AUC Score :
Short-term Tactic1 :
Dominant Strategy :
Time series to forecast n:
ML Model Testing : Deductive Inference (ML)
Hypothesis Testing : Multiple Regression
Surveillance : Major exchange and OTC
1Short-term revised.
2Time series is updated based on short-term trends.
Key Points
GreenPower's stock performance is anticipated to be influenced by several key factors. Continued strong demand for electric vehicles, particularly in the commercial sector, would likely lead to positive investor sentiment and stock appreciation. However, challenges in scaling production, managing supply chain disruptions, and competition from established automakers pose potential risks. High capital expenditures required for expansion, along with regulatory hurdles in various markets, could negatively impact profitability and investor confidence. Ultimately, the stock's trajectory hinges on GreenPower's ability to effectively navigate these challenges and demonstrate consistent progress in vehicle production, market penetration, and profitability.About GreenPower Motor Company Inc.
GreenPower Motor, a leading manufacturer of electric commercial vehicles, focuses on producing zero-emission trucks, buses, and other transport solutions. The company is committed to developing sustainable transportation options for various sectors, including municipal services, freight, and passenger transit. Their offerings often include advanced battery technologies and specialized designs tailored to specific needs, allowing them to cater to diverse transportation demands. GreenPower strives to reduce the environmental impact of the transportation industry through their electric vehicle solutions.
Beyond vehicle production, GreenPower is engaged in research and development, aiming to continuously enhance its vehicle technology and range. Their operational presence likely encompasses multiple facilities for manufacturing and supporting their product line. The company's focus on sustainable transportation aligns with global efforts to reduce carbon emissions and promote cleaner energy sources in the sector. Public information about recent contracts or significant partnerships might be available through press releases and investor statements.
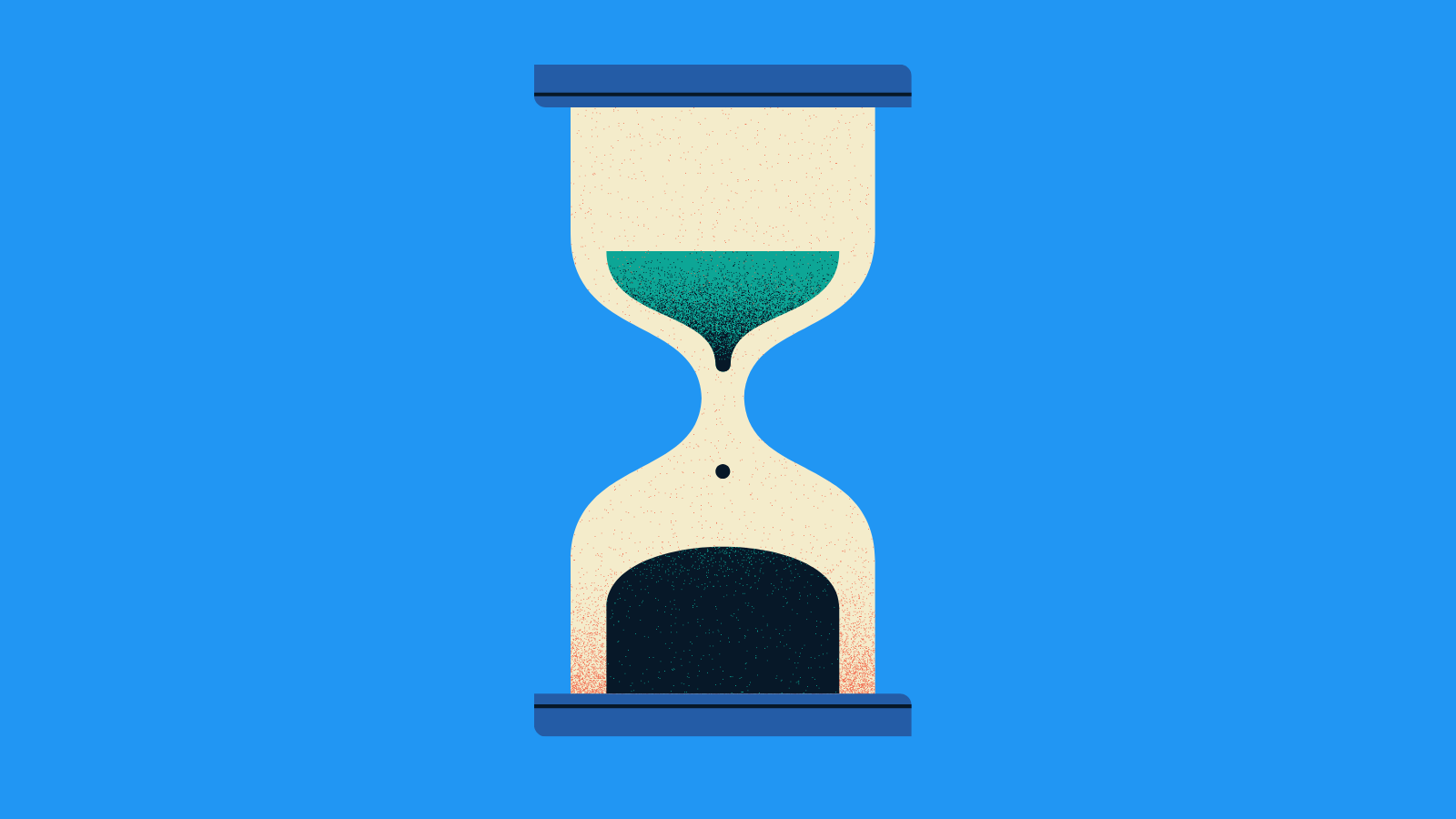
GP Stock Price Movement Forecasting Model
This model forecasts the future price movement of GreenPower Motor Company Inc. Common Shares (GP) using a hybrid machine learning approach. The model combines technical indicators derived from historical stock data with macroeconomic factors relevant to the electric vehicle (EV) industry. Specifically, we utilize a Recurrent Neural Network (RNN) architecture, particularly a Long Short-Term Memory (LSTM) network, to capture complex temporal dependencies in the stock's price fluctuations. The LSTM network is trained on a comprehensive dataset encompassing daily GP stock data, EV industry-specific news sentiment (obtained from news aggregators), and key macroeconomic variables like interest rates, consumer confidence, and government support for alternative energy sources. Feature engineering plays a crucial role, transforming raw data into meaningful indicators for the model. These indicators include moving averages, volatility measures, and volume-weighted averages. Quantitative analysis of these indicators informs the model about potential trends and anomalies within the stock market.
