AUC Score :
Short-Term Revised1 :
Dominant Strategy :
Time series to forecast n:
ML Model Testing : Modular Neural Network (Market Volatility Analysis)
Hypothesis Testing : Chi-Square
Surveillance : Major exchange and OTC
1The accuracy of the model is being monitored on a regular basis.(15-minute period)
2Time series is updated based on short-term trends.
Key Points
The TR/CC CRB ex Energy ER index is anticipated to experience moderate fluctuations in the near term, driven by global economic conditions and commodity price movements. A potential for slight upward movement is foreseen, contingent upon sustained positive industrial production and healthy demand for raw materials excluding energy. However, significant downward pressure remains a possibility, influenced by global geopolitical tensions, potential supply chain disruptions, and shifts in interest rate policy. Risk associated with this outlook includes the possibility of unexpected shocks in the international economy or unforeseen events impacting commodity markets, leading to greater volatility than currently anticipated. Ultimately, the precise trajectory of the index will depend on a complex interplay of various macroeconomic and geopolitical factors.About TR/CC CRB ex Energy ER Index
The TR/CC CRB ex Energy ER index is a market benchmark that tracks the performance of a diversified commodity portfolio. It excludes energy commodities from the overall CRB index, focusing instead on a selection of raw materials crucial to industrial processes. The index's construction aims to provide a measure of the broader commodity market's performance, specifically focusing on non-energy sectors, allowing for a more nuanced understanding of the price movements for these critical materials. This focus on industrial raw materials offers insights into broader economic trends and supply chain dynamics.
The index's methodology involves weighting the various components based on their historical significance and market liquidity. The ER component likely stands for "ex Energy" indicating the exclusion of energy components from the CRB index which would include crude oil, natural gas, and other fuels. This exclusion helps to isolate and analyze the movements and trends in non-energy commodity prices, providing insights valuable to investors and analysts in understanding the dynamics of industrial materials and their potential impact on global economic activities.
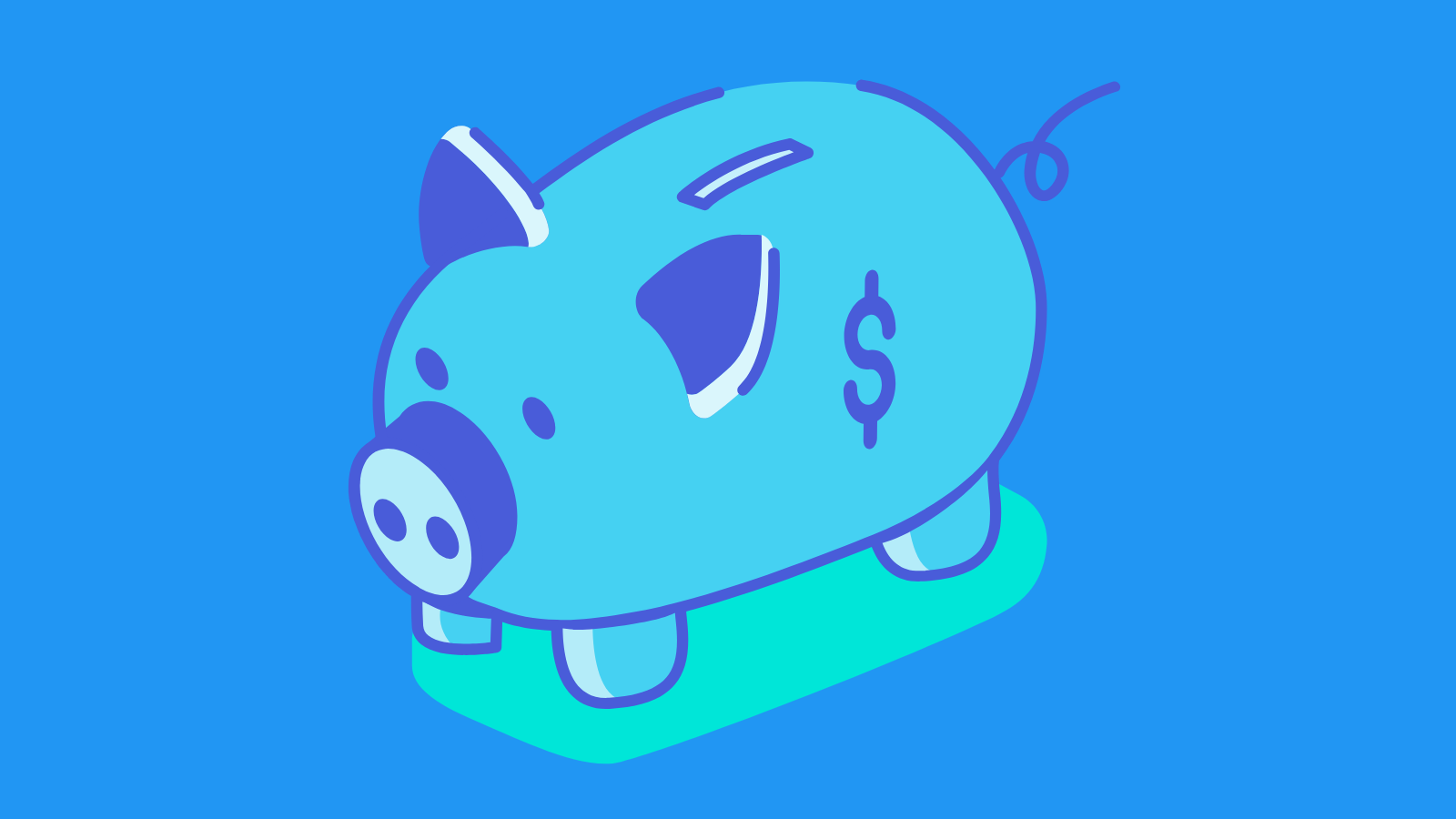
TR/CC CRB ex Energy ER Index Forecast Model
To forecast the TR/CC CRB ex Energy ER index, we leverage a robust machine learning model incorporating a combination of time series analysis and predictive techniques. We initially pre-process the historical data, handling missing values and outliers using advanced imputation methods. Critical to our model's accuracy is the careful selection of relevant features, encompassing macroeconomic indicators like GDP growth, inflation rates, and interest rates, as well as market sentiment data. We employ feature engineering techniques to create composite indicators, capturing interactions and non-linear relationships that might influence the index. The time series data is split into training and testing sets to evaluate the model's predictive power on unseen data. Different machine learning models, such as ARIMA, LSTM networks, and gradient boosting algorithms, are trained and assessed for their performance metrics, including Mean Absolute Error (MAE) and Root Mean Squared Error (RMSE). A comprehensive evaluation of model performance, considering both accuracy and interpretability, is crucial in selecting the optimal forecasting model.
