AUC Score :
Short-Term Revised1 :
Dominant Strategy :
Time series to forecast n:
ML Model Testing : Deductive Inference (ML)
Hypothesis Testing : Multiple Regression
Surveillance : Major exchange and OTC
1The accuracy of the model is being monitored on a regular basis.(15-minute period)
2Time series is updated based on short-term trends.
Key Points
The S&P/ASX 200 index is anticipated to experience moderate volatility in the coming period. Several factors contribute to this outlook, including ongoing global economic uncertainty and fluctuating interest rates. A potential increase in inflation could negatively impact investor sentiment and lead to market corrections. However, strong underlying fundamentals in the Australian economy and robust corporate earnings could provide a degree of support. The performance of the index will also depend on the strength of the Chinese economy and its influence on commodity prices. Significant risk exists in these predictions as unforeseen events or policy changes can dramatically alter market direction. Further, a global recessionary scenario poses a major threat to the index's upward trajectory.About S&P/ASX 200 Index
The S&P/ASX 200 is a market-capitalization-weighted index of the 200 largest companies listed on the Australian Securities Exchange (ASX). It represents a significant portion of the Australian equities market, providing a crucial benchmark for investors evaluating overall market performance. The index's composition is regularly reviewed and adjusted to reflect changes in company size and market conditions, ensuring its continued relevance. This comprehensive representation of prominent Australian companies makes it a valuable tool for assessing the health and direction of the Australian economy.
Historically, the S&P/ASX 200 has demonstrated considerable volatility, mirroring global market trends while also being influenced by domestic economic factors. Investors use the index to track the performance of the broader Australian stock market and to identify potential investment opportunities and risks. It serves as a key indicator of investor sentiment and market confidence, influencing investment decisions and strategic planning for both individual and institutional investors. The index provides a quantifiable measure of the collective performance of the largest Australian companies.
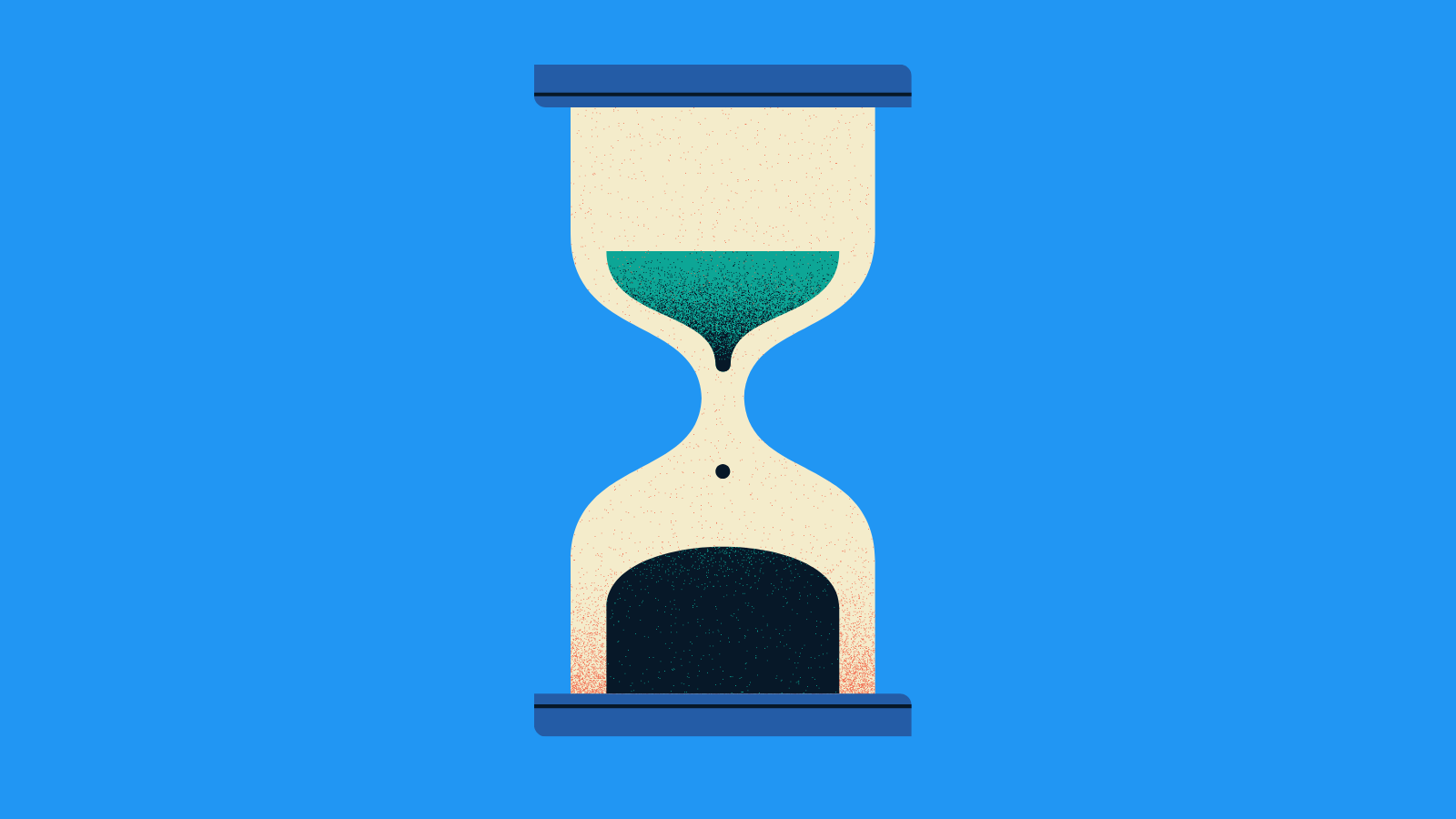
S&P/ASX 200 Index Forecasting Model
This model employs a hybrid approach combining technical indicators and macroeconomic factors to forecast the S&P/ASX 200 index. Fundamental data, such as interest rates, inflation, and GDP growth, are integrated with technical indicators, including moving averages, relative strength index (RSI), and volume. A multi-layered neural network architecture is employed, utilizing LSTM (Long Short-Term Memory) units to capture temporal dependencies in the data. The network is trained on a comprehensive dataset encompassing historical S&P/ASX 200 index