AUC Score :
Short-Term Revised1 :
Dominant Strategy :
Time series to forecast n:
ML Model Testing : Ensemble Learning (ML)
Hypothesis Testing : Chi-Square
Surveillance : Major exchange and OTC
1The accuracy of the model is being monitored on a regular basis.(15-minute period)
2Time series is updated based on short-term trends.
Key Points
The TR/CC CRB ex Energy ER index is anticipated to exhibit a moderate upward trajectory, driven by strengthening global economic activity and increasing demand for commodities outside the energy sector. However, significant risks include unforeseen geopolitical events that could disrupt supply chains and lead to volatile price fluctuations. Further, inflationary pressures and potential interest rate hikes could negatively impact market sentiment and create headwinds for commodity prices. While a positive outlook is present, the index's performance is likely to be influenced by a complex interplay of macroeconomic factors, making precise predictions challenging.About TR/CC CRB ex Energy ER Index
The TR/CC CRB ex Energy ER index is a market-based benchmark that tracks the performance of a portfolio of commodities. It specifically excludes energy components, focusing on the remaining raw materials. The index is designed to provide a measure of price fluctuations and investment returns within the non-energy commodity sector. This allows for a focused evaluation of the performance of a range of raw materials. The precise constituents of the index and its calculation methodology are typically proprietary and publicly available information may be limited.
The index's design aims to offer investors and analysts a more refined perspective on the performance of commodities outside the volatile energy sector. This allows for a clearer picture of the broader commodity market trends compared to benchmarks which encompass energy. By excluding energy, it isolates and highlights the trends in the remaining commodities and allows for specific investment decisions based on this sector of the broader market. This focused view can be beneficial in different market contexts.
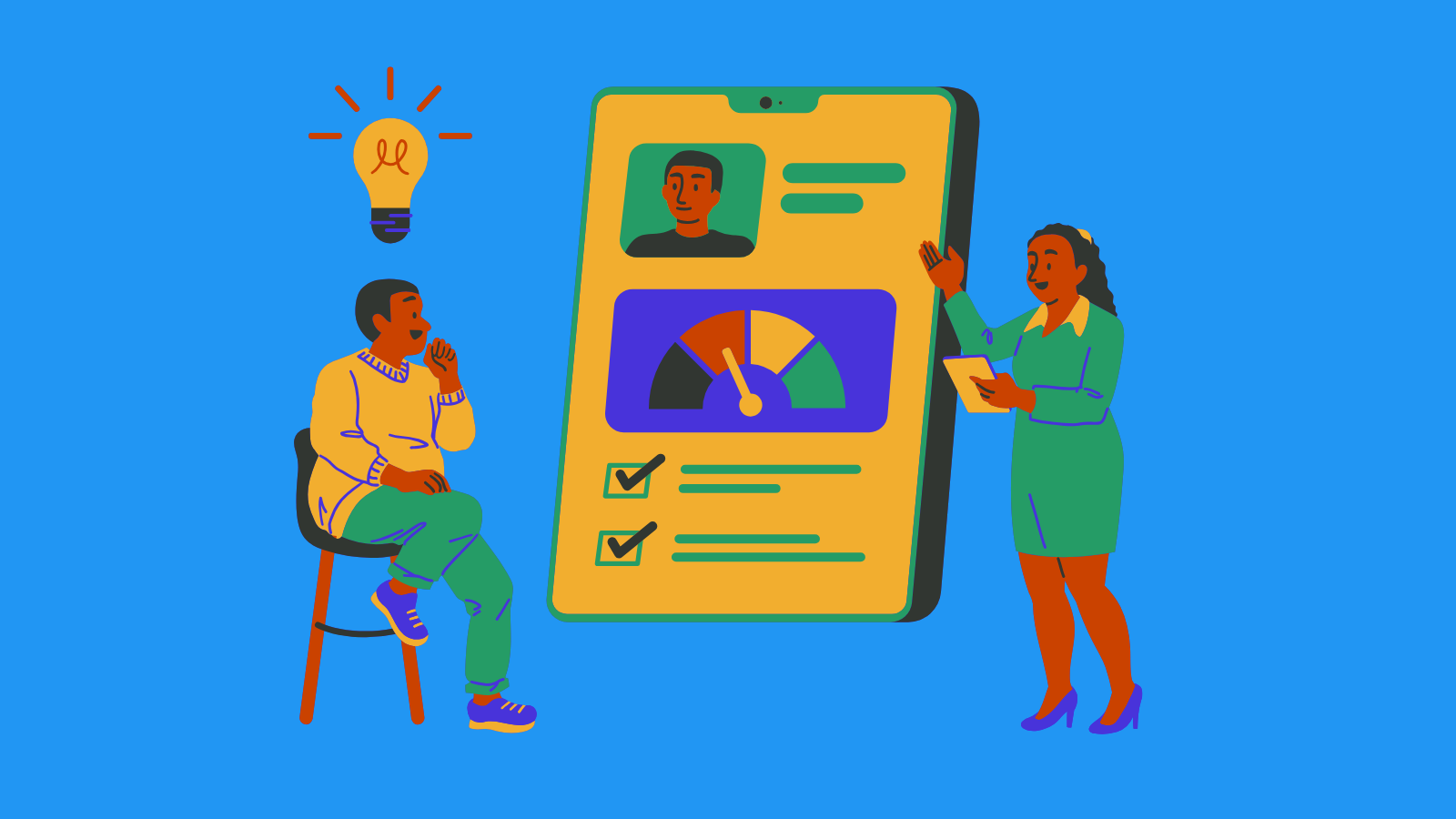
TR/CC CRB ex Energy ER Index Forecast Model
A machine learning model for forecasting the TR/CC CRB ex Energy ER index requires a comprehensive approach incorporating historical data, economic indicators, and potential market drivers. The model should leverage a diverse dataset including past TR/CC CRB ex Energy ER index values, alongside relevant economic indicators such as GDP growth, inflation rates, interest rates, and commodity prices (excluding energy). Feature engineering plays a crucial role, potentially transforming raw data into more informative variables. For example, lagged values of the index and economic indicators can capture temporal dependencies. Crucially, this model must consider potential seasonality and cyclical patterns within the data, which can significantly influence forecasting accuracy. Employing a time series model, such as an ARIMA or a more advanced model like a Prophet, would be appropriate to capture these patterns and potentially improve the predictive power of the model. Rigorous model validation against historical data is critical to ensure the model's robustness and to avoid overfitting. Performance metrics, like Mean Absolute Error (MAE) and Root Mean Squared Error (RMSE), will be employed to evaluate the model's efficacy.
