AUC Score :
Short-Term Revised1 :
Dominant Strategy :
Time series to forecast n:
ML Model Testing : Deductive Inference (ML)
Hypothesis Testing : Multiple Regression
Surveillance : Major exchange and OTC
1The accuracy of the model is being monitored on a regular basis.(15-minute period)
2Time series is updated based on short-term trends.
Key Points
Teradyne's future performance hinges on several key factors. Continued success in the semiconductor testing market, particularly with advancements in AI and automation, is crucial. Sustained demand for advanced test equipment, driven by the burgeoning demand for integrated circuits and consumer electronics, is a critical success factor. However, global economic headwinds and geopolitical uncertainty could negatively impact demand for high-tech products, posing a significant risk. Further, competition from both established and emerging players in the semiconductor testing sector will likely intensify, requiring Teradyne to maintain its technological edge and adapt to changing market dynamics. Successfully navigating these challenges will be vital for Teradyne's long-term growth trajectory and profitability. The company's ability to innovate and execute strategically will dictate its success.About Teradyne
Teradyne is a leading provider of automated test equipment and software solutions for the semiconductor, electronics, and other high-tech industries. The company designs, manufactures, and sells a wide range of advanced test systems used in the entire product lifecycle, from design validation to production testing. Teradyne's expertise in high-speed testing and complex integrated circuit (IC) validation is well-regarded in the industry. Their commitment to innovation and continuous development of testing methodologies positions them as a key player in driving technological advancement. The company's customer base spans a broad range of major companies in the electronics sector, demonstrating a strong market presence and influence.
Teradyne's product portfolio is typically categorized by the applications they serve. From advanced semiconductor testing to systems verification, they provide comprehensive solutions to meet the specific requirements of various industry segments. Their global reach and extensive customer support networks ensure effective integration and maintenance of the sophisticated systems they deploy. Key differentiators include a strong focus on customization to meet specific customer needs, as well as a dedication to ongoing product improvements and technological advancements.
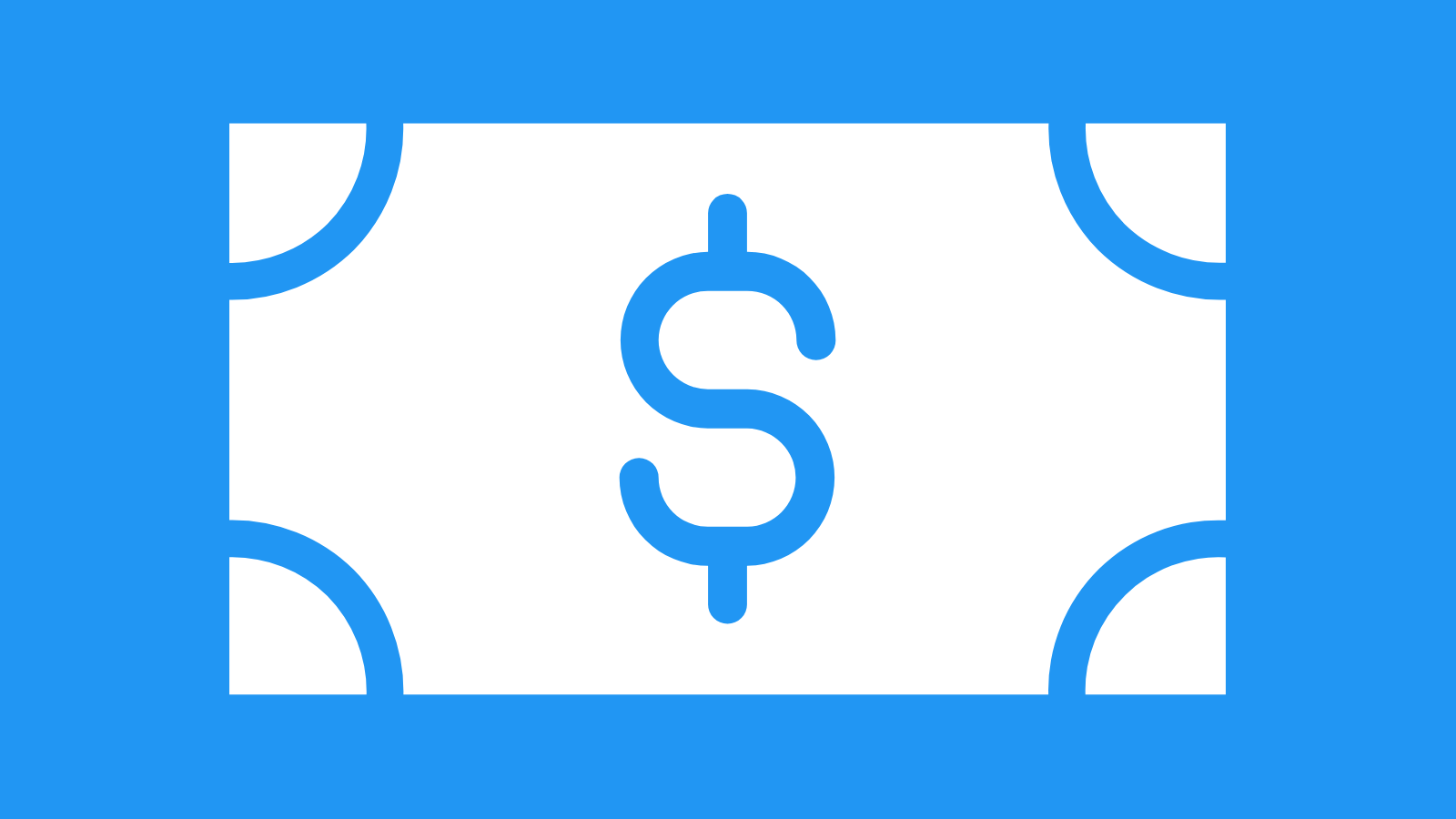
TERadyne Inc. Common Stock Forecasting Model
This model utilizes a machine learning approach to forecast the future performance of Teradyne Inc. (TER) common stock. The model leverages a comprehensive dataset encompassing historical stock performance data, macroeconomic indicators, industry trends, and company-specific financial metrics. Key variables considered in the model include Teradyne's quarterly earnings reports, revenue growth, capital expenditure, and the company's competitive landscape within the semiconductor testing sector. Fundamental analysis, analyzing the company's financial statements to assess its profitability and solvency, is integrated into the model's feature engineering process. A robust time series