AUC Score :
Short-Term Revised1 :
Dominant Strategy :
Time series to forecast n:
ML Model Testing : Ensemble Learning (ML)
Hypothesis Testing : Sign Test
Surveillance : Major exchange and OTC
1The accuracy of the model is being monitored on a regular basis.(15-minute period)
2Time series is updated based on short-term trends.
Key Points
Schrodinger's stock performance is predicted to be influenced significantly by the trajectory of its research and development efforts, specifically the commercialization of its AI-driven drug discovery platform. Success in securing partnerships or demonstrating tangible progress in clinical trials will likely drive investor confidence and potentially lead to a price increase. Conversely, setbacks in these areas could result in investor skepticism and a decline in share value. Regulatory hurdles and competition from established pharmaceutical companies represent substantial risks. Failure to achieve meaningful milestones or adapt to evolving market demands could severely impact Schrodinger's stock valuation.About Schrodinger
Schrodinger, Inc. is a leading provider of advanced computational chemistry solutions. The company focuses on accelerating the discovery and design of novel materials, pharmaceuticals, and other products. Their core technology leverages quantum chemistry and machine learning algorithms to model molecular interactions and predict material properties. Schrodinger's software and services are utilized by pharmaceutical companies, materials scientists, and academic researchers worldwide, enabling them to streamline their research processes and enhance productivity.
Schrodinger's offerings encompass a range of tools, from basic molecular modeling software to sophisticated simulation platforms. The company actively invests in research and development to continually enhance its capabilities and expand its application areas. Their commitment to innovation and cutting-edge computational techniques positions them as a key player in the rapidly evolving field of computational materials science and drug discovery.
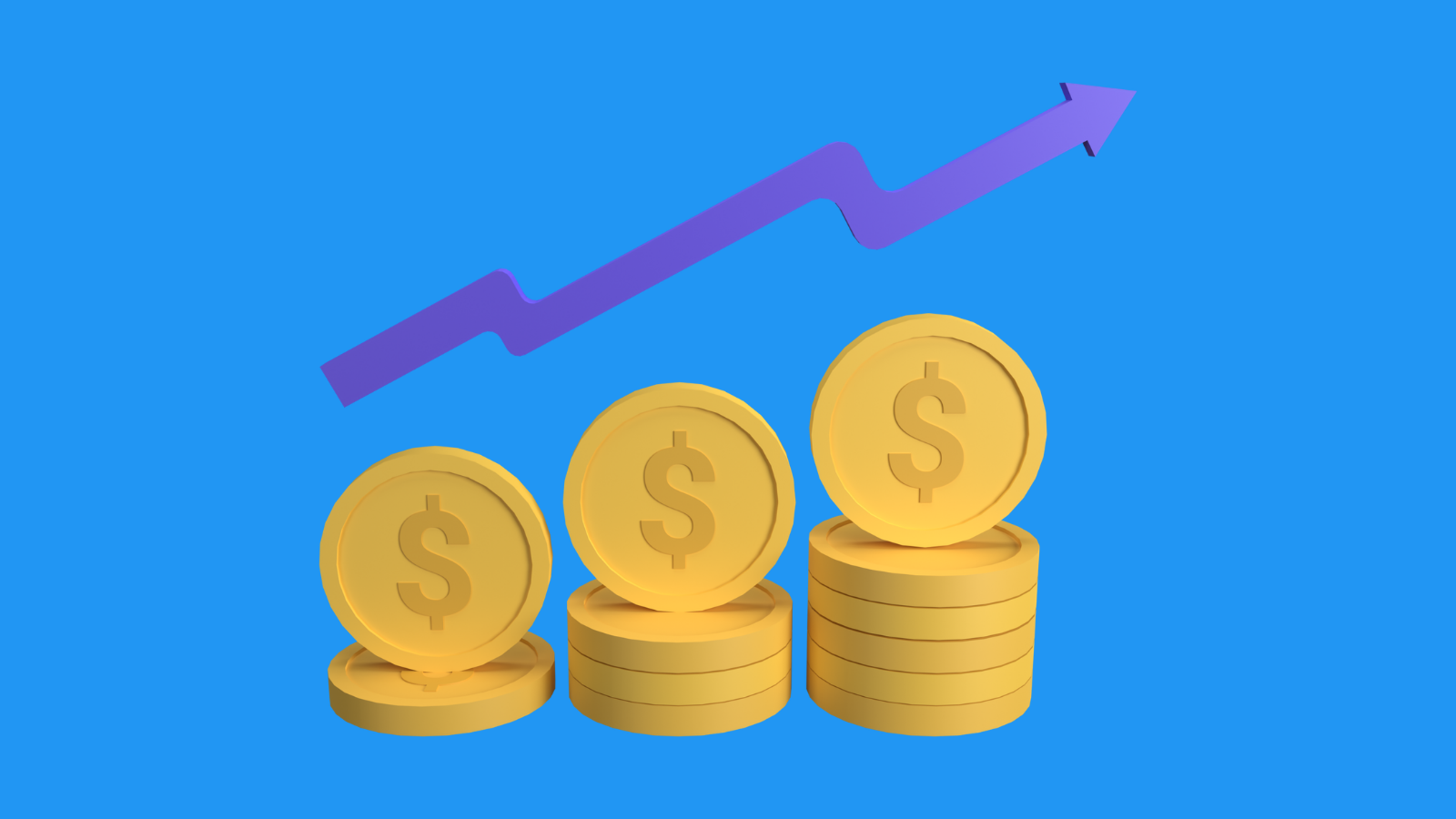
SDGR Stock Price Forecasting Model
This model utilizes a hybrid approach combining historical financial data, macroeconomic indicators, and sentiment analysis to predict the future performance of Schrodinger Inc. (SDGR) common stock. The core of the model rests on a recurrent neural network (RNN) architecture, specifically a Long Short-Term Memory (LSTM) network. LSTMs excel at capturing temporal dependencies in financial time series, a crucial aspect for accurate stock prediction. The model is trained on a comprehensive dataset including past stock prices, earnings reports, news articles, and relevant economic data (GDP growth, inflation rates, interest rates). This data is preprocessed and transformed to ensure data quality and prevent overfitting. Feature engineering plays a critical role in improving model performance. Key features