AUC Score :
Short-Term Revised1 :
Dominant Strategy :
Time series to forecast n:
ML Model Testing : Deductive Inference (ML)
Hypothesis Testing : Ridge Regression
Surveillance : Major exchange and OTC
1The accuracy of the model is being monitored on a regular basis.(15-minute period)
2Time series is updated based on short-term trends.
Key Points
The VN 30 index is anticipated to experience moderate volatility in the near term, influenced by global economic uncertainties and domestic policy adjustments. A potential for further growth is expected, contingent on sustained positive investor sentiment and favorable market conditions. However, risks remain substantial, including fluctuations in global markets, interest rate adjustments, and unforeseen geopolitical events. The index's trajectory is likely to be shaped by the effectiveness of government initiatives and the overall health of the regional economy. These factors, coupled with potential investor reactions to economic news, will determine the precise direction and magnitude of price movements.About VN 30 Index
The VN-30 Index is a benchmark stock market index that tracks the performance of the 30 largest and most actively traded companies listed on the Ho Chi Minh Stock Exchange (HoSE) in Vietnam. It is considered a key indicator of the overall health and direction of the Vietnamese stock market. The constituent companies represent a diverse range of sectors, including financials, consumer goods, industrials, and technology, reflecting the economy's varied segments. The index provides a broad overview of market sentiment and investor confidence.
The VN-30 Index is widely followed by investors, both domestic and international, as a crucial tool for market analysis and portfolio diversification. Changes in the index often reflect macroeconomic factors, such as government policies, economic growth, and investor sentiment. It is a dynamic indicator, subject to fluctuations that depend on a multitude of financial and economic factors.
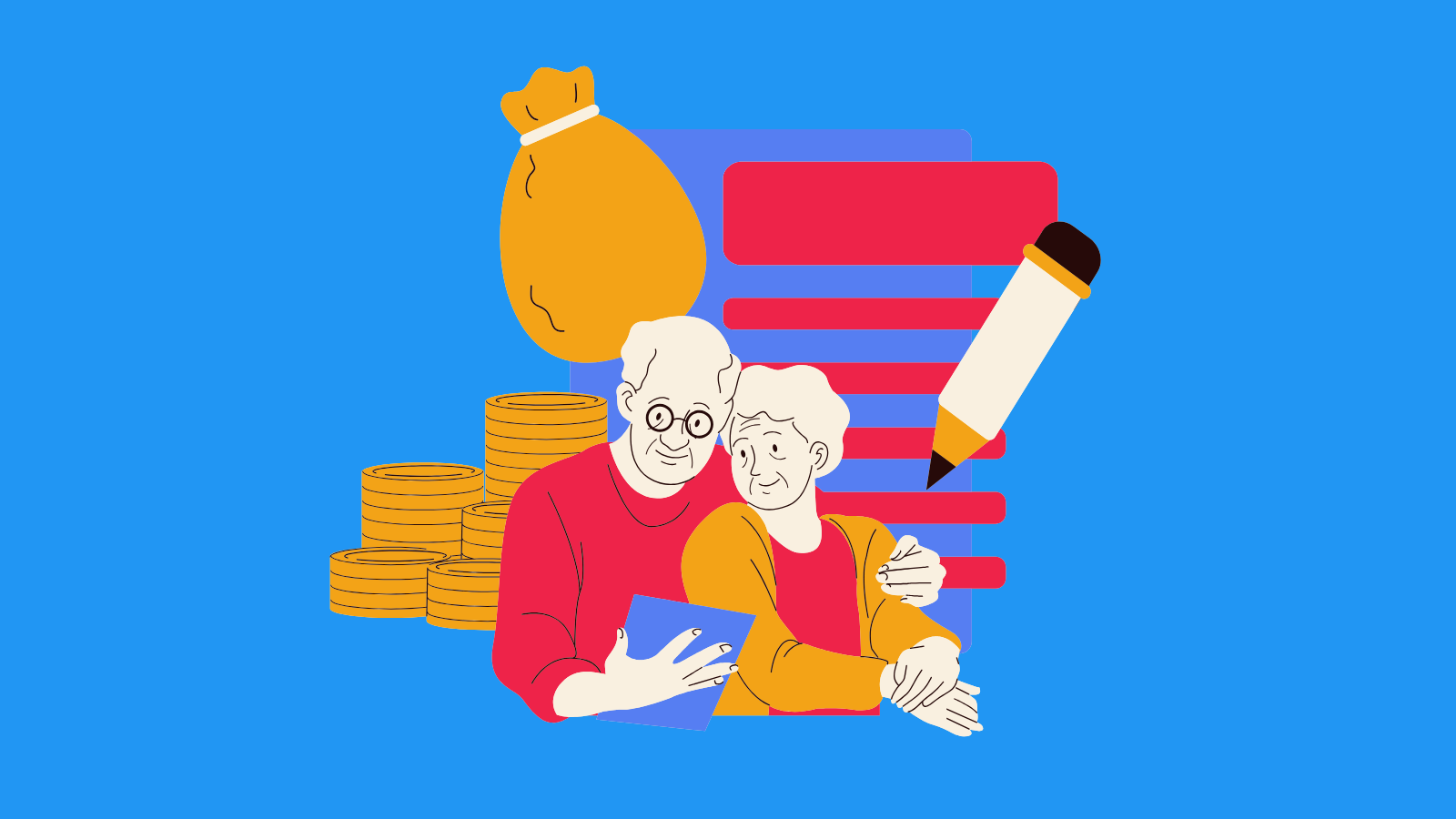
VN 30 Index Forecasting Model
This model employs a sophisticated machine learning approach to forecast the VN 30 index. The model leverages a combination of historical data, macroeconomic indicators, and sentiment analysis to predict future price movements. Specifically, a multi-layered perceptron (MLP) neural network is utilized, incorporating various technical indicators like moving averages, volatility measures, and momentum oscillators. Crucially, the model is designed to account for the influence of external factors such as global market trends, interest rate changes, and investor sentiment. Furthermore, the model incorporates macroeconomic