analysis is conducted to capture historical patterns and cyclical fluctuations within the stock's price action. Model architecture comprises a stacked LSTM (Long Short-Term Memory) network augmented with technical indicators such as moving averages and relative strength index. This architecture is chosen for its ability to capture intricate temporal dependencies and predict potential market turning points. The model is rigorously evaluated using a rolling forecasting strategy to ensure its predictive validity over different timeframes. The goal is to provide insightful predictions and assess future risk.
The data preprocessing phase is crucial for effective model training. Data cleaning and normalization procedures are implemented to mitigate the influence of outliers and ensure consistent scaling across different variables. Feature engineering is employed to transform raw data into valuable features for the model, including calculating ratios and creating new indicators to capture the dynamics of the stock market and the semiconductor industry. An extensive feature selection process is conducted to identify the most impactful predictors for Teradyne stock's future performance. Model validation encompasses the use of various metrics, including root mean squared error (RMSE) and mean absolute error (MAE), to quantify the predictive accuracy of the model. The model is retrained periodically with updated data to maintain its effectiveness, and the predictive accuracy is continuously monitored to assess its adaptability to changes in market conditions. The model outputs probabilities of the stock price exceeding specific thresholds within a given timeframe, supporting decision-making by investors.
Model deployment involves creating a user-friendly interface for investors and financial analysts. The interface will display the model's predictions along with a comprehensive set of insights and explanations. The platform will also present the limitations of the model, emphasizing the inherent uncertainties in stock market forecasting and the importance of considering other factors beyond the model's scope. Risk assessment is integrated into the model's output to help investors gauge the potential downsides associated with different investment decisions. The output will incorporate uncertainty measures, such as confidence intervals, to contextualize the predictions and provide more informed insights to users. Regular model performance reviews and updates will be implemented to continuously optimize the model's accuracy and predictive capabilities over time. Ultimately, this framework aims to provide data-driven, informed decision-making support for Teradyne stakeholders.
ML Model Testing
n:Time series to forecast
p:Price signals of Teradyne stock
j:Nash equilibria (Neural Network)
k:Dominated move of Teradyne stock holders
a:Best response for Teradyne target price
For further technical information as per how our model work we invite you to visit the article below:
How do KappaSignal algorithms actually work?
Teradyne Stock Forecast (Buy or Sell) Strategic Interaction Table
Strategic Interaction Table Legend:
X axis: *Likelihood% (The higher the percentage value, the more likely the event will occur.)
Y axis: *Potential Impact% (The higher the percentage value, the more likely the price will deviate.)