extracted include technical indicators such as moving averages, Bollinger Bands, and relative strength index, alongside fundamental indicators derived from financial statements. A crucial element is the integration of sentiment analysis, utilizing natural language processing techniques to gauge market sentiment concerning Schrodinger Inc., which can significantly influence stock price fluctuations. This data is embedded into the model, allowing it to consider public perception and market mood as factors.
The model is rigorously evaluated using techniques such as backtesting and cross-validation to assess its predictive accuracy. Performance metrics like mean absolute error (MAE), root mean squared error (RMSE), and R-squared are employed to quantify the model's ability to forecast future stock values. Further refinements are implemented to mitigate potential bias and enhance the robustness of the model, including hyperparameter tuning and ensemble methods. These methods involve combining the predictions of multiple models to create a more stable and reliable forecast. In addition, the model is continuously updated with new data to reflect changes in market dynamics and company performance. This dynamic adaptation is vital for maintaining the model's predictive accuracy and relevance over time. The model's outputs are probabilistic, providing confidence intervals around the predicted price points, which are essential for investors to make informed decisions.
The output of the model is a probabilistic forecast of future SDGR stock prices. This forecast is presented as a range of possible outcomes, along with associated probabilities. These probabilities offer insights into the likelihood of different price trajectories, helping investors to assess potential risks and rewards. The model incorporates risk management considerations by identifying potential market shocks and providing early warnings of unusual price movements. This proactive approach assists in mitigating investment risks and maximizing returns. The model is designed to be transparent and explainable, allowing users to understand the rationale behind the predicted outcomes. This enhanced transparency supports the process of informed decision making, reducing guesswork and promoting confidence in the model's output.
ML Model Testing
n:Time series to forecast
p:Price signals of Schrodinger stock
j:Nash equilibria (Neural Network)
k:Dominated move of Schrodinger stock holders
a:Best response for Schrodinger target price
For further technical information as per how our model work we invite you to visit the article below:
How do KappaSignal algorithms actually work?
Schrodinger Stock Forecast (Buy or Sell) Strategic Interaction Table
Strategic Interaction Table Legend:
X axis: *Likelihood% (The higher the percentage value, the more likely the event will occur.)
Y axis: *Potential Impact% (The higher the percentage value, the more likely the price will deviate.)