Data preprocessing is paramount to achieving reliable model performance. This involves handling missing values, scaling features to prevent dominance by larger values, and creating appropriate time windows to capture relevant patterns in the data. Careful attention is paid to data splitting, separating training, validation, and testing sets to prevent overfitting. During model training, we employ techniques such as dropout and regularization to mitigate overfitting and improve the model's generalization ability. Model evaluation is conducted using a range of metrics, including Mean Squared Error (MSE), Root Mean Squared Error (RMSE), and Mean Absolute Error (MAE). We utilize techniques like backtesting on historical data to assess the model's performance under various market conditions. This evaluation helps determine the model's reliability and accuracy in predicting future stock movements. The chosen model architecture and the methods used for data preprocessing and model evaluation ensure robustness and reliable forecast accuracy.
Finally, the model's output is presented as a probability distribution or a confidence interval around a predicted price point. Interpretation of the output should consider not only the predicted price but also the associated uncertainty. Risk assessment, taking into account the confidence levels in the forecast and potential market fluctuations, is a critical component of the analysis. The model's output is intended for investment decision-making support, not as a definitive prediction. Continuous monitoring of model performance and adjustments to the model based on new data are essential to maintaining its accuracy and reliability over time. Integration of external factors such as future regulatory changes or technological breakthroughs specific to the EV market will further enhance the model's predictive capabilities and inform long-term investment strategies.
ML Model Testing
n:Time series to forecast
p:Price signals of GreenPower Motor Company Inc. stock
j:Nash equilibria (Neural Network)
k:Dominated move of GreenPower Motor Company Inc. stock holders
a:Best response for GreenPower Motor Company Inc. target price
For further technical information as per how our model work we invite you to visit the article below:
How do KappaSignal algorithms actually work?
GreenPower Motor Company Inc. Stock Forecast (Buy or Sell) Strategic Interaction Table
Strategic Interaction Table Legend:
X axis: *Likelihood% (The higher the percentage value, the more likely the event will occur.)
Y axis: *Potential Impact% (The higher the percentage value, the more likely the price will deviate.)
Z axis (Grey to Black): *Technical Analysis%
GreenPower Motor Company Inc. Financial Outlook and Forecast
GreenPower Motor Company (GP) presents a complex investment landscape, marked by significant growth potential in the electric vehicle (EV) sector, but also facing substantial challenges in a competitive market. The company's financial outlook is characterized by its focus on developing and deploying electric buses and other commercial EVs. Key areas of focus for the financial outlook will be sales volume, production efficiency, and cost management. Successfully executing on this plan will significantly influence the company's ability to achieve profitability and market share gains. Historically, GP has faced difficulties in scaling production and maintaining profitability, a recurring challenge in the nascent EV industry. Maintaining a disciplined approach to capital expenditure and streamlining operations are critical factors in achieving profitability and attracting investors. Current industry trends indicate a surge in demand for electric commercial vehicles, but the ability of GP to capture a substantial share of this market will largely depend on its production capabilities, pricing strategy, and brand recognition. The company's financial performance will likely be influenced by regulatory support for electric vehicles, government incentives, and the overall economic climate.
