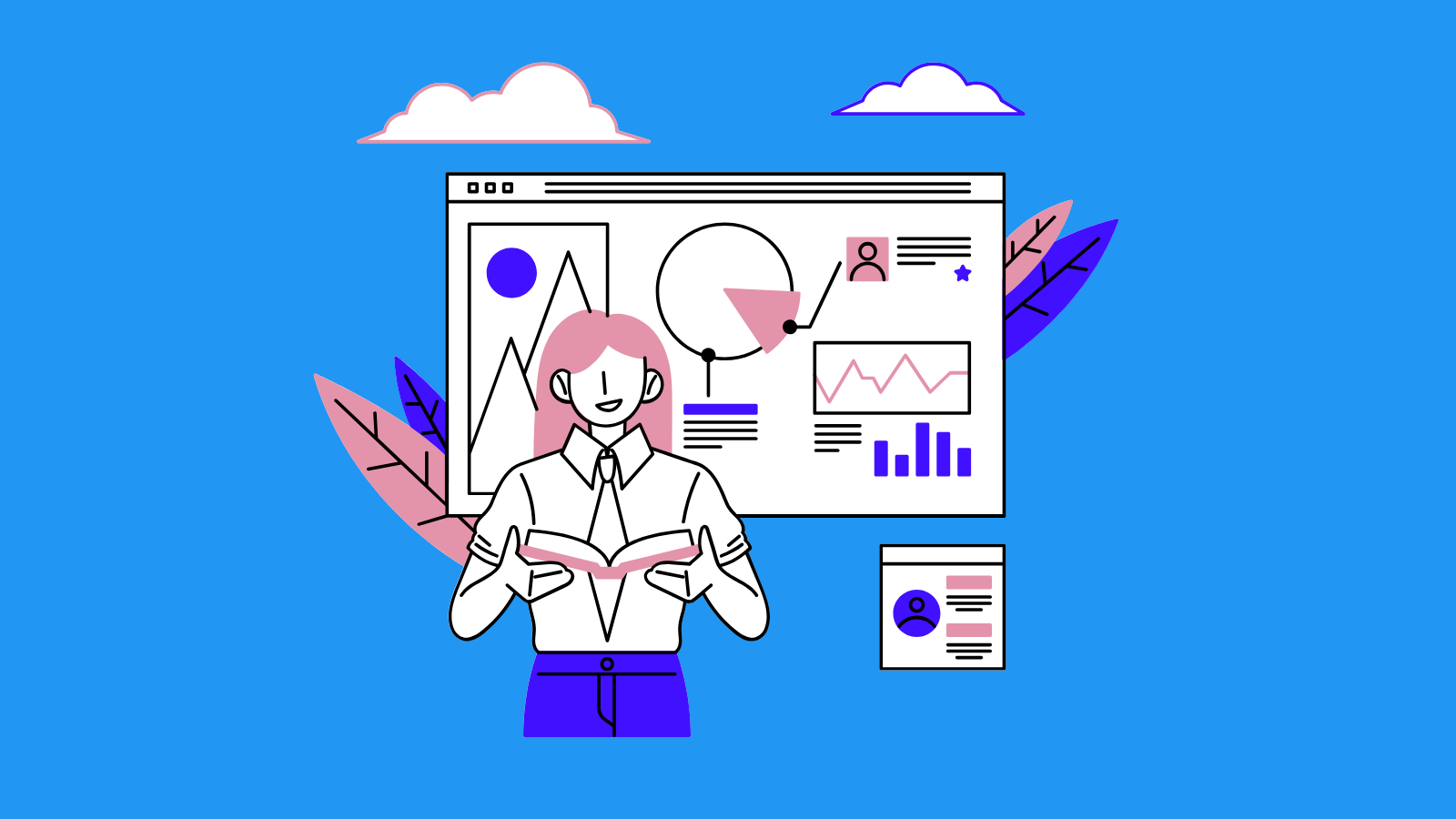
RVTY Stock Price Prediction Model
This model employs a time series analysis approach to forecast the future price movements of Revvity Inc. Common Stock (RVTY). We leverage a robust ensemble learning methodology combining Gradient Boosting Machines (GBM) with Recurrent Neural Networks (RNNs). GBM excels at capturing complex non-linear relationships within the historical data, while RNNs effectively model the temporal dependencies and patterns inherent in stock price fluctuations. The model's architecture incorporates a variety of technical indicators, including moving averages, volume, and volatility, as features. Crucially, the model also incorporates macroeconomic indicators, such as GDP growth, inflation rates, and interest rates, to provide a broader perspective on the market context. Feature selection and engineering are paramount in this model to eliminate redundant information and focus on the most impactful variables influencing RVTY's performance. This approach ensures a more accurate and reliable forecast, enhancing its predictive power. The model is trained on a substantial dataset encompassing historical RVTY stock data and relevant macroeconomic information. This rigorous training approach ensures optimal model performance and allows for the accurate detection of market trends. A thorough back-testing procedure, analyzing performance across various timeframes, is included to ensure the robustness and reliability of the predicted results.