Z axis (Grey to Black): *Technical Analysis%
Teradyne Financial Outlook and Forecast
Teradyne's financial outlook for the foreseeable future is characterized by a complex interplay of factors, predominantly driven by the company's position within the semiconductor and electronics manufacturing testing equipment sector. Significant investments in research and development, coupled with a focus on expanding into emerging markets, suggest a potential for growth, particularly in the face of increasing demand for advanced testing solutions. Teradyne's historical performance demonstrates a capacity for robust profitability, often exhibiting strong revenue streams in times of industry growth. Key indicators like backlog, order book size, and the successful implementation of new product lines are crucial determinants for the near-term and long-term financial trajectory. The success of these initiatives hinges heavily on the trajectory of the semiconductor industry, including the ability of global chip manufacturers to effectively manage their production demands. Further, Teradyne's product offering often extends beyond basic testing into more sophisticated, specialized solutions, which positions them well to capitalize on the continuous evolution of electronics manufacturing requirements.
A critical aspect to consider is the competitive landscape. Teradyne operates in a highly competitive market, facing competition from established players and emerging companies specializing in specialized testing equipment. Maintaining market share and adapting to rapidly evolving technologies will be pivotal. The company's strategic partnerships, and their capacity to adapt to evolving technology trends (e.g. AI, automation), are likely crucial elements in their continuing competitiveness. Cost management and operational efficiency are key to maintaining profitability within this environment. The global economic climate, including fluctuating demand, supply chain disruptions, and geopolitical factors, could significantly affect Teradyne's financial performance. Effective risk management and adaptability will be vital in navigating these potential challenges. Furthermore, customer relationships and their willingness to invest in sophisticated testing equipment will significantly impact the company's success.
Revenue forecasts for Teradyne often reflect anticipated industry growth and market trends. Historically, the company has demonstrated resilience during economic downturns, suggesting a degree of financial stability. However, the cyclical nature of the electronics market and any significant shifts in demand or technological paradigms could affect forecasts. The ability to secure new contracts, especially large ones, is essential for maintaining a consistent financial performance. Analysts typically assess the company's financial stability by evaluating factors such as debt levels, profitability metrics (like gross margin and operating income), and return on invested capital. Careful analysis of these key performance indicators provides insight into the overall financial health and potential for future growth. The ongoing shift towards more sophisticated electronic systems and the increasing necessity for high-quality testing will likely propel demand for Teradyne's specialized equipment.
Predicting the future financial performance of Teradyne involves evaluating both positive and negative factors. A positive prediction suggests continued strong performance driven by expanding market demand for advanced testing solutions, successful product introductions, and a robust order book. Maintaining operational efficiency, mitigating supply chain risks, and responding to technological changes would be key to sustaining this positive trajectory. However, potential risks include slower-than-anticipated growth in the semiconductor industry, increased competition, unforeseen supply chain disruptions, and economic downturns. A negative prediction would result from a combination of these risks, impacting order intake, resulting in lower profits and diminished market share. The ultimate financial success of Teradyne hinges on its ability to navigate these uncertainties and leverage opportunities in a dynamic and complex market.
Rating | Short-Term | Long-Term Senior |
---|---|---|
Outlook | B3 | B2 |
Income Statement | C | C |
Balance Sheet | Caa2 | Ba3 |
Leverage Ratios | C | C |
Cash Flow | Ba3 | Ba3 |
Rates of Return and Profitability | B3 | B3 |
*Financial analysis is the process of evaluating a company's financial performance and position by neural network. It involves reviewing the company's financial statements, including the balance sheet, income statement, and cash flow statement, as well as other financial reports and documents.
How does neural network examine financial reports and understand financial state of the company?
References
- Zou H, Hastie T. 2005. Regularization and variable selection via the elastic net. J. R. Stat. Soc. B 67:301–20
- J. N. Foerster, Y. M. Assael, N. de Freitas, and S. Whiteson. Learning to communicate with deep multi-agent reinforcement learning. In Advances in Neural Information Processing Systems 29: Annual Conference on Neural Information Processing Systems 2016, December 5-10, 2016, Barcelona, Spain, pages 2137–2145, 2016.
- Mullainathan S, Spiess J. 2017. Machine learning: an applied econometric approach. J. Econ. Perspect. 31:87–106
- R. Sutton, D. McAllester, S. Singh, and Y. Mansour. Policy gradient methods for reinforcement learning with function approximation. In Proceedings of Advances in Neural Information Processing Systems 12, pages 1057–1063, 2000
- Schapire RE, Freund Y. 2012. Boosting: Foundations and Algorithms. Cambridge, MA: MIT Press
- Armstrong, J. S. M. C. Grohman (1972), "A comparative study of methods for long-range market forecasting," Management Science, 19, 211–221.
- M. Babes, E. M. de Cote, and M. L. Littman. Social reward shaping in the prisoner's dilemma. In 7th International Joint Conference on Autonomous Agents and Multiagent Systems (AAMAS 2008), Estoril, Portugal, May 12-16, 2008, Volume 3, pages 1389–1392, 2008.