Data preprocessing steps, like handling missing values and outliers, are paramount to ensuring data quality and reliability. Feature selection techniques, such as correlation analysis and recursive feature elimination, can help in identifying the most relevant predictors for the model. A critical aspect is to analyze the impact of external factors, which could include geopolitical events, trade policies, and changes in supply chains. Explicitly incorporating these external factors via feature engineering would further enhance the model's explanatory and predictive capabilities. The model should be capable of handling potential shifts in the data patterns over time, known as regime shifts. To this end, using a model that can dynamically adjust to changing conditions, such as a machine learning model with adaptable parameters or a switching regression model, will increase the model's generalizability across different economic scenarios. This is essential for maintaining the model's effectiveness in the long run.
Model deployment and monitoring are equally crucial components of this project. Once the model is developed and validated, it should be deployed in a robust and efficient manner to generate timely forecasts. A crucial component of the model's lifespan is monitoring its performance over time. This includes continuously tracking the model's accuracy and adjusting it based on new data and changing market conditions. Regular evaluation against new data will help maintain the model's predictive accuracy and address any drift in its performance over time. Implementing a system to automatically retrain the model on a scheduled basis will ensure the model continues to be updated. This approach will yield a predictive model that is not just informative but also suitable for integration into larger economic forecasting frameworks, offering actionable insights for businesses and policymakers.
ML Model Testing
n:Time series to forecast
p:Price signals of TR/CC CRB ex Energy ER index
j:Nash equilibria (Neural Network)
k:Dominated move of TR/CC CRB ex Energy ER index holders
a:Best response for TR/CC CRB ex Energy ER target price
For further technical information as per how our model work we invite you to visit the article below:
How do KappaSignal algorithms actually work?
TR/CC CRB ex Energy ER Index Forecast Strategic Interaction Table
Strategic Interaction Table Legend:
X axis: *Likelihood% (The higher the percentage value, the more likely the event will occur.)
Y axis: *Potential Impact% (The higher the percentage value, the more likely the price will deviate.)