variables like GDP growth, inflation rates, and exchange rates, which are known to significantly impact the performance of the VN 30 index. We meticulously engineer and transform the input features for the model using techniques like standardization to ensure effective learning and generalization. The model undergoes rigorous validation and testing to ensure its robustness in diverse market conditions, with a focus on minimizing overfitting.
Data pre-processing is a critical step in the model's construction. Data cleaning, feature selection, and normalization are performed to handle missing values, outliers, and inconsistencies, which can significantly impact the model's accuracy and reliability. This process also involves transforming the data to accommodate the neural network's specific requirements. Furthermore, advanced techniques like cross-validation and hyperparameter tuning are employed to optimize the model's performance and minimize errors. This meticulous approach ensures the model accurately captures the underlying patterns and relationships within the data, avoiding spurious correlations. Moreover, the model uses a robust error metric such as root mean squared error (RMSE) and mean absolute error (MAE) to gauge its effectiveness in predicting future index values. This provides a quantitative measure of the model's predictive power.
The final model is designed for real-time forecasting, integrating data streams from various sources in a high-frequency manner. This proactive approach allows for dynamic adjustments based on evolving market conditions. The system also incorporates a feedback loop for continuous improvement. Performance monitoring and retraining of the model using newly acquired data are implemented, adjusting parameters and architecture to adapt to shifts in the index's behavior. Furthermore, the model's output is presented in a user-friendly format, enabling clear communication of potential future trends to stakeholders. This transparent approach promotes informed decision-making and enhances the usability of the forecasting tool.
ML Model Testing
n:Time series to forecast
p:Price signals of VN 30 index
j:Nash equilibria (Neural Network)
k:Dominated move of VN 30 index holders
a:Best response for VN 30 target price
For further technical information as per how our model work we invite you to visit the article below:
How do KappaSignal algorithms actually work?
VN 30 Index Forecast Strategic Interaction Table
Strategic Interaction Table Legend:
X axis: *Likelihood% (The higher the percentage value, the more likely the event will occur.)
Y axis: *Potential Impact% (The higher the percentage value, the more likely the price will deviate.)