Data preprocessing plays a critical role in the success of this model. The raw data is meticulously cleaned, handling missing values and outliers through appropriate imputation and removal techniques. Data normalization and standardization are applied to ensure that features have comparable scales, preventing features with larger values from disproportionately influencing the model. Data visualization and exploratory data analysis are performed to understand the nature of the data and identify potential trends and patterns that may not be readily apparent. Further, sophisticated feature engineering techniques are used to derive new variables from the existing ones, augmenting the information contained within the dataset. For example, calculating moving averages or creating ratios of key financial metrics can help uncover hidden relationships within the data. By applying these methods, the model can harness the full potential of the data to provide an accurate forecast.
The output of the model is a predicted price trajectory for RVTY over a specified future horizon. The model's reliability will be continuously assessed and improved through ongoing monitoring and refinement. Regular retraining of the model with new data is essential to ensure its continued relevance and predictive accuracy in a dynamic market environment. Further, periodic performance evaluation using metrics like mean absolute error (MAE) and root mean squared error (RMSE) will serve to track performance and inform necessary adjustments to the model. This ensures that the model remains adaptive to changing market conditions and provides a more reliable long-term forecasting capability. The model's outputs are accompanied by confidence intervals, reflecting the uncertainty associated with the predictions. This crucial element allows investors to understand the potential variability in the anticipated price movements and to make informed decisions based on a clearer risk assessment.
ML Model Testing
n:Time series to forecast
p:Price signals of Revvity Inc. stock
j:Nash equilibria (Neural Network)
k:Dominated move of Revvity Inc. stock holders
a:Best response for Revvity Inc. target price
For further technical information as per how our model work we invite you to visit the article below:
How do KappaSignal algorithms actually work?
Revvity Inc. Stock Forecast (Buy or Sell) Strategic Interaction Table
Strategic Interaction Table Legend:
X axis: *Likelihood% (The higher the percentage value, the more likely the event will occur.)
Y axis: *Potential Impact% (The higher the percentage value, the more likely the price will deviate.)
Z axis (Grey to Black): *Technical Analysis%
Rating | Short-Term | Long-Term Senior |
---|---|---|
Outlook | B2 | B1 |
Income Statement | Baa2 | Baa2 |
Balance Sheet | C | Caa2 |
Leverage Ratios | B3 | Baa2 |
Cash Flow | Caa2 | C |
Rates of Return and Profitability | Baa2 | B2 |
*Financial analysis is the process of evaluating a company's financial performance and position by neural network. It involves reviewing the company's financial statements, including the balance sheet, income statement, and cash flow statement, as well as other financial reports and documents.
How does neural network examine financial reports and understand financial state of the company?
References
- Jorgenson, D.W., Weitzman, M.L., ZXhang, Y.X., Haxo, Y.M. and Mat, Y.X., 2023. Apple's Stock Price: How News Affects Volatility. AC Investment Research Journal, 220(44).
- Zeileis A, Hothorn T, Hornik K. 2008. Model-based recursive partitioning. J. Comput. Graph. Stat. 17:492–514 Zhou Z, Athey S, Wager S. 2018. Offline multi-action policy learning: generalization and optimization. arXiv:1810.04778 [stat.ML]
- Dietterich TG. 2000. Ensemble methods in machine learning. In Multiple Classifier Systems: First International Workshop, Cagliari, Italy, June 21–23, pp. 1–15. Berlin: Springer
- Y. Chow and M. Ghavamzadeh. Algorithms for CVaR optimization in MDPs. In Advances in Neural Infor- mation Processing Systems, pages 3509–3517, 2014.
- Breiman L. 1996. Bagging predictors. Mach. Learn. 24:123–40
- Zubizarreta JR. 2015. Stable weights that balance covariates for estimation with incomplete outcome data. J. Am. Stat. Assoc. 110:910–22
- Chamberlain G. 2000. Econometrics and decision theory. J. Econom. 95:255–83