Z axis (Grey to Black): *Technical Analysis%
TR/CC CRB ex Energy ER Index Financial Outlook and Forecast
The TR/CC CRB ex Energy ER index, a benchmark tracking commodity prices excluding energy, presents a complex financial outlook. This index gauges the performance of a diverse portfolio of raw materials, excluding volatile energy prices, offering investors a view into the broader commodity market. A fundamental understanding of the market dynamics surrounding these raw materials is crucial to evaluating potential investment opportunities. Factors such as global economic growth, supply chain disruptions, geopolitical tensions, and inflationary pressures directly influence the index's performance. Current global economic conditions, including the ongoing war in Ukraine and the lingering effects of the pandemic, play a significant role in shaping the demand and supply dynamics for various commodities. Historically, commodity prices have exhibited cyclical trends, and current economic forecasts suggest a potential for continued volatility in the market. A thorough analysis of supply and demand fundamentals, combined with geopolitical considerations, is necessary to make accurate predictions about the index's future direction.
Several macro-economic indicators point to both challenges and potential opportunities for the index. Increasing global interest rates and concerns about potential recessionary pressures can exert downward pressure on commodity prices. Reduced industrial activity and consumer spending are likely to moderate demand for certain raw materials. On the other hand, persistent inflationary pressures and ongoing geopolitical events could create a fluctuating environment, leading to heightened volatility in commodity prices. Supply chain disruptions and limitations, particularly regarding raw material access in certain regions, can impact the availability and pricing of key commodities, thus influencing the TR/CC CRB ex Energy ER index. A deeper understanding of these interconnected elements is essential in formulating accurate investment strategies. Moreover, the fluctuating currency markets and exchange rates can also significantly affect the prices of internationally traded commodities. Therefore, a thorough examination of these factors is necessary for a comprehensive market analysis.
Forecasting the precise trajectory of the TR/CC CRB ex Energy ER index requires careful consideration of multiple variables. The extent to which various economies experience growth or recession, alongside the response of governments to inflationary pressures, are key determinants. Political stability in key commodity-producing regions and ongoing trade negotiations globally can all significantly influence the availability and pricing of raw materials. The long-term outlook of the index relies heavily on sustainable infrastructure projects, environmental regulations, and the development of new technologies. These factors can directly impact production costs and resource allocation. Changes in consumer preferences and evolving technological trends will also play a crucial role in shaping the demand for specific raw materials, and hence their market values.
The prediction for the TR/CC CRB ex Energy ER index is likely to exhibit moderate fluctuations over the next 12-18 months. A potential positive outlook hinges on a sustained period of global economic expansion, accompanied by a relative stability in geopolitical landscapes. This would drive demand for the commodities in the index, resulting in price appreciation. However, a period of economic contraction or heightened geopolitical instability would significantly dampen the index's performance. Risks to this prediction include a prolonged period of economic uncertainty, supply chain disruptions, and unforeseen geopolitical events. The anticipated volatility necessitates careful monitoring of market trends and macroeconomic indicators before making investment decisions. Diversification across various asset classes and industries within the commodity sector is strongly advised, as it minimizes the impact of any unforeseen challenges that might affect the index's performance. It is crucial to adopt a cautious approach and conduct thorough research before entering or exiting any commodity investment.
Rating | Short-Term | Long-Term Senior |
---|---|---|
Outlook | B1 | Ba1 |
Income Statement | Baa2 | Caa2 |
Balance Sheet | Baa2 | Baa2 |
Leverage Ratios | B2 | B1 |
Cash Flow | Caa2 | Baa2 |
Rates of Return and Profitability | Caa2 | Baa2 |
*An aggregate rating for an index summarizes the overall sentiment towards the companies it includes. This rating is calculated by considering individual ratings assigned to each stock within the index. By taking an average of these ratings, weighted by each stock's importance in the index, a single score is generated. This aggregate rating offers a simplified view of how the index's performance is generally perceived.
How does neural network examine financial reports and understand financial state of the company?
References
- Breiman L. 2001a. Random forests. Mach. Learn. 45:5–32
- Ashley, R. (1988), "On the relative worth of recent macroeconomic forecasts," International Journal of Forecasting, 4, 363–376.
- Nie X, Wager S. 2019. Quasi-oracle estimation of heterogeneous treatment effects. arXiv:1712.04912 [stat.ML]
- M. Ono, M. Pavone, Y. Kuwata, and J. Balaram. Chance-constrained dynamic programming with application to risk-aware robotic space exploration. Autonomous Robots, 39(4):555–571, 2015
- Hastie T, Tibshirani R, Wainwright M. 2015. Statistical Learning with Sparsity: The Lasso and Generalizations. New York: CRC Press
- N. B ̈auerle and J. Ott. Markov decision processes with average-value-at-risk criteria. Mathematical Methods of Operations Research, 74(3):361–379, 2011
- A. Tamar, D. Di Castro, and S. Mannor. Policy gradients with variance related risk criteria. In Proceedings of the Twenty-Ninth International Conference on Machine Learning, pages 387–396, 2012.