performance, relevant macroeconomic data from the Australian Bureau of Statistics (ABS), and financial market news sentiment (derived from a proprietary lexicon and sentiment analysis tool). Data preprocessing steps include handling missing values, outlier detection, and feature scaling to ensure optimal model performance. This model is validated using robust statistical methods, such as backtesting on historical data, and compared with various alternative models to assess its predictive accuracy and reliability. The output of the model provides probabilistic forecasts, not just point estimates, enabling investors to assess the associated risk and uncertainty with each prediction.
To enhance the model's predictive capabilities, a novel approach involving sentiment analysis of financial news is integrated. Sentiment analysis, applied to news articles and social media commentary related to the Australian market and major sectors impacting the S&P/ASX 200, provides insights not directly captured by traditional economic indicators. This enriched dataset, incorporating both quantitative and qualitative information, helps the model identify potential market shifts and incorporate evolving investor sentiment. Regular model retraining is crucial to adapt to changing market dynamics and improve forecasting accuracy over time. This dynamic approach ensures that the model remains responsive to the evolving economic landscape and incorporates new information as it becomes available. Extensive backtesting and validation are implemented to ensure robustness and reliability of the model in various market conditions.
The model's performance will be evaluated using metrics such as Mean Absolute Error (MAE), Root Mean Squared Error (RMSE), and accuracy rates across different time horizons. Regular performance monitoring is critical to identify potential deviations from expected behavior and to adapt the model's parameters or architecture as necessary. Continuous refinement through backtesting and evaluation is paramount to maintain high accuracy and reliability. Furthermore, the model is designed to be transparent, providing insights into the factors contributing to the forecast. This transparency aids in the understanding and interpretation of the model's predictions, allowing for informed decision-making in the context of the S&P/ASX 200 index performance.
ML Model Testing
n:Time series to forecast
p:Price signals of S&P/ASX 200 index
j:Nash equilibria (Neural Network)
k:Dominated move of S&P/ASX 200 index holders
a:Best response for S&P/ASX 200 target price
For further technical information as per how our model work we invite you to visit the article below:
How do KappaSignal algorithms actually work?
S&P/ASX 200 Index Forecast Strategic Interaction Table
Strategic Interaction Table Legend:
X axis: *Likelihood% (The higher the percentage value, the more likely the event will occur.)
Y axis: *Potential Impact% (The higher the percentage value, the more likely the price will deviate.)