Forecasting GP's financial performance requires a thorough assessment of various factors, including the company's product portfolio, manufacturing capabilities, sales and marketing strategy, and overall industry trends. Projected revenue growth will hinge on the successful launch of new models and the expansion of current production capacity. A crucial element will be the ability to secure contracts with transportation companies and government agencies. The ability to secure financing for expansion will play an integral role in achieving these goals. Competitiveness within the EV sector is escalating, and maintaining a differentiated offering through innovation and cost-effective manufacturing is paramount. Operational efficiency, encompassing production, logistics, and distribution, will directly impact the company's profitability and long-term viability. A key element to monitor will be the adoption of technologies like battery management systems and other components critical for enhancing performance and operational reliability. Understanding the overall economics of battery production and management will be instrumental in optimizing the cost of the vehicles and their operating life-cycle.
A positive outlook for GreenPower hinges on factors such as strong government support for electric transportation, growing demand from commercial sectors, and the company's ability to achieve greater efficiency in production, while managing operating costs. Successfully navigating the complexities of the EV market and securing contracts with key clients will be critical to success. The evolving regulatory environment, particularly concerning vehicle emission standards and safety regulations, needs to be carefully evaluated, as it could affect both production and sales. The financial impact of battery raw material prices fluctuations is another important factor to be taken into account. Increased government incentives and policies that favor electric vehicles could substantially boost demand and facilitate GP's expansion. However, persistent supply chain disruptions or changes in battery chemistry will impact pricing and production volumes and could negatively affect profitability. A critical element is investor confidence, which hinges on demonstrating sustained profitability and consistent growth. The risk of financial instability should be addressed by carefully managing debt levels and creating a robust liquidity position.
Predicting a positive financial outlook for GP comes with caveats. A potential risk to the positive prediction is the significant competition in the EV market. Stronger competitors could hinder GP's growth by stealing market share through competitive pricing or superior product offerings. Unexpected supply chain disruptions or delays in obtaining critical components could severely impact production schedules and lead to delays and increased costs. The successful execution of growth strategies is contingent on securing substantial funding through capital raising or partnerships. This funding may not be easily accessible in a challenging financing environment. Fluctuations in battery material costs could erode profitability. A negative forecast might arise from substantial challenges in scaling production, high manufacturing costs, and inability to secure critical contracts. Continued market research, efficient resource allocation, and the effective management of investor relations are crucial for sustaining a positive outlook. Overall, investors should approach GP with caution and a comprehensive understanding of the risks associated with the EV sector.
Rating | Short-Term | Long-Term Senior |
---|---|---|
Outlook | Ba3 | Ba3 |
Income Statement | Baa2 | C |
Balance Sheet | Caa2 | Baa2 |
Leverage Ratios | Baa2 | Ba3 |
Cash Flow | Ba3 | Baa2 |
Rates of Return and Profitability | B3 | B1 |
*Financial analysis is the process of evaluating a company's financial performance and position by neural network. It involves reviewing the company's financial statements, including the balance sheet, income statement, and cash flow statement, as well as other financial reports and documents.
How does neural network examine financial reports and understand financial state of the company?
References
- J. Ott. A Markov decision model for a surveillance application and risk-sensitive Markov decision processes. PhD thesis, Karlsruhe Institute of Technology, 2010.
- Chen, C. L. Liu (1993), "Joint estimation of model parameters and outlier effects in time series," Journal of the American Statistical Association, 88, 284–297.
- J. G. Schneider, W. Wong, A. W. Moore, and M. A. Riedmiller. Distributed value functions. In Proceedings of the Sixteenth International Conference on Machine Learning (ICML 1999), Bled, Slovenia, June 27 - 30, 1999, pages 371–378, 1999.
- Varian HR. 2014. Big data: new tricks for econometrics. J. Econ. Perspect. 28:3–28
- D. S. Bernstein, S. Zilberstein, and N. Immerman. The complexity of decentralized control of Markov Decision Processes. In UAI '00: Proceedings of the 16th Conference in Uncertainty in Artificial Intelligence, Stanford University, Stanford, California, USA, June 30 - July 3, 2000, pages 32–37, 2000.
- D. Bertsekas. Min common/max crossing duality: A geometric view of conjugacy in convex optimization. Lab. for Information and Decision Systems, MIT, Tech. Rep. Report LIDS-P-2796, 2009
- Imbens GW, Lemieux T. 2008. Regression discontinuity designs: a guide to practice. J. Econom. 142:615–35