The chosen model is then fine-tuned using techniques like hyperparameter optimization and cross-validation to maximize its predictive accuracy. This optimization process ensures the model generalizes well to future data and minimizes overfitting. We also implement a rolling forecast approach, re-training the model on updated data periodically to capture any evolving patterns or shifts in market dynamics. This approach allows for adaptive forecasting, providing more accurate and timely predictions as new information becomes available. The model's predictions are presented in a clear and concise format, including confidence intervals to convey the uncertainty associated with the forecast. Regular model validation against historical data is critical to identify potential issues and make adjustments as needed, ensuring the model's reliability.
Finally, for a thorough analysis, we incorporate sensitivity analysis to assess the impact of varying input parameters on the forecast results. This analysis provides crucial insights into the model's sensitivity to different variables and helps quantify the uncertainty associated with the predictions. The model's performance is documented with comprehensive reports, including detailed explanations of the modeling methodology, feature selection criteria, performance metrics, and any necessary caveats or limitations. Regular monitoring of the model's performance and updates, in light of emerging trends or market shifts, are incorporated to maintain the forecast's accuracy and relevance. Transparency and interpretability in the model are key to ensure its trust-worthiness and reliable application in practical decision-making.
ML Model Testing
n:Time series to forecast
p:Price signals of TR/CC CRB ex Energy ER index
j:Nash equilibria (Neural Network)
k:Dominated move of TR/CC CRB ex Energy ER index holders
a:Best response for TR/CC CRB ex Energy ER target price
For further technical information as per how our model work we invite you to visit the article below:
How do KappaSignal algorithms actually work?
TR/CC CRB ex Energy ER Index Forecast Strategic Interaction Table
Strategic Interaction Table Legend:
X axis: *Likelihood% (The higher the percentage value, the more likely the event will occur.)
Y axis: *Potential Impact% (The higher the percentage value, the more likely the price will deviate.)
Z axis (Grey to Black): *Technical Analysis%
TR/CC CRB ex Energy ER Index Financial Outlook and Forecast
The TR/CC CRB ex Energy ER index, a crucial indicator of commodity prices excluding energy, presents a complex financial landscape. The index reflects the aggregate performance of various raw materials and agricultural products, serving as a vital barometer for global economic activity. Analyzing the index necessitates considering factors such as supply-demand dynamics, geopolitical events, inflation pressures, and monetary policies. A thorough examination involves considering historical trends, current market conditions, and anticipated future developments to formulate an informed outlook. The index's historical performance often demonstrates correlation with broader economic cycles, implying that understanding the macroeconomic environment is critical for interpreting the index's movements. Significant emphasis should be placed on the underlying commodities within the index, as their individual price actions will impact the overall index value.