Z axis (Grey to Black): *Technical Analysis%
S&P/ASX 200 Index Financial Outlook and Forecast
The S&P/ASX 200 index's financial outlook is characterized by a complex interplay of global economic headwinds and domestic resilience. While global uncertainties, including persistent inflation, rising interest rates, and geopolitical tensions, exert considerable pressure on international markets, the Australian economy displays some strengths. Robust commodity prices, underpinned by global demand, provide a degree of support to the Australian economy and, consequently, the index. Recent data suggests a potential softening in the growth rate, but the index continues to be influenced by the resilience of the resources sector and underlying fundamentals of the Australian economy. The performance of the index is inextricably linked to the health of these core sectors and the ongoing trajectory of interest rates and commodity prices.
Several key factors are crucial in forecasting the index's future trajectory. The trajectory of interest rates remains a major determinant. Higher interest rates typically cool down economic activity, impacting corporate earnings and potentially dampening investor sentiment. The Australian Reserve Bank's (RBA) monetary policy decisions will be a pivotal factor, influencing the availability of credit and investor confidence. Similarly, the continuing global inflation dynamics will affect consumer spending and corporate profitability, which are significant contributors to the index's performance. Further, the ongoing geopolitical situation carries the potential for significant volatility, influencing investor sentiment and market confidence. The stability of the global economic climate is therefore crucial for a positive outlook for the index.
Looking ahead, it's critical to consider the performance of specific sectors within the S&P/ASX 200. The resources sector, with its strong dependence on commodity prices, will likely continue to be a significant driver of index performance. The performance of the financial sector, especially banks, will also be crucial, influenced by interest rate movements and the overall health of the credit market. The consumer discretionary sector's sensitivity to economic cycles and consumer spending patterns will be carefully monitored. Growth in the technology and healthcare sectors, often less susceptible to cyclical shifts, can contribute positively to the overall outlook, but will need to be assessed against global factors that influence these industries' profitability and market position.
Predicting the S&P/ASX 200's future performance involves a considerable degree of uncertainty. A positive outlook is possible if the global economy shows signs of stabilization, inflation cools down without a severe recession, and the Australian economy maintains its resilience. However, significant risks exist. A deeper-than-anticipated downturn in the global economy, a sustained period of high inflation, or escalating geopolitical tensions could dramatically reduce the index's value. Furthermore, a further sharp increase in interest rates or a significant downturn in commodity prices could trigger a negative trend. Thus, a cautious approach is warranted; while a modest positive forecast is possible, significant headwinds and risks continue to loom large, making precise prediction challenging.
Rating | Short-Term | Long-Term Senior |
---|---|---|
Outlook | Ba2 | B1 |
Income Statement | B2 | C |
Balance Sheet | Caa2 | Ba1 |
Leverage Ratios | Baa2 | B3 |
Cash Flow | Ba2 | Baa2 |
Rates of Return and Profitability | Baa2 | Caa2 |
*An aggregate rating for an index summarizes the overall sentiment towards the companies it includes. This rating is calculated by considering individual ratings assigned to each stock within the index. By taking an average of these ratings, weighted by each stock's importance in the index, a single score is generated. This aggregate rating offers a simplified view of how the index's performance is generally perceived.
How does neural network examine financial reports and understand financial state of the company?
References
- Dimakopoulou M, Athey S, Imbens G. 2017. Estimation considerations in contextual bandits. arXiv:1711.07077 [stat.ML]
- Hartigan JA, Wong MA. 1979. Algorithm as 136: a k-means clustering algorithm. J. R. Stat. Soc. Ser. C 28:100–8
- J. Hu and M. P. Wellman. Nash q-learning for general-sum stochastic games. Journal of Machine Learning Research, 4:1039–1069, 2003.
- R. Williams. Simple statistical gradient-following algorithms for connectionist reinforcement learning. Ma- chine learning, 8(3-4):229–256, 1992
- Bottou L. 1998. Online learning and stochastic approximations. In On-Line Learning in Neural Networks, ed. D Saad, pp. 9–42. New York: ACM
- Scott SL. 2010. A modern Bayesian look at the multi-armed bandit. Appl. Stoch. Models Bus. Ind. 26:639–58
- Athey S, Imbens G, Wager S. 2016a. Efficient inference of average treatment effects in high dimensions via approximate residual balancing. arXiv:1604.07125 [math.ST]