Z axis (Grey to Black): *Technical Analysis%
Schrodinger Inc. Common Stock Financial Outlook and Forecast
Schrodinger's financial outlook hinges significantly on its ability to transition from a research-oriented company to a commercially viable entity in the drug discovery and materials science sectors. The company's primary focus is on accelerating drug discovery and development using its proprietary computational platforms. While these platforms hold substantial promise, successful commercialization remains a key driver of future profitability. Key performance indicators, such as revenue generation, cost management, and the establishment of strategic partnerships, will be critical in determining the company's future trajectory. Significant investment in research and development, while essential for innovation, has historically placed pressure on near-term profitability. The company's strategy for generating revenue through licensing agreements, collaborations, and potential software sales will define its financial success in the coming years. Understanding the evolving market dynamics surrounding computational drug discovery is crucial in assessing Schrodinger's potential to capture market share.
A crucial aspect of evaluating Schrodinger's future involves analyzing market trends in computational drug discovery. The increasing demand for innovative approaches to drug development creates a potential opportunity for Schrodinger. The growing adoption of AI and machine learning in scientific research also presents opportunities for the company. Successful partnerships and collaborations will be instrumental in expanding market access and accelerating revenue generation. Demonstrating the practical application of its computational platforms, particularly in successfully completing and demonstrating preclinical studies, will be pivotal in generating interest among pharmaceutical companies and investors. The market for computational tools in materials science is also potentially substantial, although less mature than the pharmaceutical industry. Securing and maintaining intellectual property rights related to its core technology is essential for maintaining a competitive edge.
A significant factor in Schrodinger's future financial performance is the execution of its business strategy. Successfully monetizing its technology through licensing agreements, collaborations, and sales of software tools is crucial for positive outcomes. Careful cost management and efficient resource allocation will be essential to maintaining profitability, particularly in the face of ongoing research and development efforts. The ability to attract and retain top talent in the computational sciences will be vital to the continued development and refinement of Schrodinger's platform. Maintaining a strong presence and brand recognition in the scientific community are key to driving demand for the platform's services. The company's leadership team's expertise and experience will be a critical factor in navigating the complexities of the market and executing a successful strategy.
Prediction: A cautiously optimistic outlook for Schrodinger's future is warranted. The potential for substantial returns, given the transformative power of its technology, exists. However, the path to achieving significant profits is not guaranteed. Risks associated with this prediction include: the inability to secure significant licensing or sales agreements, substantial unforeseen technological limitations of the platform, or intense competition in the market. Significant market volatility in the pharmaceutical industry could also impact Schrodinger's prospects. The success will depend largely on the speed and effectiveness of translating research findings into commercial applications. Investors should carefully monitor the company's execution of its business strategy and the progress of ongoing collaborations. Further evaluation of the market for computational tools in materials science and the company's market positioning within that space is necessary.
Rating | Short-Term | Long-Term Senior |
---|---|---|
Outlook | B2 | Ba1 |
Income Statement | C | Ba2 |
Balance Sheet | Caa2 | B2 |
Leverage Ratios | B1 | Ba3 |
Cash Flow | Baa2 | Baa2 |
Rates of Return and Profitability | Baa2 | Baa2 |
*Financial analysis is the process of evaluating a company's financial performance and position by neural network. It involves reviewing the company's financial statements, including the balance sheet, income statement, and cash flow statement, as well as other financial reports and documents.
How does neural network examine financial reports and understand financial state of the company?
References
- Athey S, Imbens G, Wager S. 2016a. Efficient inference of average treatment effects in high dimensions via approximate residual balancing. arXiv:1604.07125 [math.ST]
- Harris ZS. 1954. Distributional structure. Word 10:146–62
- A. Tamar and S. Mannor. Variance adjusted actor critic algorithms. arXiv preprint arXiv:1310.3697, 2013.
- Morris CN. 1983. Parametric empirical Bayes inference: theory and applications. J. Am. Stat. Assoc. 78:47–55
- M. Sobel. The variance of discounted Markov decision processes. Applied Probability, pages 794–802, 1982
- Keane MP. 2013. Panel data discrete choice models of consumer demand. In The Oxford Handbook of Panel Data, ed. BH Baltagi, pp. 54–102. Oxford, UK: Oxford Univ. Press
- Bastani H, Bayati M. 2015. Online decision-making with high-dimensional covariates. Work. Pap., Univ. Penn./ Stanford Grad. School Bus., Philadelphia/Stanford, CA