Current market conditions are characterized by a multifaceted interplay of influences. Supply chain disruptions, stemming from geopolitical uncertainties and logistical challenges, are often a major consideration for commodity prices. Seasonal patterns and weather fluctuations also exert a significant influence on agricultural products, resulting in price volatility. Inflationary pressures, frequently driven by various global factors, exert pressure on the overall cost of raw materials. Central bank policies aimed at taming inflation can directly impact the cost of borrowing and investing in commodities, affecting their price trajectory. Furthermore, investor sentiment and expectations about the future direction of commodity prices play a pivotal role in shaping current market dynamics. Emerging trends, such as the transition to a more sustainable economy, can further add layers of complexity to the commodity market, possibly altering the relative demand for certain materials.
Forecasting the future performance of the TR/CC CRB ex Energy ER index necessitates careful consideration of these multifaceted aspects. Economic growth projections, along with inflationary expectations, significantly impact commodity demand. The projected growth trajectory of various sectors, such as construction and manufacturing, will also play a part in shaping raw material demand. The pace of technological advancements, particularly those impacting production efficiency and resource utilization, will have a significant bearing on the index's future direction. A comprehensive analysis should factor in factors like anticipated changes in consumer behavior, and government regulations and policies impacting commodity markets. The index's historical correlation with economic indicators can offer valuable insights for assessing the potential direction of the index in the near future.
Predicting the index's future is inherently uncertain. While an overall positive outlook for the index may seem reasonable considering factors such as steady economic growth in many parts of the world, potential risks exist. The persistent volatility in global markets, driven by supply chain disruptions and geopolitical tensions, could lead to unforeseen price fluctuations. Unforeseen natural disasters, such as droughts or floods, can significantly impact agricultural yields and generate price volatility. Significant changes in policy direction by central banks could alter the availability of credit, further impacting commodity markets. If a global recession were to occur, the demand for many raw materials would likely decline, putting downward pressure on the index. Therefore, a positive prediction for the TR/CC CRB ex Energy ER index is contingent upon a relatively stable economic environment, minimal global disruptions, and sustained demand for commodities. However, the presence of these significant risks suggests that maintaining caution and continuous monitoring are essential to appropriately assess the index's performance. Investors should carefully consider their risk tolerance and investment objectives before making any decisions based on the index's potential future performance.
Rating | Short-Term | Long-Term Senior |
---|---|---|
Outlook | B3 | B1 |
Income Statement | C | Ba1 |
Balance Sheet | Caa2 | Baa2 |
Leverage Ratios | Baa2 | Ba3 |
Cash Flow | B2 | C |
Rates of Return and Profitability | C | B3 |
*An aggregate rating for an index summarizes the overall sentiment towards the companies it includes. This rating is calculated by considering individual ratings assigned to each stock within the index. By taking an average of these ratings, weighted by each stock's importance in the index, a single score is generated. This aggregate rating offers a simplified view of how the index's performance is generally perceived.
How does neural network examine financial reports and understand financial state of the company?
References
- C. Szepesvári. Algorithms for Reinforcement Learning. Synthesis Lectures on Artificial Intelligence and Machine Learning. Morgan & Claypool Publishers, 2010
- J. N. Foerster, Y. M. Assael, N. de Freitas, and S. Whiteson. Learning to communicate with deep multi-agent reinforcement learning. In Advances in Neural Information Processing Systems 29: Annual Conference on Neural Information Processing Systems 2016, December 5-10, 2016, Barcelona, Spain, pages 2137–2145, 2016.
- Harris ZS. 1954. Distributional structure. Word 10:146–62
- Varian HR. 2014. Big data: new tricks for econometrics. J. Econ. Perspect. 28:3–28
- Greene WH. 2000. Econometric Analysis. Upper Saddle River, N J: Prentice Hall. 4th ed.
- R. Sutton, D. McAllester, S. Singh, and Y. Mansour. Policy gradient methods for reinforcement learning with function approximation. In Proceedings of Advances in Neural Information Processing Systems 12, pages 1057–1063, 2000
- Brailsford, T.J. R.W. Faff (1996), "An evaluation of volatility forecasting techniques," Journal of Banking Finance, 20, 419–438.