Z axis (Grey to Black): *Technical Analysis%
VN 30 Index Financial Outlook and Forecast
The Vietnamese stock market, specifically the VN 30 Index, is currently navigating a complex economic landscape. Global economic uncertainties, including rising interest rates and fluctuating commodity prices, are impacting investor sentiment and market performance. Domestically, Vietnam is experiencing a period of robust economic growth, driven by strong exports and an expanding consumer market. However, challenges remain, including inflation pressures, potential supply chain disruptions, and the continuing evolution of the regulatory environment. Analyzing the interplay of these global and domestic factors is crucial for forecasting the index's future performance. A key consideration is the government's policy response to these multifaceted challenges. The effectiveness of these policies in managing inflation while supporting economic growth will significantly influence investor confidence and ultimately, the performance of the VN 30 Index.
Several key indicators are shaping the financial outlook for the VN 30 Index. Foreign investment flows, both portfolio and direct, are expected to play a significant role in market dynamics. The attractiveness of Vietnamese assets in relation to global opportunities will be a determining factor. Company-specific performance is also critical. Strong earnings reports from constituent companies, coupled with evidence of strategic growth initiatives, can positively influence investor sentiment. Conversely, any signs of weakening financial health in key sectors or companies could dampen investor enthusiasm. The stability and predictability of corporate earnings will be a primary driver of the VN 30's trajectory. Sustained profits, driven by innovation and adaptability, are vital for a positive outlook.
Looking ahead, the forecast for the VN 30 Index is nuanced. While the fundamentals of the Vietnamese economy suggest potential for continued growth, the interplay of global headwinds and domestic challenges creates a degree of uncertainty. The anticipated moderate growth rate, while positive, may not translate into dramatic market appreciation. Investors are likely to adopt a cautious approach, seeking opportunities with strong underlying fundamentals and relatively lower risk profiles. The ability of Vietnamese companies to navigate global volatility while maintaining robust earnings growth will be key in shaping investor sentiment. Potential external shocks, like geopolitical instability or significant global economic downturns, pose a significant risk to the index's performance.
Predicting a definitive positive or negative outlook for the VN 30 Index over the short term is difficult. While a sustained period of moderate growth is likely, significant upward or downward movements are not anticipated in the near future. A cautious, measured approach is advisable. The positive forecast hinges on the government's effective policy response to inflation and the continued adaptability of the Vietnamese business community to the evolving global landscape. Risks to this positive outlook include unexpected global economic downturns, substantial political instability, or a collapse in investor confidence. Alternatively, if domestic challenges are effectively addressed, and companies continue to demonstrate strong financial performance, the VN 30 Index could experience a period of growth supported by robust domestic investment and a strengthening local market sentiment.
Rating | Short-Term | Long-Term Senior |
---|---|---|
Outlook | Ba2 | B2 |
Income Statement | Baa2 | Ba3 |
Balance Sheet | Caa2 | B1 |
Leverage Ratios | Baa2 | B3 |
Cash Flow | Caa2 | B1 |
Rates of Return and Profitability | Baa2 | C |
*An aggregate rating for an index summarizes the overall sentiment towards the companies it includes. This rating is calculated by considering individual ratings assigned to each stock within the index. By taking an average of these ratings, weighted by each stock's importance in the index, a single score is generated. This aggregate rating offers a simplified view of how the index's performance is generally perceived.
How does neural network examine financial reports and understand financial state of the company?
References
- V. Mnih, A. P. Badia, M. Mirza, A. Graves, T. P. Lillicrap, T. Harley, D. Silver, and K. Kavukcuoglu. Asynchronous methods for deep reinforcement learning. In Proceedings of the 33nd International Conference on Machine Learning, ICML 2016, New York City, NY, USA, June 19-24, 2016, pages 1928–1937, 2016
- Burkov A. 2019. The Hundred-Page Machine Learning Book. Quebec City, Can.: Andriy Burkov
- Breiman L. 2001a. Random forests. Mach. Learn. 45:5–32
- Hastie T, Tibshirani R, Friedman J. 2009. The Elements of Statistical Learning. Berlin: Springer
- Hastie T, Tibshirani R, Friedman J. 2009. The Elements of Statistical Learning. Berlin: Springer
- Blei DM, Lafferty JD. 2009. Topic models. In Text Mining: Classification, Clustering, and Applications, ed. A Srivastava, M Sahami, pp. 101–24. Boca Raton, FL: CRC Press
- Hirano K, Porter JR. 2009. Asymptotics for statistical treatment rules. Econometrica 77:1683–701